کد مقاله | کد نشریه | سال انتشار | مقاله انگلیسی | نسخه تمام متن |
---|---|---|---|---|
384443 | 660847 | 2012 | 13 صفحه PDF | دانلود رایگان |
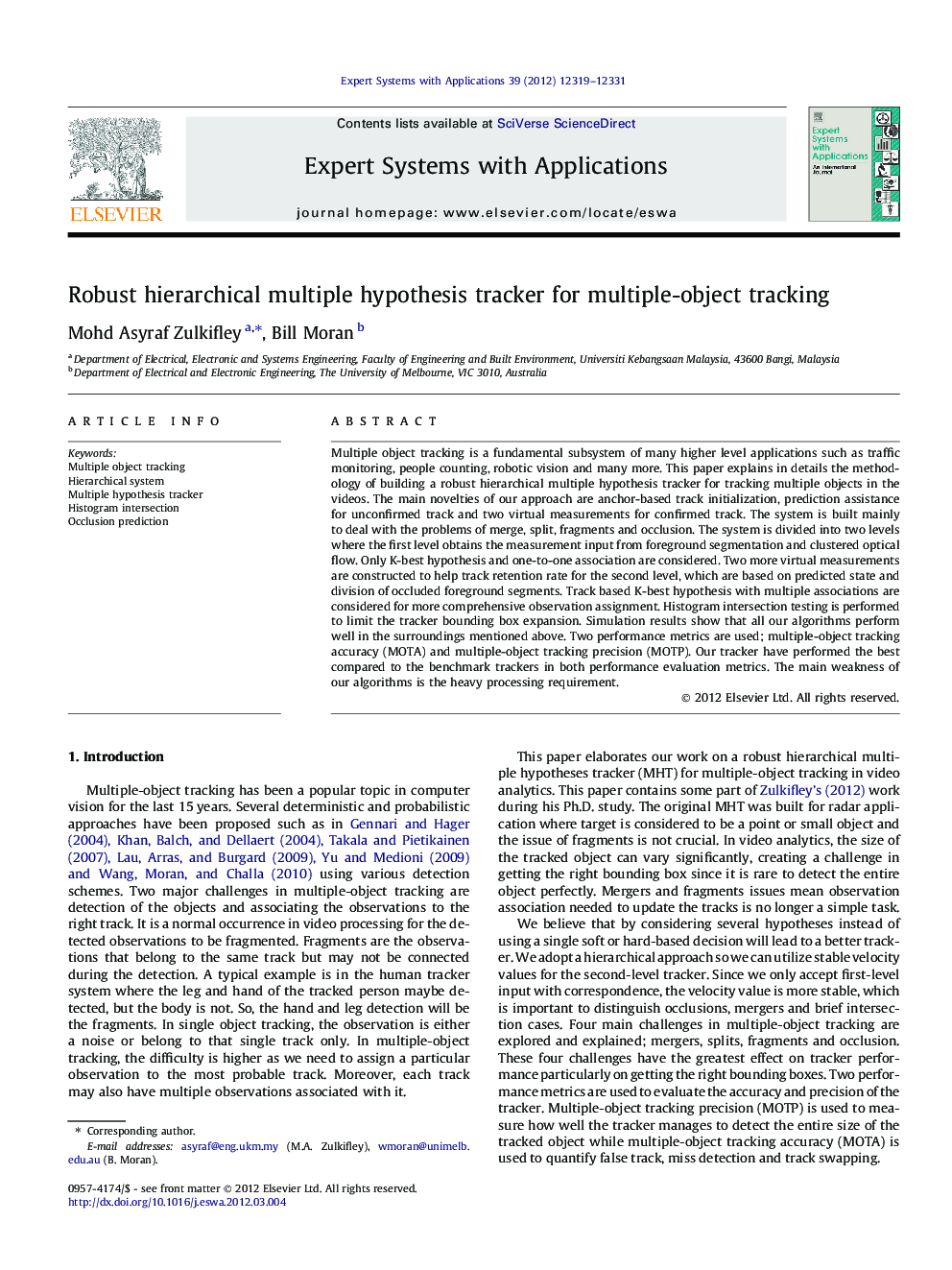
Multiple object tracking is a fundamental subsystem of many higher level applications such as traffic monitoring, people counting, robotic vision and many more. This paper explains in details the methodology of building a robust hierarchical multiple hypothesis tracker for tracking multiple objects in the videos. The main novelties of our approach are anchor-based track initialization, prediction assistance for unconfirmed track and two virtual measurements for confirmed track. The system is built mainly to deal with the problems of merge, split, fragments and occlusion. The system is divided into two levels where the first level obtains the measurement input from foreground segmentation and clustered optical flow. Only K-best hypothesis and one-to-one association are considered. Two more virtual measurements are constructed to help track retention rate for the second level, which are based on predicted state and division of occluded foreground segments. Track based K-best hypothesis with multiple associations are considered for more comprehensive observation assignment. Histogram intersection testing is performed to limit the tracker bounding box expansion. Simulation results show that all our algorithms perform well in the surroundings mentioned above. Two performance metrics are used; multiple-object tracking accuracy (MOTA) and multiple-object tracking precision (MOTP). Our tracker have performed the best compared to the benchmark trackers in both performance evaluation metrics. The main weakness of our algorithms is the heavy processing requirement.
► Our robust tracker has improved the tracking accuracy especially for these four cases of split, merge, occlusion and fragment.
► Two levels of association are implemented where the first level focuses of obtaining stable velocity values while multiple associations are utilized for better observation assignment.
► Occlusion predictor is used to distinguish merge, occlusion and brief interference.
► Virtual measurements are introduced to help track retention rate.
► Histogram intersection is used to limit the tracker’s size expansion.
Journal: Expert Systems with Applications - Volume 39, Issue 16, 15 November 2012, Pages 12319–12331