کد مقاله | کد نشریه | سال انتشار | مقاله انگلیسی | نسخه تمام متن |
---|---|---|---|---|
382358 | 660760 | 2014 | 11 صفحه PDF | دانلود رایگان |
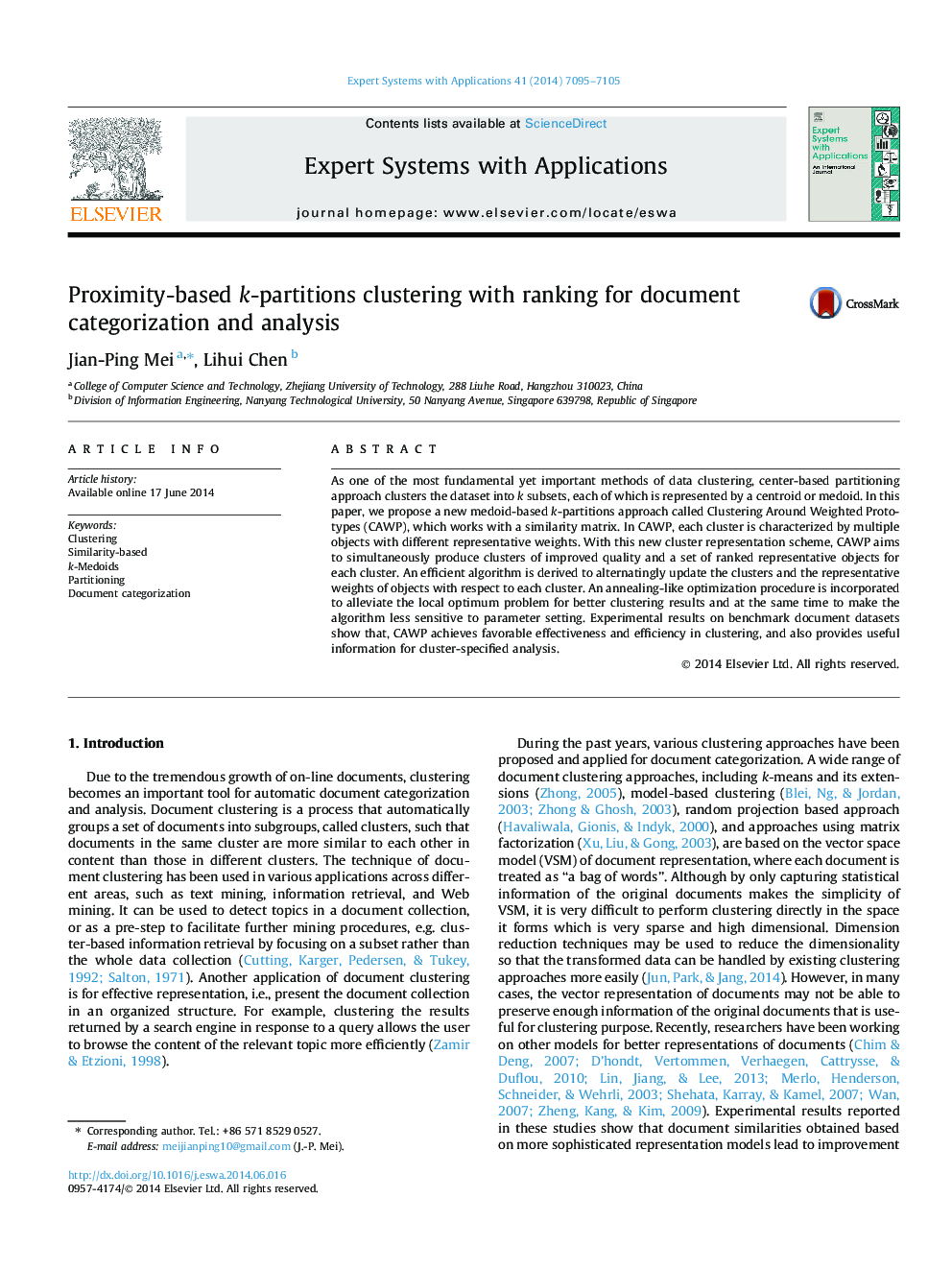
• Weighted medoids are used for cluster representation.
• “Annealing-like” mechanism is introduced to alleviate local optimum.
• Partitions and rankings are obtained by maximizing the objective function.
• Effectiveness and efficiency for document categorization are both reasonable.
As one of the most fundamental yet important methods of data clustering, center-based partitioning approach clusters the dataset into k subsets, each of which is represented by a centroid or medoid. In this paper, we propose a new medoid-based k-partitions approach called Clustering Around Weighted Prototypes (CAWP), which works with a similarity matrix. In CAWP, each cluster is characterized by multiple objects with different representative weights. With this new cluster representation scheme, CAWP aims to simultaneously produce clusters of improved quality and a set of ranked representative objects for each cluster. An efficient algorithm is derived to alternatingly update the clusters and the representative weights of objects with respect to each cluster. An annealing-like optimization procedure is incorporated to alleviate the local optimum problem for better clustering results and at the same time to make the algorithm less sensitive to parameter setting. Experimental results on benchmark document datasets show that, CAWP achieves favorable effectiveness and efficiency in clustering, and also provides useful information for cluster-specified analysis.
Journal: Expert Systems with Applications - Volume 41, Issue 16, 15 November 2014, Pages 7095–7105