کد مقاله | کد نشریه | سال انتشار | مقاله انگلیسی | نسخه تمام متن |
---|---|---|---|---|
2811887 | 1569295 | 2007 | 17 صفحه PDF | دانلود رایگان |
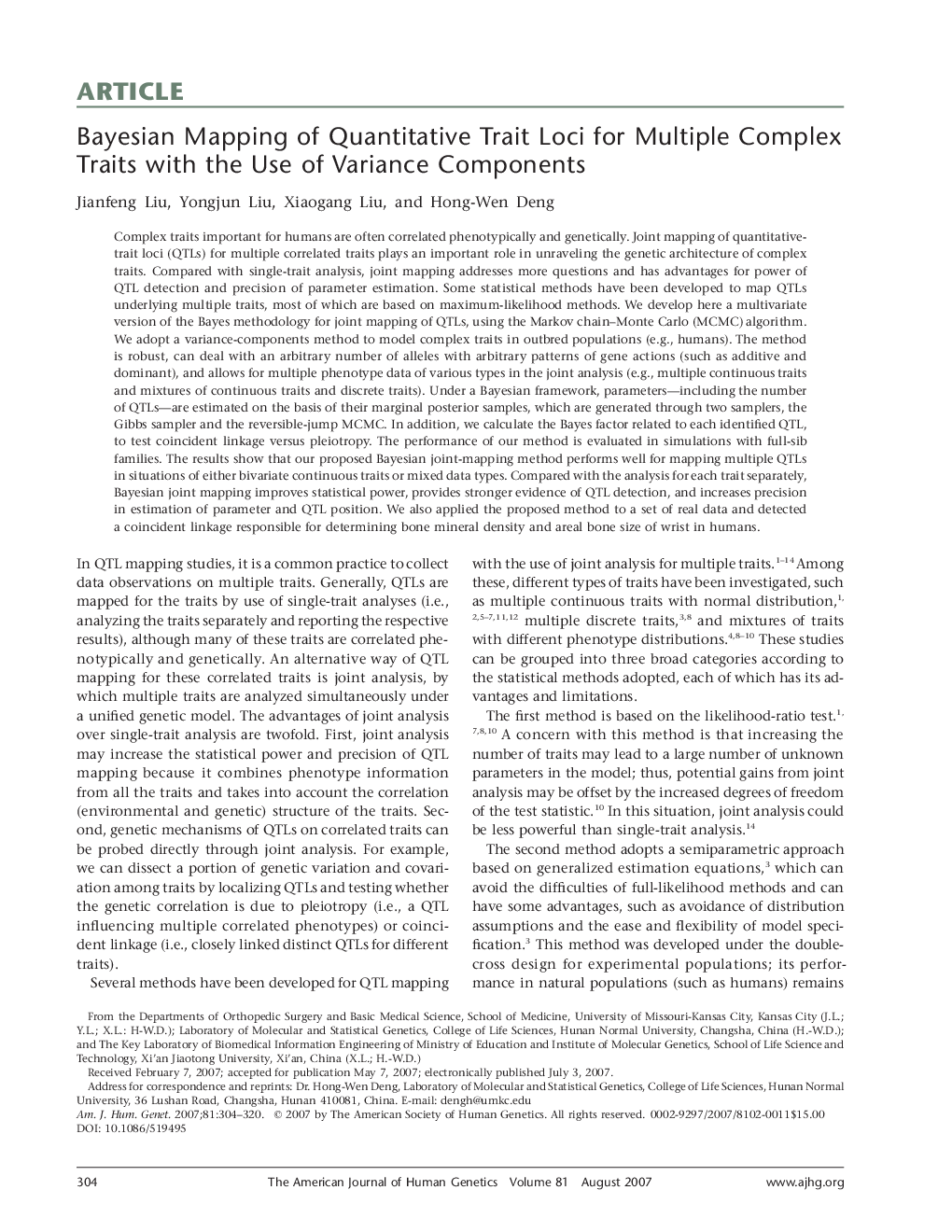
Complex traits important for humans are often correlated phenotypically and genetically. Joint mapping of quantitative-trait loci (QTLs) for multiple correlated traits plays an important role in unraveling the genetic architecture of complex traits. Compared with single-trait analysis, joint mapping addresses more questions and has advantages for power of QTL detection and precision of parameter estimation. Some statistical methods have been developed to map QTLs underlying multiple traits, most of which are based on maximum-likelihood methods. We develop here a multivariate version of the Bayes methodology for joint mapping of QTLs, using the Markov chain–Monte Carlo (MCMC) algorithm. We adopt a variance-components method to model complex traits in outbred populations (e.g., humans). The method is robust, can deal with an arbitrary number of alleles with arbitrary patterns of gene actions (such as additive and dominant), and allows for multiple phenotype data of various types in the joint analysis (e.g., multiple continuous traits and mixtures of continuous traits and discrete traits). Under a Bayesian framework, parameters—including the number of QTLs—are estimated on the basis of their marginal posterior samples, which are generated through two samplers, the Gibbs sampler and the reversible-jump MCMC. In addition, we calculate the Bayes factor related to each identified QTL, to test coincident linkage versus pleiotropy. The performance of our method is evaluated in simulations with full-sib families. The results show that our proposed Bayesian joint-mapping method performs well for mapping multiple QTLs in situations of either bivariate continuous traits or mixed data types. Compared with the analysis for each trait separately, Bayesian joint mapping improves statistical power, provides stronger evidence of QTL detection, and increases precision in estimation of parameter and QTL position. We also applied the proposed method to a set of real data and detected a coincident linkage responsible for determining bone mineral density and areal bone size of wrist in humans.
Journal: - Volume 81, Issue 2, August 2007, Pages 304–320