کد مقاله | کد نشریه | سال انتشار | مقاله انگلیسی | نسخه تمام متن |
---|---|---|---|---|
381689 | 1437516 | 2006 | 11 صفحه PDF | دانلود رایگان |
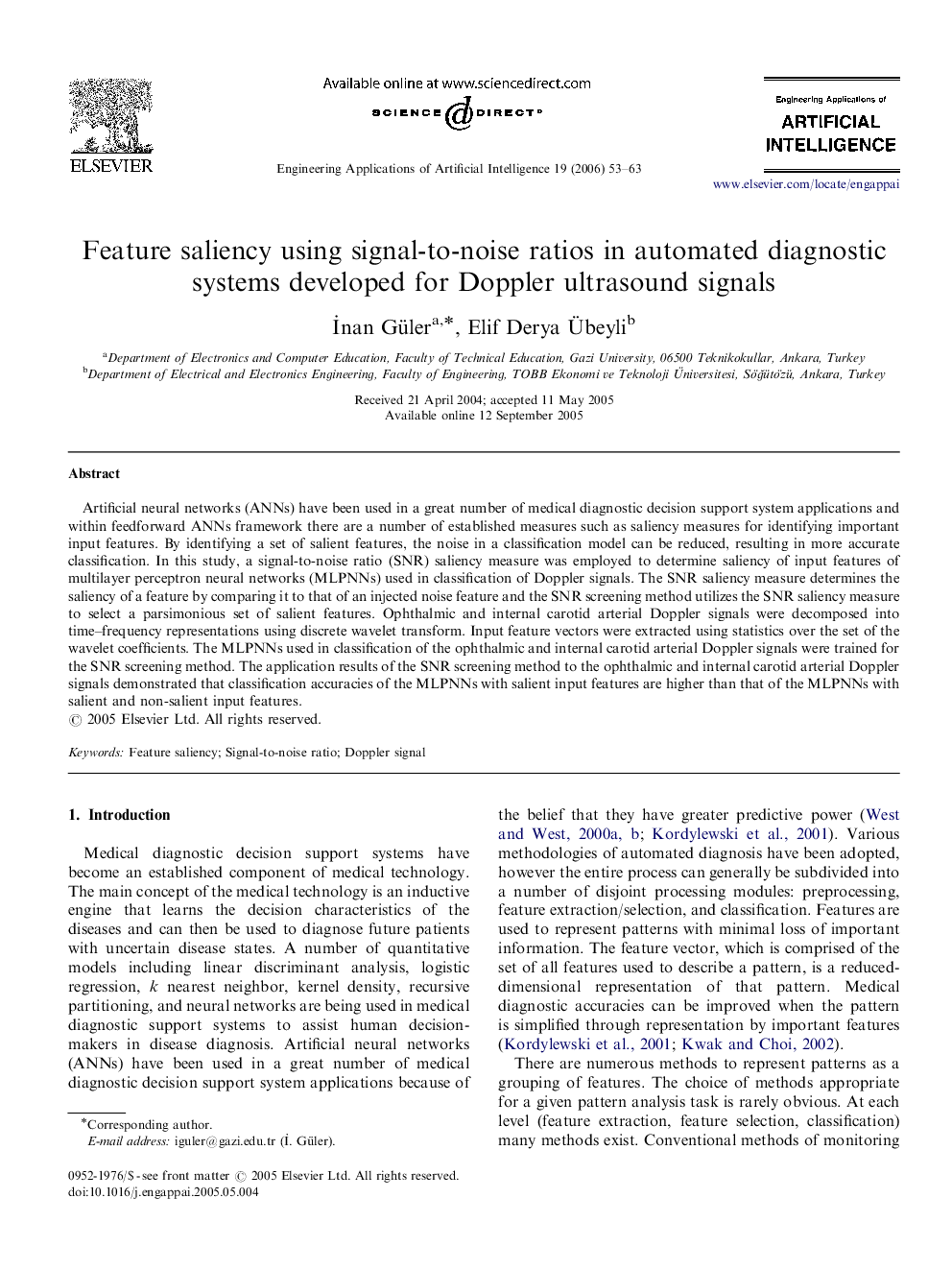
Artificial neural networks (ANNs) have been used in a great number of medical diagnostic decision support system applications and within feedforward ANNs framework there are a number of established measures such as saliency measures for identifying important input features. By identifying a set of salient features, the noise in a classification model can be reduced, resulting in more accurate classification. In this study, a signal-to-noise ratio (SNR) saliency measure was employed to determine saliency of input features of multilayer perceptron neural networks (MLPNNs) used in classification of Doppler signals. The SNR saliency measure determines the saliency of a feature by comparing it to that of an injected noise feature and the SNR screening method utilizes the SNR saliency measure to select a parsimonious set of salient features. Ophthalmic and internal carotid arterial Doppler signals were decomposed into time–frequency representations using discrete wavelet transform. Input feature vectors were extracted using statistics over the set of the wavelet coefficients. The MLPNNs used in classification of the ophthalmic and internal carotid arterial Doppler signals were trained for the SNR screening method. The application results of the SNR screening method to the ophthalmic and internal carotid arterial Doppler signals demonstrated that classification accuracies of the MLPNNs with salient input features are higher than that of the MLPNNs with salient and non-salient input features.
Journal: Engineering Applications of Artificial Intelligence - Volume 19, Issue 1, February 2006, Pages 53–63