کد مقاله | کد نشریه | سال انتشار | مقاله انگلیسی | نسخه تمام متن |
---|---|---|---|---|
263227 | 504068 | 2013 | 10 صفحه PDF | دانلود رایگان |
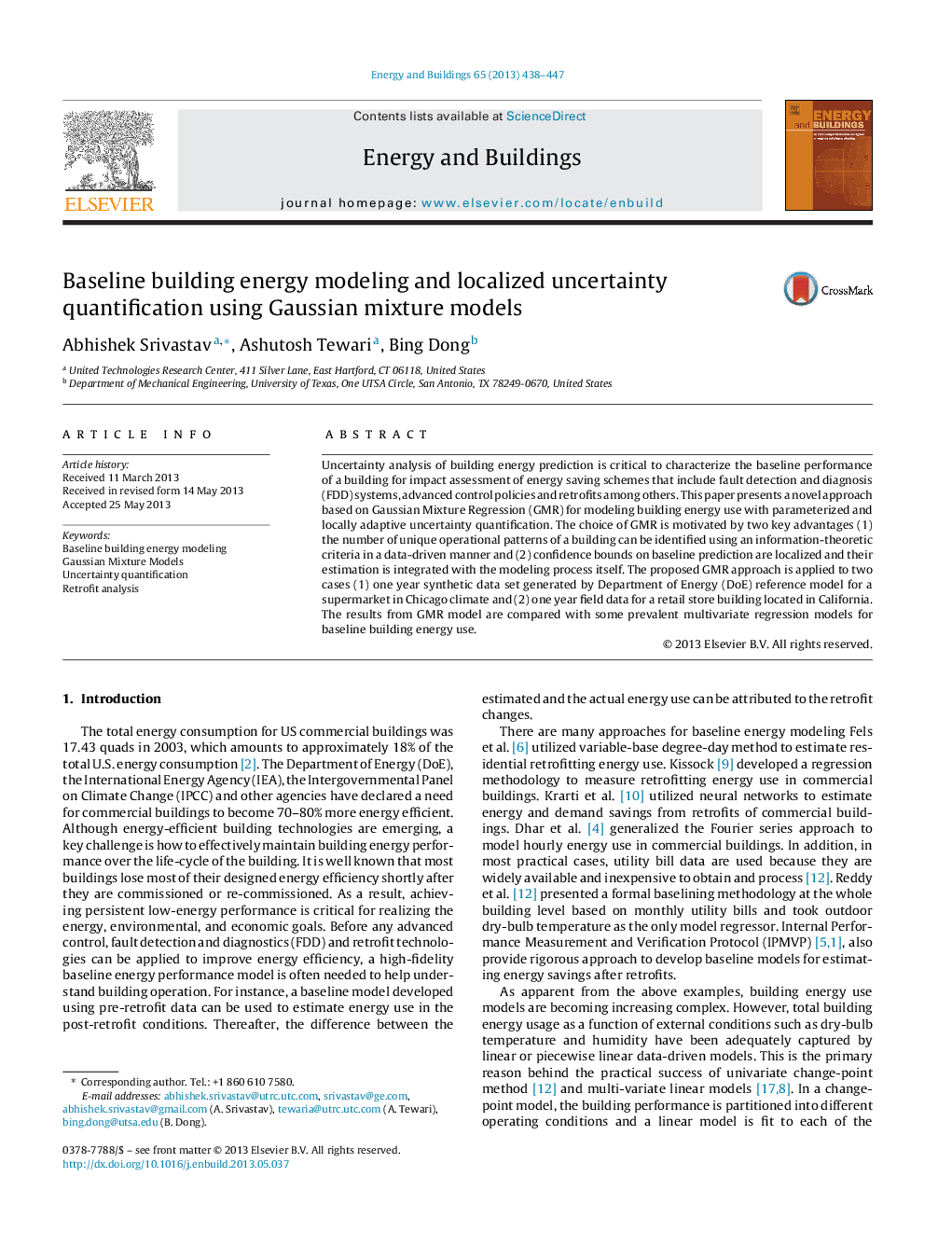
• Gaussian Mixture Regression approach for building energy modeling.
• Parameterized and locally adaptive uncertainty quantification.
• Applied to both synthetic and real one year data.
• The results from GMR compared with multivariate regression models.
Uncertainty analysis of building energy prediction is critical to characterize the baseline performance of a building for impact assessment of energy saving schemes that include fault detection and diagnosis (FDD) systems, advanced control policies and retrofits among others. This paper presents a novel approach based on Gaussian Mixture Regression (GMR) for modeling building energy use with parameterized and locally adaptive uncertainty quantification. The choice of GMR is motivated by two key advantages (1) the number of unique operational patterns of a building can be identified using an information-theoretic criteria in a data-driven manner and (2) confidence bounds on baseline prediction are localized and their estimation is integrated with the modeling process itself. The proposed GMR approach is applied to two cases (1) one year synthetic data set generated by Department of Energy (DoE) reference model for a supermarket in Chicago climate and (2) one year field data for a retail store building located in California. The results from GMR model are compared with some prevalent multivariate regression models for baseline building energy use.
Journal: Energy and Buildings - Volume 65, October 2013, Pages 438–447