کد مقاله | کد نشریه | سال انتشار | مقاله انگلیسی | نسخه تمام متن |
---|---|---|---|---|
3075084 | 1580960 | 2015 | 9 صفحه PDF | دانلود رایگان |
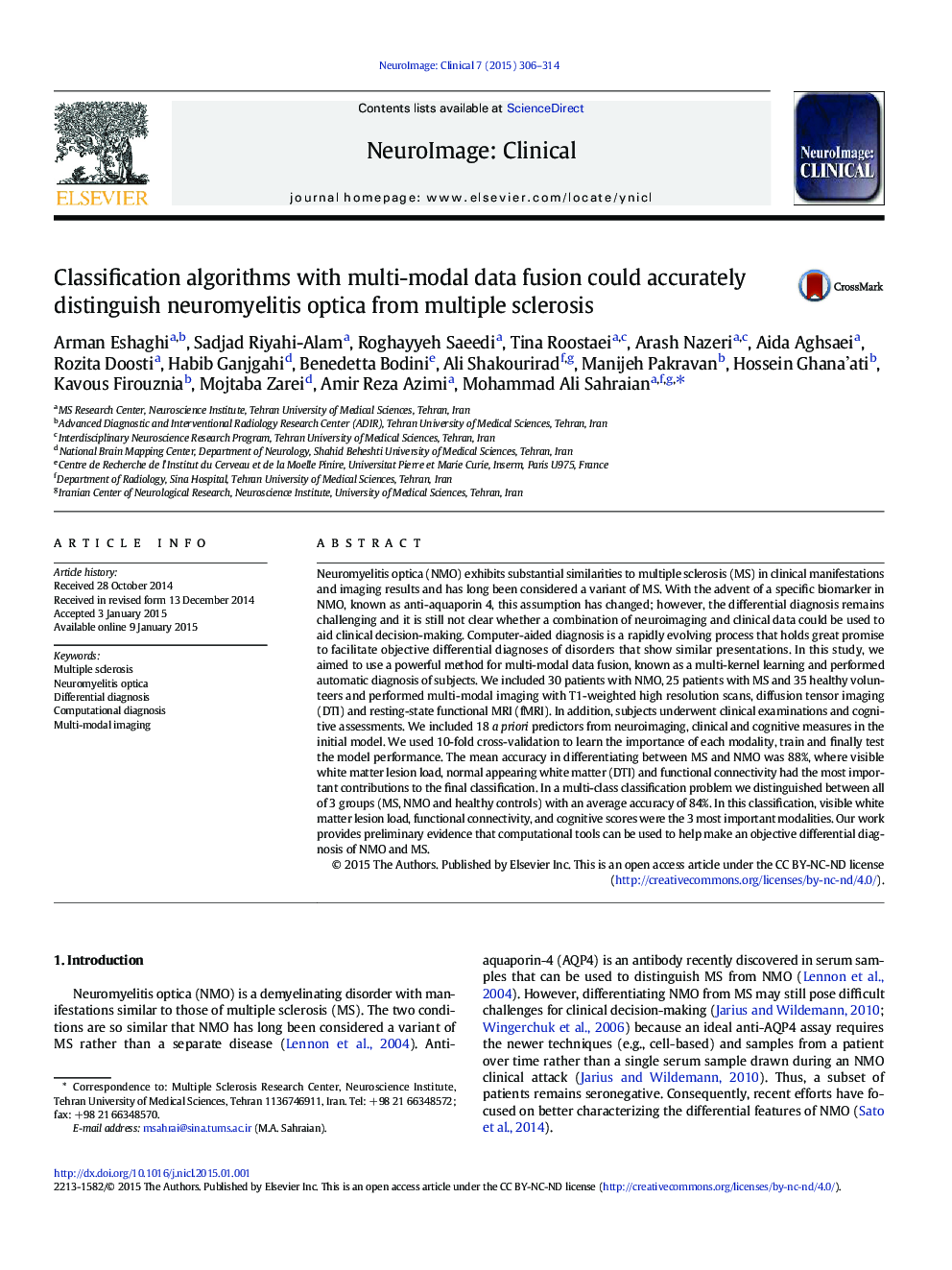
• We developed models for automatic differential diagnosis between multiple sclerosis and neuromyelitis optica.
• Multimodal imaging may be integrated with clinical and cognitive data to develop multidimensional classification algorithms.
• Classification algorithms could be used to aid in objective clinical decision making.
• Future research should assess the generalizability of classification algorithms in independent cohorts.
Neuromyelitis optica (NMO) exhibits substantial similarities to multiple sclerosis (MS) in clinical manifestations and imaging results and has long been considered a variant of MS. With the advent of a specific biomarker in NMO, known as anti-aquaporin 4, this assumption has changed; however, the differential diagnosis remains challenging and it is still not clear whether a combination of neuroimaging and clinical data could be used to aid clinical decision-making. Computer-aided diagnosis is a rapidly evolving process that holds great promise to facilitate objective differential diagnoses of disorders that show similar presentations. In this study, we aimed to use a powerful method for multi-modal data fusion, known as a multi-kernel learning and performed automatic diagnosis of subjects. We included 30 patients with NMO, 25 patients with MS and 35 healthy volunteers and performed multi-modal imaging with T1-weighted high resolution scans, diffusion tensor imaging (DTI) and resting-state functional MRI (fMRI). In addition, subjects underwent clinical examinations and cognitive assessments. We included 18 a priori predictors from neuroimaging, clinical and cognitive measures in the initial model. We used 10-fold cross-validation to learn the importance of each modality, train and finally test the model performance. The mean accuracy in differentiating between MS and NMO was 88%, where visible white matter lesion load, normal appearing white matter (DTI) and functional connectivity had the most important contributions to the final classification. In a multi-class classification problem we distinguished between all of 3 groups (MS, NMO and healthy controls) with an average accuracy of 84%. In this classification, visible white matter lesion load, functional connectivity, and cognitive scores were the 3 most important modalities. Our work provides preliminary evidence that computational tools can be used to help make an objective differential diagnosis of NMO and MS.
Journal: NeuroImage: Clinical - Volume 7, 2015, Pages 306–314