کد مقاله | کد نشریه | سال انتشار | مقاله انگلیسی | نسخه تمام متن |
---|---|---|---|---|
383521 | 660824 | 2015 | 10 صفحه PDF | دانلود رایگان |
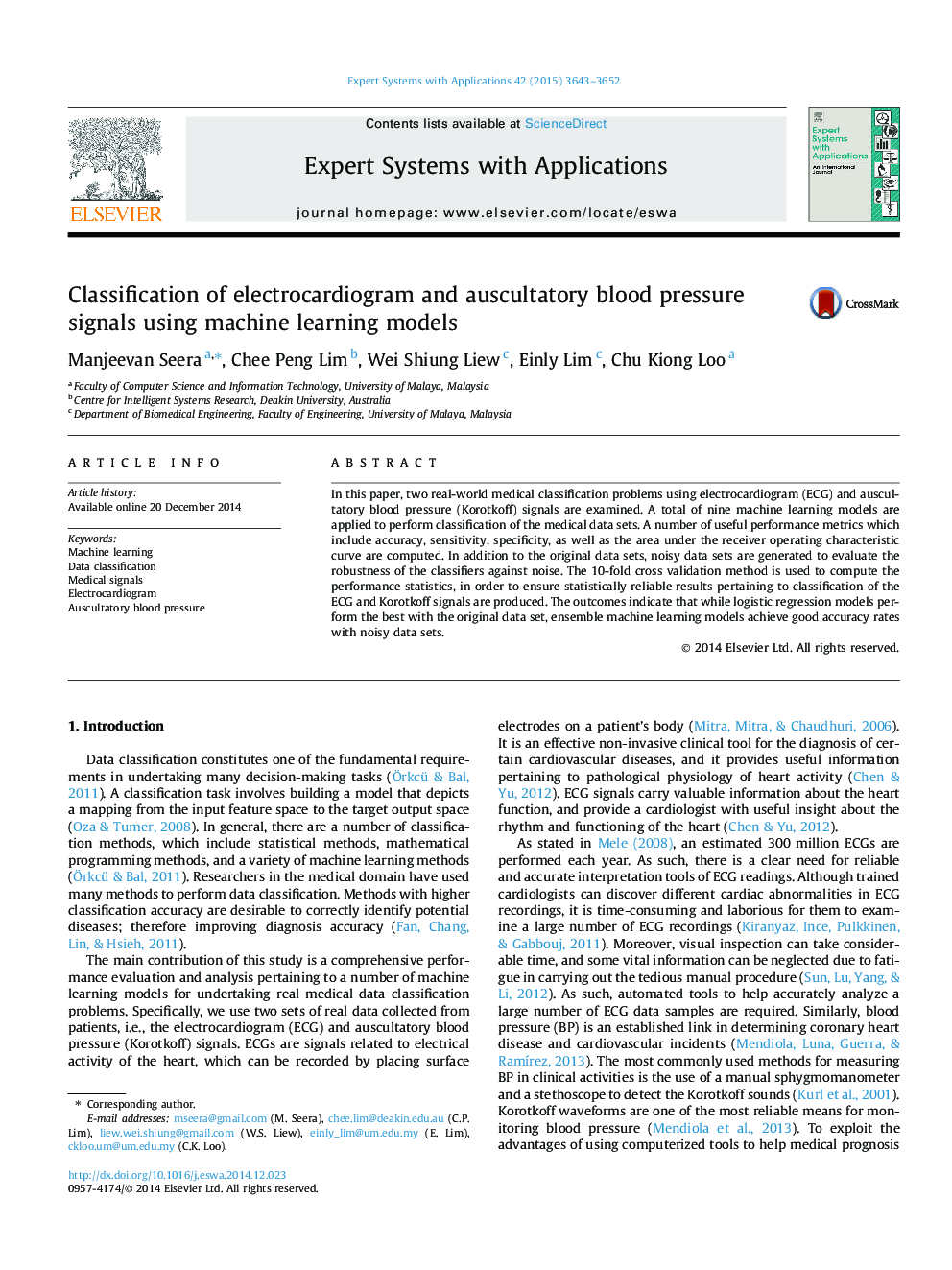
• Medical data classification problems with two real data sets are investigated.
• A literature review on biomedical signal processing techniques is provided.
• The data sets are corrupted with noise to assess the robustness of different models.
• The logistic regression model produces the best results in noise-free environments.
• Ensemble-based learning model yields the best results in noisy environments.
In this paper, two real-world medical classification problems using electrocardiogram (ECG) and auscultatory blood pressure (Korotkoff) signals are examined. A total of nine machine learning models are applied to perform classification of the medical data sets. A number of useful performance metrics which include accuracy, sensitivity, specificity, as well as the area under the receiver operating characteristic curve are computed. In addition to the original data sets, noisy data sets are generated to evaluate the robustness of the classifiers against noise. The 10-fold cross validation method is used to compute the performance statistics, in order to ensure statistically reliable results pertaining to classification of the ECG and Korotkoff signals are produced. The outcomes indicate that while logistic regression models perform the best with the original data set, ensemble machine learning models achieve good accuracy rates with noisy data sets.
Journal: Expert Systems with Applications - Volume 42, Issue 7, 1 May 2015, Pages 3643–3652