کد مقاله | کد نشریه | سال انتشار | مقاله انگلیسی | نسخه تمام متن |
---|---|---|---|---|
381066 | 1437461 | 2013 | 14 صفحه PDF | دانلود رایگان |
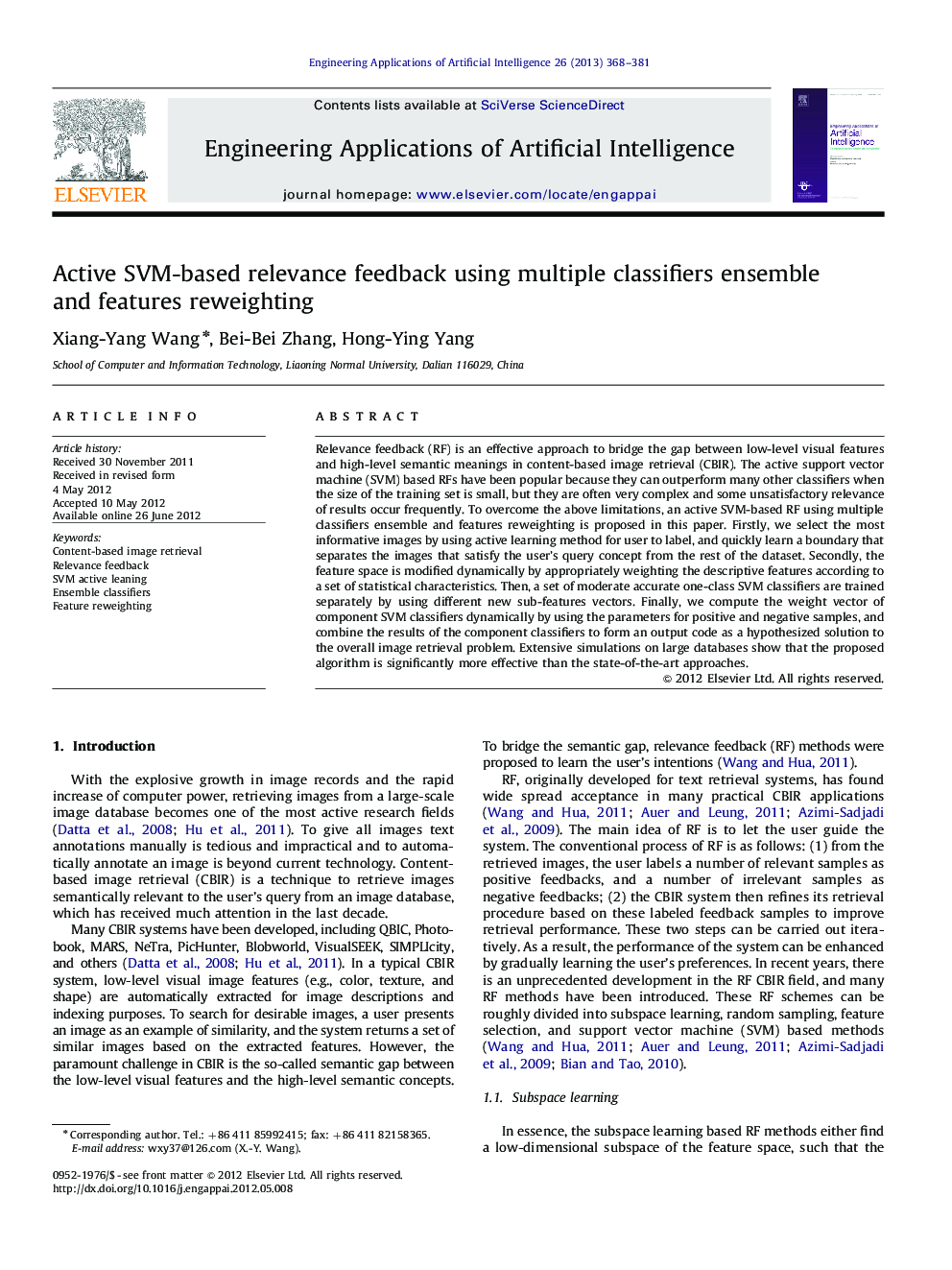
Relevance feedback (RF) is an effective approach to bridge the gap between low-level visual features and high-level semantic meanings in content-based image retrieval (CBIR). The active support vector machine (SVM) based RFs have been popular because they can outperform many other classifiers when the size of the training set is small, but they are often very complex and some unsatisfactory relevance of results occur frequently. To overcome the above limitations, an active SVM-based RF using multiple classifiers ensemble and features reweighting is proposed in this paper. Firstly, we select the most informative images by using active learning method for user to label, and quickly learn a boundary that separates the images that satisfy the user's query concept from the rest of the dataset. Secondly, the feature space is modified dynamically by appropriately weighting the descriptive features according to a set of statistical characteristics. Then, a set of moderate accurate one-class SVM classifiers are trained separately by using different new sub-features vectors. Finally, we compute the weight vector of component SVM classifiers dynamically by using the parameters for positive and negative samples, and combine the results of the component classifiers to form an output code as a hypothesized solution to the overall image retrieval problem. Extensive simulations on large databases show that the proposed algorithm is significantly more effective than the state-of-the-art approaches.
Journal: Engineering Applications of Artificial Intelligence - Volume 26, Issue 1, January 2013, Pages 368–381