کد مقاله | کد نشریه | سال انتشار | مقاله انگلیسی | نسخه تمام متن |
---|---|---|---|---|
383886 | 660836 | 2013 | 11 صفحه PDF | دانلود رایگان |
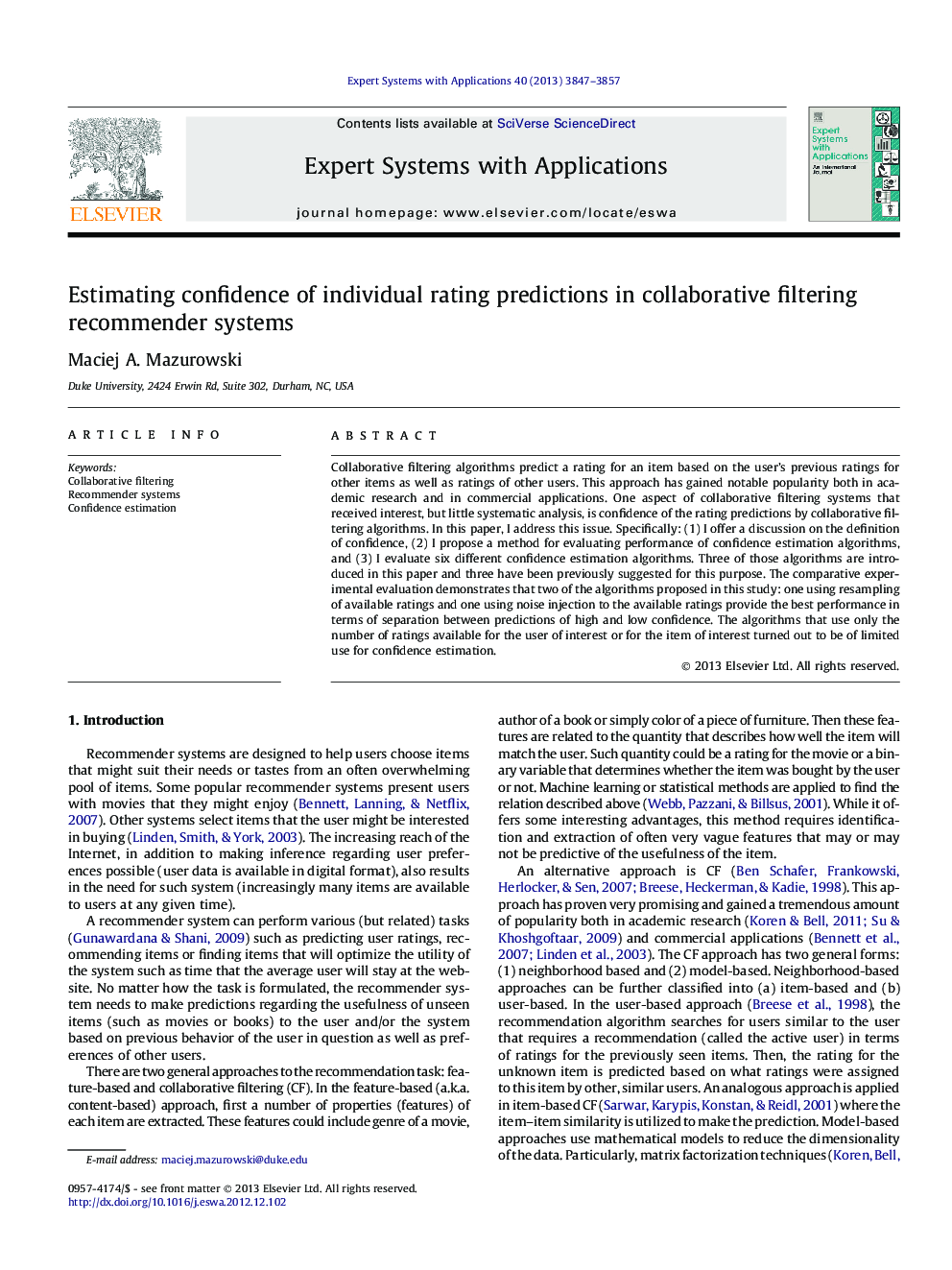
Collaborative filtering algorithms predict a rating for an item based on the user’s previous ratings for other items as well as ratings of other users. This approach has gained notable popularity both in academic research and in commercial applications. One aspect of collaborative filtering systems that received interest, but little systematic analysis, is confidence of the rating predictions by collaborative filtering algorithms. In this paper, I address this issue. Specifically: (1) I offer a discussion on the definition of confidence, (2) I propose a method for evaluating performance of confidence estimation algorithms, and (3) I evaluate six different confidence estimation algorithms. Three of those algorithms are introduced in this paper and three have been previously suggested for this purpose. The comparative experimental evaluation demonstrates that two of the algorithms proposed in this study: one using resampling of available ratings and one using noise injection to the available ratings provide the best performance in terms of separation between predictions of high and low confidence. The algorithms that use only the number of ratings available for the user of interest or for the item of interest turned out to be of limited use for confidence estimation.
► A definition of confidence of predictions in collaborative filtering is presented.
► A method for evaluating performance of confidence estimation algorithms is proposed.
► Three confidence estimation algorithms are proposed.
► Six algorithms including the three proposed here are evaluated experimentally.
► The algorithms proposed here performed better than previously proposed ones.
Journal: Expert Systems with Applications - Volume 40, Issue 10, August 2013, Pages 3847–3857