کد مقاله | کد نشریه | سال انتشار | مقاله انگلیسی | نسخه تمام متن |
---|---|---|---|---|
380661 | 1437450 | 2014 | 15 صفحه PDF | دانلود رایگان |
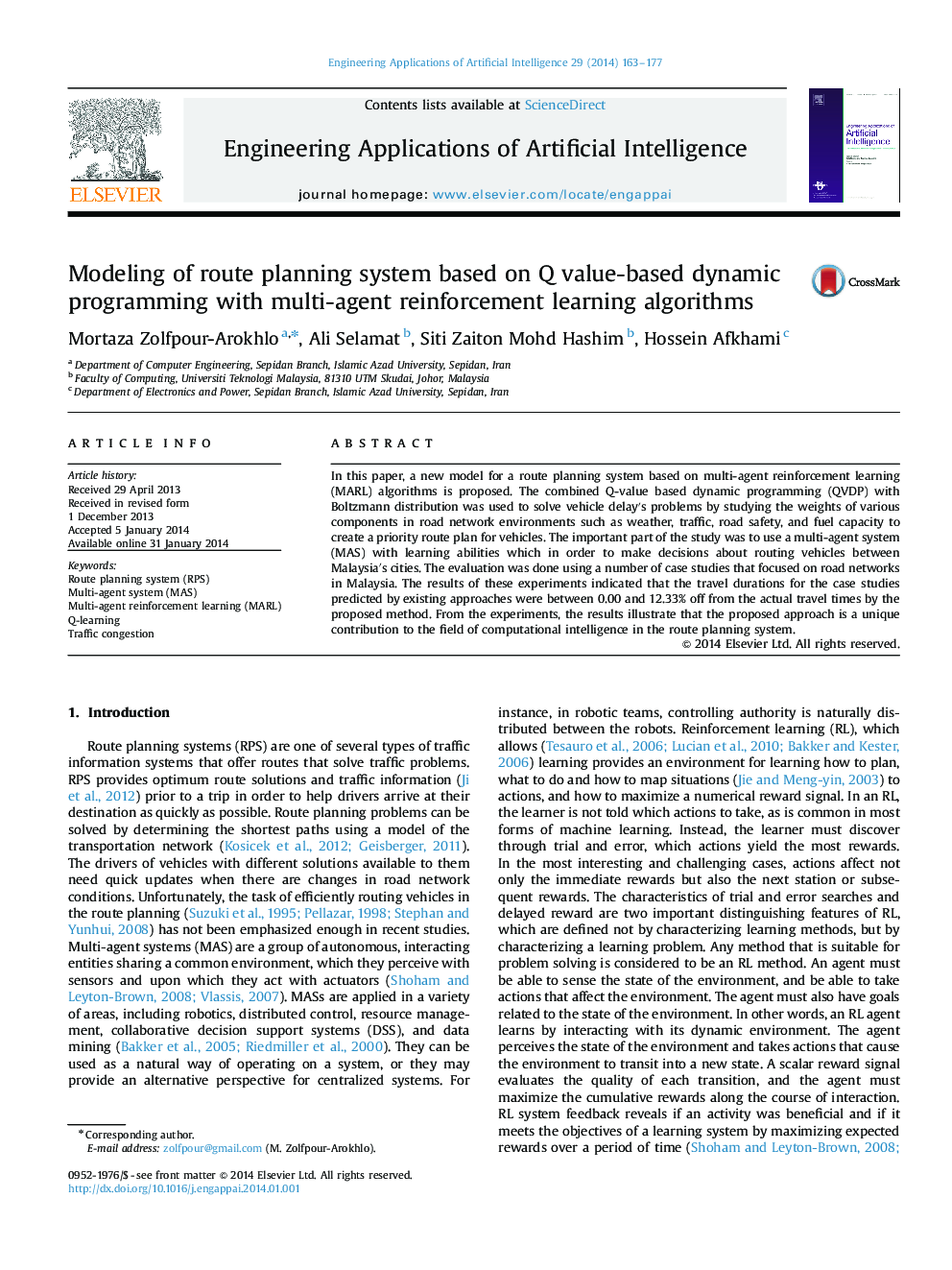
• We report a model in order to reduce vehicle trip times by giving a priority trip plan to vehicles.
• The research uses the ability of learning models to propose several route alternatives to minimize travel costs during driving.
• The multi-agent system also uses its learning abilities to make decisions about routing vehicles between cities.
• Provide high quality travel planning service to the vehicle drivers.
In this paper, a new model for a route planning system based on multi-agent reinforcement learning (MARL) algorithms is proposed. The combined Q-value based dynamic programming (QVDP) with Boltzmann distribution was used to solve vehicle delay's problems by studying the weights of various components in road network environments such as weather, traffic, road safety, and fuel capacity to create a priority route plan for vehicles. The important part of the study was to use a multi-agent system (MAS) with learning abilities which in order to make decisions about routing vehicles between Malaysia's cities. The evaluation was done using a number of case studies that focused on road networks in Malaysia. The results of these experiments indicated that the travel durations for the case studies predicted by existing approaches were between 0.00 and 12.33% off from the actual travel times by the proposed method. From the experiments, the results illustrate that the proposed approach is a unique contribution to the field of computational intelligence in the route planning system.
Comparison of the proposed and the existing method results based on Q value-based dynamic programming with multi-agent reinforcement learning algorithms.Figure optionsDownload as PowerPoint slide
Journal: Engineering Applications of Artificial Intelligence - Volume 29, March 2014, Pages 163–177