کد مقاله | کد نشریه | سال انتشار | مقاله انگلیسی | نسخه تمام متن |
---|---|---|---|---|
378745 | 659213 | 2013 | 22 صفحه PDF | دانلود رایگان |
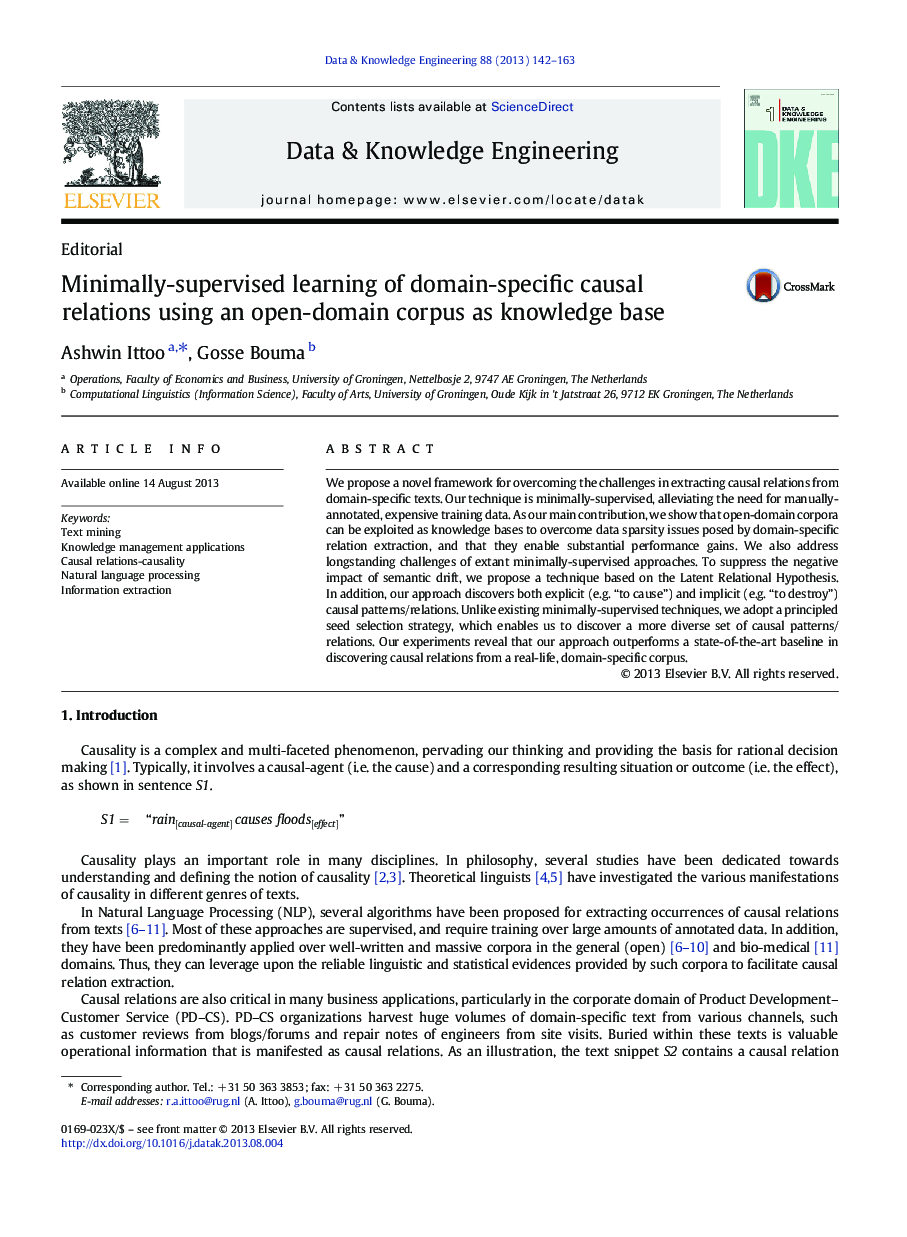
• Novel technique that accurately extracts implicit and explicit causal patterns from sparse, ungrammatical domain-specific texts
• Approach is minimally-supervised, eschewing the need for expensive annotated data.
• Exploits open-domain knowledge base to support domain-specific term extraction
• Addresses the issue of semantic drift using Latent Relational Hypothesis
• The proposed technique outperforms a state-of-the-art baseline over real-life texts.
We propose a novel framework for overcoming the challenges in extracting causal relations from domain-specific texts. Our technique is minimally-supervised, alleviating the need for manually-annotated, expensive training data. As our main contribution, we show that open-domain corpora can be exploited as knowledge bases to overcome data sparsity issues posed by domain-specific relation extraction, and that they enable substantial performance gains. We also address longstanding challenges of extant minimally-supervised approaches. To suppress the negative impact of semantic drift, we propose a technique based on the Latent Relational Hypothesis. In addition, our approach discovers both explicit (e.g. “to cause”) and implicit (e.g. “to destroy”) causal patterns/relations. Unlike existing minimally-supervised techniques, we adopt a principled seed selection strategy, which enables us to discover a more diverse set of causal patterns/relations. Our experiments reveal that our approach outperforms a state-of-the-art baseline in discovering causal relations from a real-life, domain-specific corpus.
Journal: Data & Knowledge Engineering - Volume 88, November 2013, Pages 142–163