کد مقاله | کد نشریه | سال انتشار | مقاله انگلیسی | نسخه تمام متن |
---|---|---|---|---|
380846 | 1437455 | 2013 | 14 صفحه PDF | دانلود رایگان |
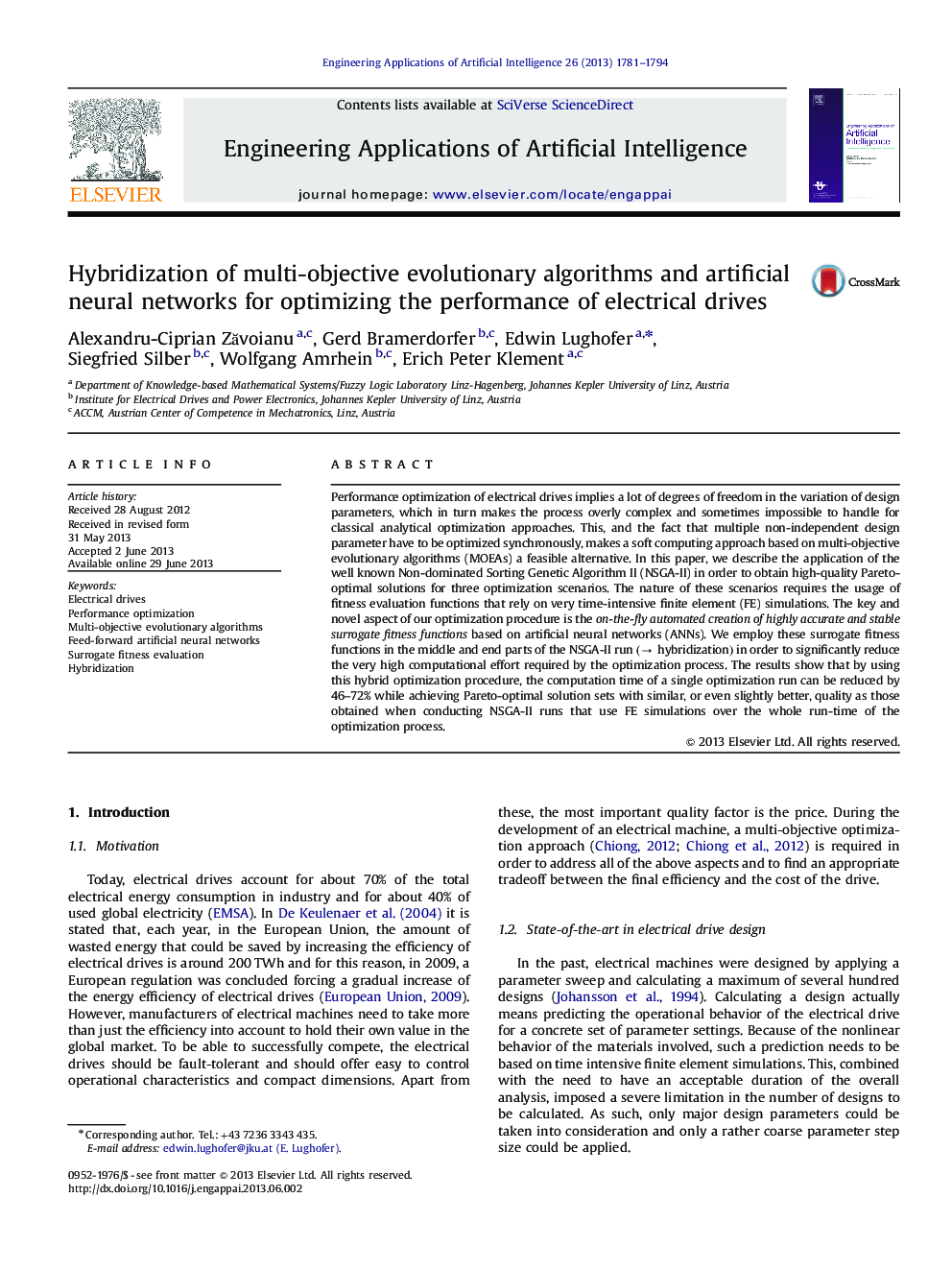
Performance optimization of electrical drives implies a lot of degrees of freedom in the variation of design parameters, which in turn makes the process overly complex and sometimes impossible to handle for classical analytical optimization approaches. This, and the fact that multiple non-independent design parameter have to be optimized synchronously, makes a soft computing approach based on multi-objective evolutionary algorithms (MOEAs) a feasible alternative. In this paper, we describe the application of the well known Non-dominated Sorting Genetic Algorithm II (NSGA-II) in order to obtain high-quality Pareto-optimal solutions for three optimization scenarios. The nature of these scenarios requires the usage of fitness evaluation functions that rely on very time-intensive finite element (FE) simulations. The key and novel aspect of our optimization procedure is the on-the-fly automated creation of highly accurate and stable surrogate fitness functions based on artificial neural networks (ANNs). We employ these surrogate fitness functions in the middle and end parts of the NSGA-II run (→hybridization) in order to significantly reduce the very high computational effort required by the optimization process. The results show that by using this hybrid optimization procedure, the computation time of a single optimization run can be reduced by 46–72% while achieving Pareto-optimal solution sets with similar, or even slightly better, quality as those obtained when conducting NSGA-II runs that use FE simulations over the whole run-time of the optimization process.
Figure optionsDownload as PowerPoint slide
Journal: Engineering Applications of Artificial Intelligence - Volume 26, Issue 8, September 2013, Pages 1781–1794