کد مقاله | کد نشریه | سال انتشار | مقاله انگلیسی | نسخه تمام متن |
---|---|---|---|---|
377841 | 658836 | 2010 | 10 صفحه PDF | دانلود رایگان |
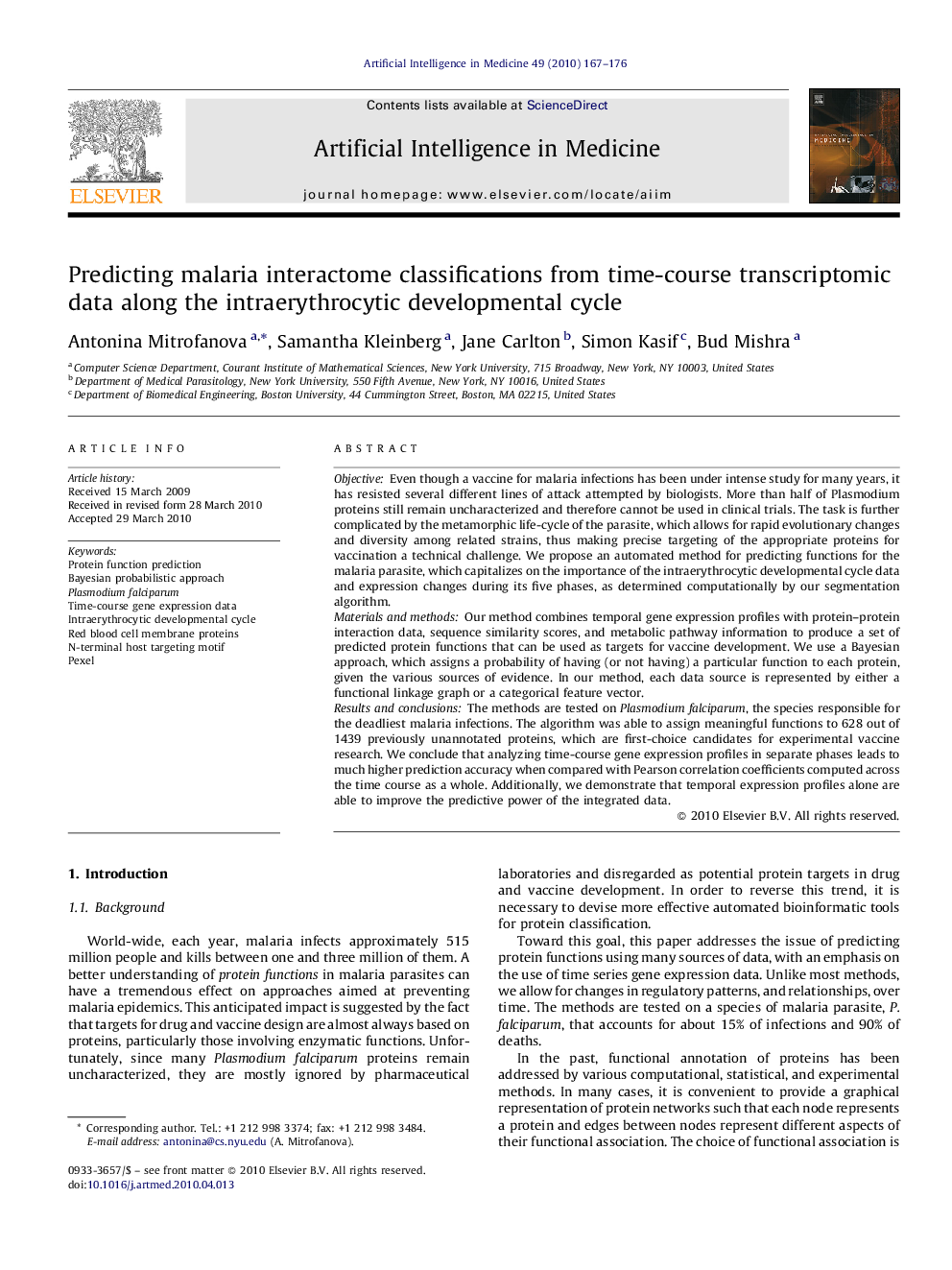
ObjectiveEven though a vaccine for malaria infections has been under intense study for many years, it has resisted several different lines of attack attempted by biologists. More than half of Plasmodium proteins still remain uncharacterized and therefore cannot be used in clinical trials. The task is further complicated by the metamorphic life-cycle of the parasite, which allows for rapid evolutionary changes and diversity among related strains, thus making precise targeting of the appropriate proteins for vaccination a technical challenge. We propose an automated method for predicting functions for the malaria parasite, which capitalizes on the importance of the intraerythrocytic developmental cycle data and expression changes during its five phases, as determined computationally by our segmentation algorithm.Materials and methodsOur method combines temporal gene expression profiles with protein–protein interaction data, sequence similarity scores, and metabolic pathway information to produce a set of predicted protein functions that can be used as targets for vaccine development. We use a Bayesian approach, which assigns a probability of having (or not having) a particular function to each protein, given the various sources of evidence. In our method, each data source is represented by either a functional linkage graph or a categorical feature vector.Results and conclusionsThe methods are tested on Plasmodium falciparum, the species responsible for the deadliest malaria infections. The algorithm was able to assign meaningful functions to 628 out of 1439 previously unannotated proteins, which are first-choice candidates for experimental vaccine research. We conclude that analyzing time-course gene expression profiles in separate phases leads to much higher prediction accuracy when compared with Pearson correlation coefficients computed across the time course as a whole. Additionally, we demonstrate that temporal expression profiles alone are able to improve the predictive power of the integrated data.
Journal: Artificial Intelligence in Medicine - Volume 49, Issue 3, July 2010, Pages 167–176