کد مقاله | کد نشریه | سال انتشار | مقاله انگلیسی | نسخه تمام متن |
---|---|---|---|---|
378321 | 659017 | 2012 | 17 صفحه PDF | دانلود رایگان |
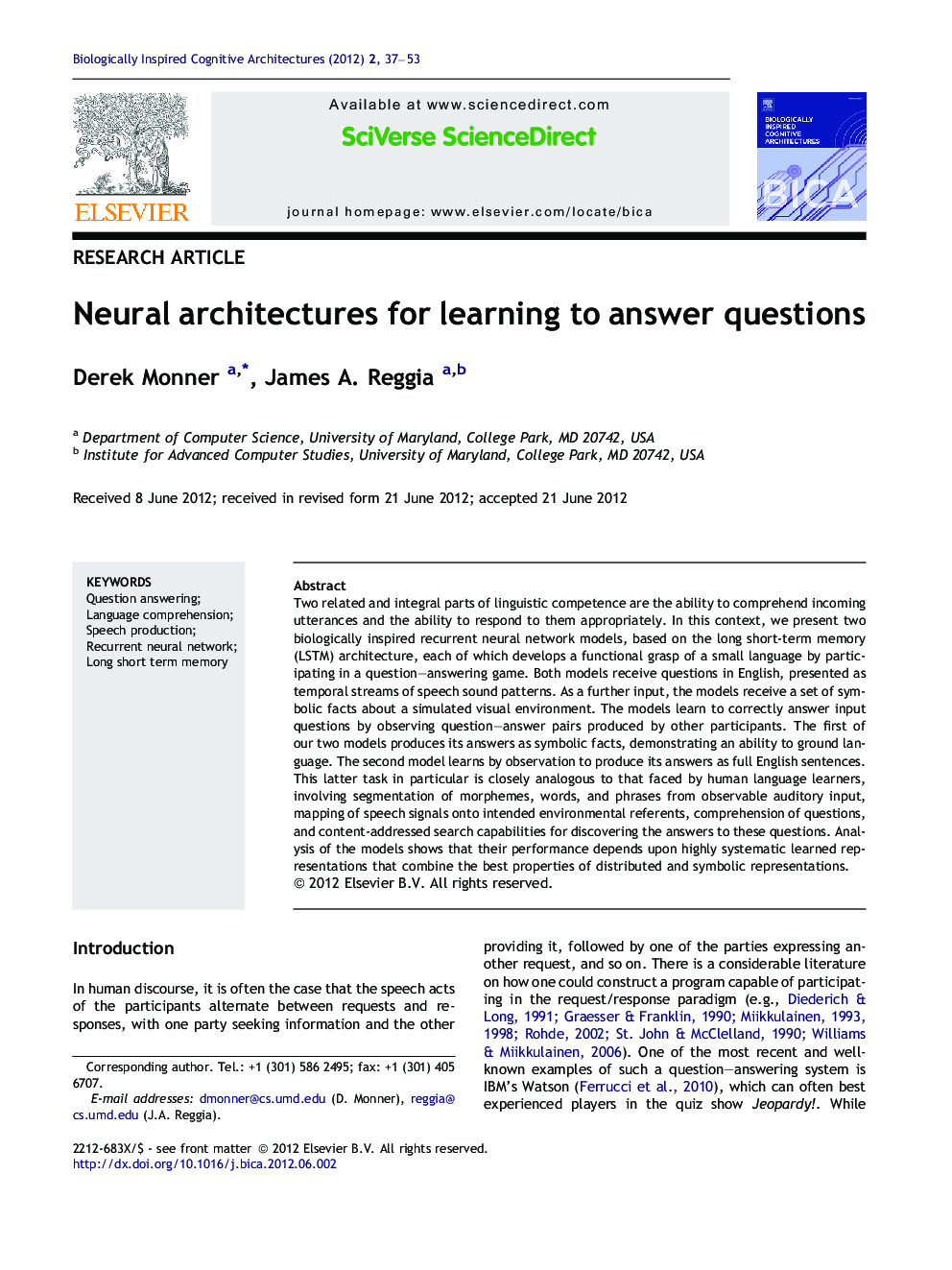
Two related and integral parts of linguistic competence are the ability to comprehend incoming utterances and the ability to respond to them appropriately. In this context, we present two biologically inspired recurrent neural network models, based on the long short-term memory (LSTM) architecture, each of which develops a functional grasp of a small language by participating in a question–answering game. Both models receive questions in English, presented as temporal streams of speech sound patterns. As a further input, the models receive a set of symbolic facts about a simulated visual environment. The models learn to correctly answer input questions by observing question–answer pairs produced by other participants. The first of our two models produces its answers as symbolic facts, demonstrating an ability to ground language. The second model learns by observation to produce its answers as full English sentences. This latter task in particular is closely analogous to that faced by human language learners, involving segmentation of morphemes, words, and phrases from observable auditory input, mapping of speech signals onto intended environmental referents, comprehension of questions, and content-addressed search capabilities for discovering the answers to these questions. Analysis of the models shows that their performance depends upon highly systematic learned representations that combine the best properties of distributed and symbolic representations.
Journal: Biologically Inspired Cognitive Architectures - Volume 2, October 2012, Pages 37–53