کد مقاله | کد نشریه | سال انتشار | مقاله انگلیسی | نسخه تمام متن |
---|---|---|---|---|
383494 | 660824 | 2015 | 12 صفحه PDF | دانلود رایگان |
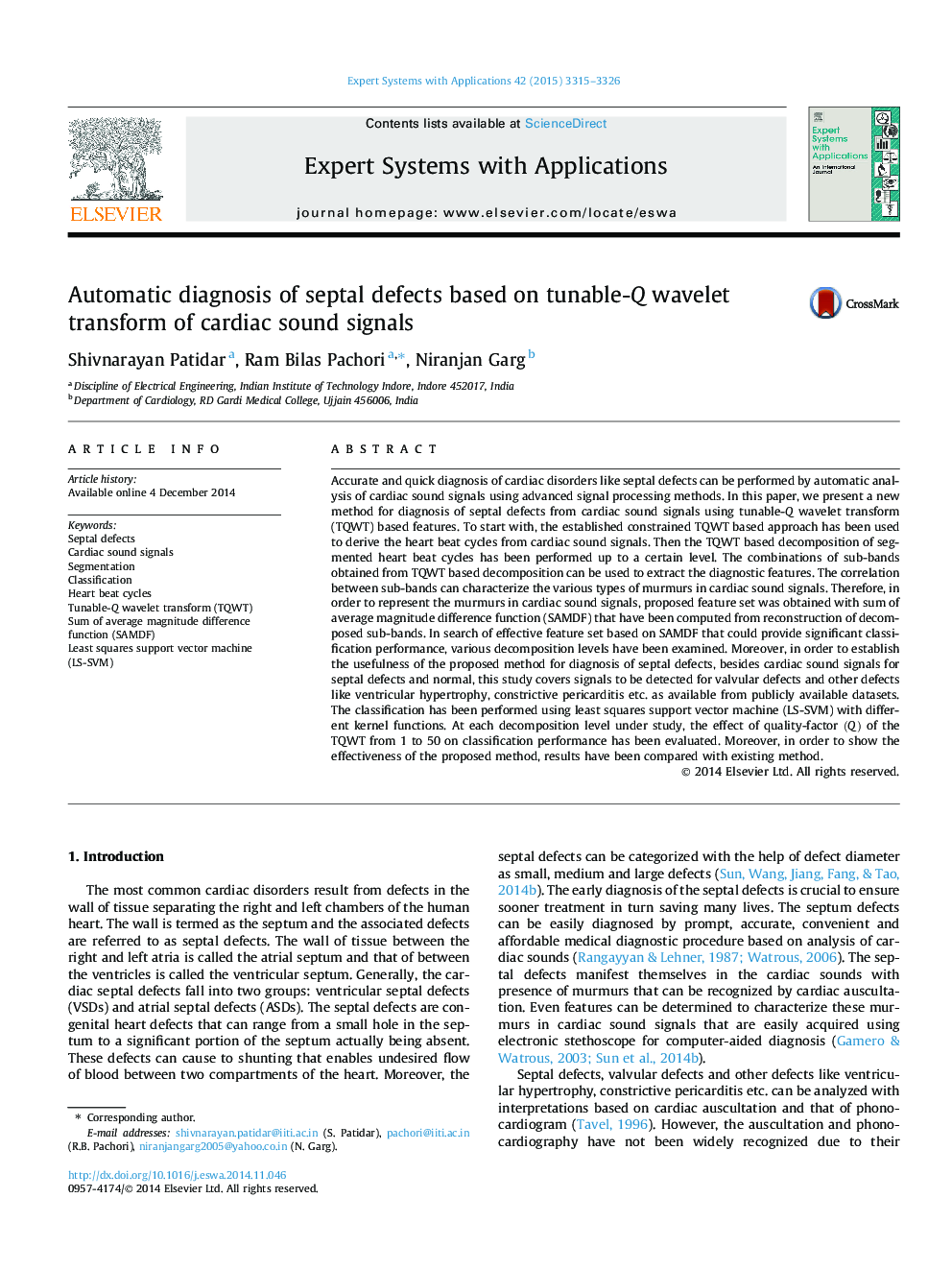
• We propose a new method for diagnosis of septal defects using TQWT.
• New feature set based on SAMDF derived from TQWT has been proposed.
• The effects of Q and decomposition levels on classification performance have been evaluated.
• Results have been evaluated with classification performance evaluation parameters and ROC graphs.
• Performance has been compared with existing TQWT based method with same datasets.
Accurate and quick diagnosis of cardiac disorders like septal defects can be performed by automatic analysis of cardiac sound signals using advanced signal processing methods. In this paper, we present a new method for diagnosis of septal defects from cardiac sound signals using tunable-Q wavelet transform (TQWT) based features. To start with, the established constrained TQWT based approach has been used to derive the heart beat cycles from cardiac sound signals. Then the TQWT based decomposition of segmented heart beat cycles has been performed up to a certain level. The combinations of sub-bands obtained from TQWT based decomposition can be used to extract the diagnostic features. The correlation between sub-bands can characterize the various types of murmurs in cardiac sound signals. Therefore, in order to represent the murmurs in cardiac sound signals, proposed feature set was obtained with sum of average magnitude difference function (SAMDF) that have been computed from reconstruction of decomposed sub-bands. In search of effective feature set based on SAMDF that could provide significant classification performance, various decomposition levels have been examined. Moreover, in order to establish the usefulness of the proposed method for diagnosis of septal defects, besides cardiac sound signals for septal defects and normal, this study covers signals to be detected for valvular defects and other defects like ventricular hypertrophy, constrictive pericarditis etc. as available from publicly available datasets. The classification has been performed using least squares support vector machine (LS-SVM) with different kernel functions. At each decomposition level under study, the effect of quality-factor (Q)(Q) of the TQWT from 1 to 50 on classification performance has been evaluated. Moreover, in order to show the effectiveness of the proposed method, results have been compared with existing method.
Journal: Expert Systems with Applications - Volume 42, Issue 7, 1 May 2015, Pages 3315–3326