کد مقاله | کد نشریه | سال انتشار | مقاله انگلیسی | نسخه تمام متن |
---|---|---|---|---|
384405 | 660846 | 2012 | 12 صفحه PDF | دانلود رایگان |
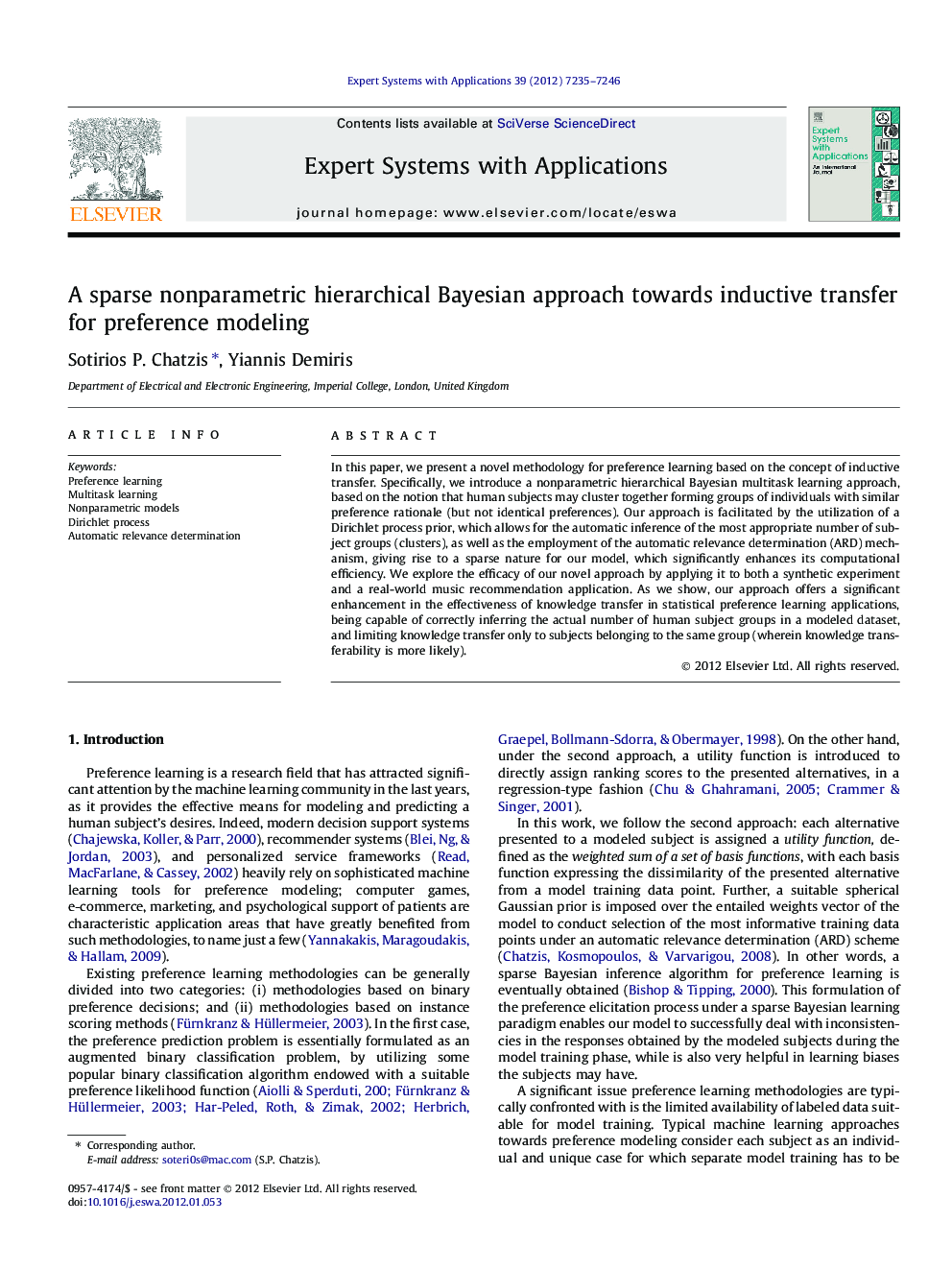
In this paper, we present a novel methodology for preference learning based on the concept of inductive transfer. Specifically, we introduce a nonparametric hierarchical Bayesian multitask learning approach, based on the notion that human subjects may cluster together forming groups of individuals with similar preference rationale (but not identical preferences). Our approach is facilitated by the utilization of a Dirichlet process prior, which allows for the automatic inference of the most appropriate number of subject groups (clusters), as well as the employment of the automatic relevance determination (ARD) mechanism, giving rise to a sparse nature for our model, which significantly enhances its computational efficiency. We explore the efficacy of our novel approach by applying it to both a synthetic experiment and a real-world music recommendation application. As we show, our approach offers a significant enhancement in the effectiveness of knowledge transfer in statistical preference learning applications, being capable of correctly inferring the actual number of human subject groups in a modeled dataset, and limiting knowledge transfer only to subjects belonging to the same group (wherein knowledge transferability is more likely).
► A method for preference learning is proposed.
► The method is based on multitask learning.
► This way, information from multiple users is exploited efficiently.
► A Bayesian inference algorithm for the method is provided.
Journal: Expert Systems with Applications - Volume 39, Issue 8, 15 June 2012, Pages 7235–7246