کد مقاله | کد نشریه | سال انتشار | مقاله انگلیسی | نسخه تمام متن |
---|---|---|---|---|
384446 | 660847 | 2012 | 9 صفحه PDF | دانلود رایگان |
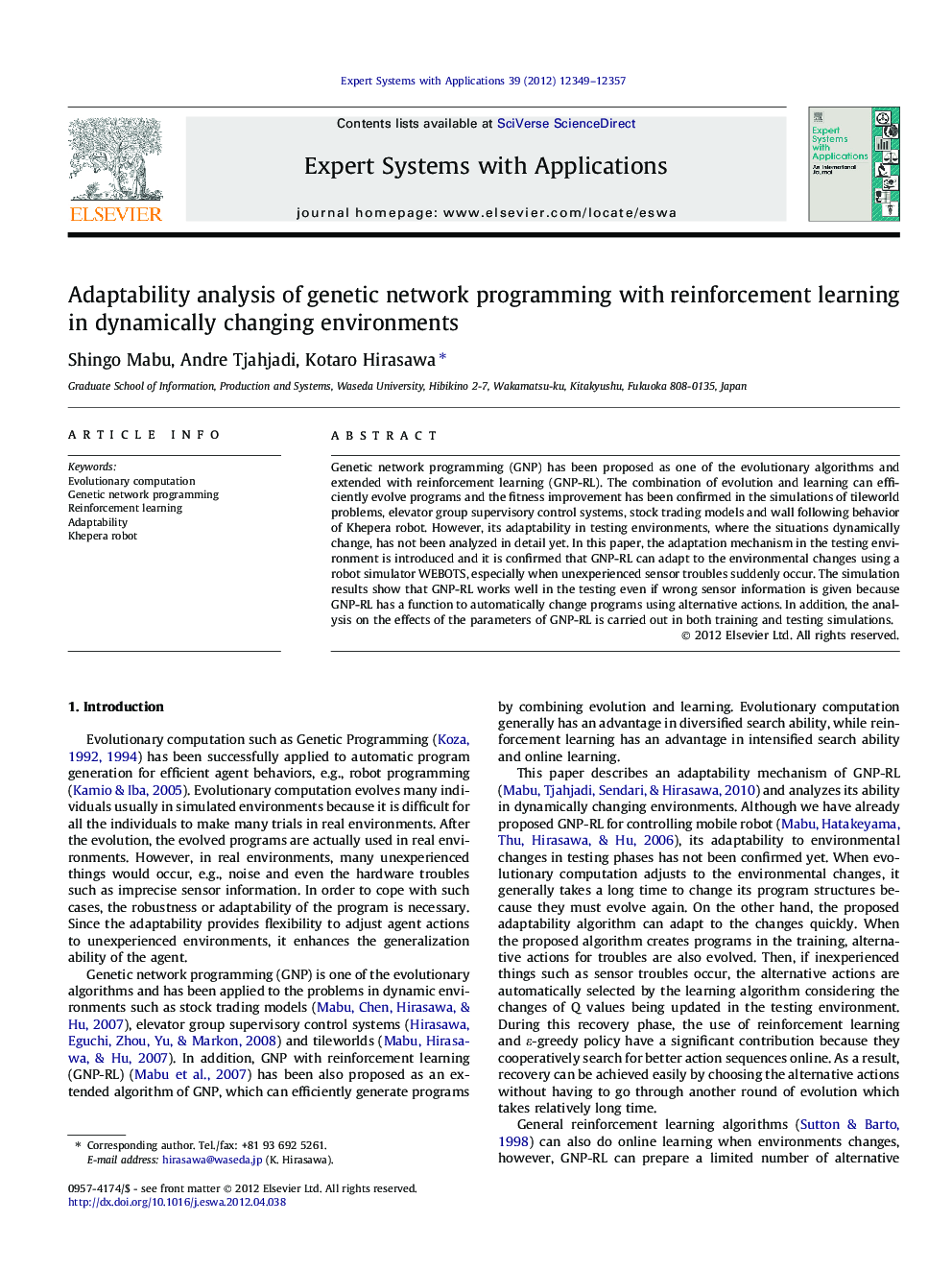
Genetic network programming (GNP) has been proposed as one of the evolutionary algorithms and extended with reinforcement learning (GNP-RL). The combination of evolution and learning can efficiently evolve programs and the fitness improvement has been confirmed in the simulations of tileworld problems, elevator group supervisory control systems, stock trading models and wall following behavior of Khepera robot. However, its adaptability in testing environments, where the situations dynamically change, has not been analyzed in detail yet. In this paper, the adaptation mechanism in the testing environment is introduced and it is confirmed that GNP-RL can adapt to the environmental changes using a robot simulator WEBOTS, especially when unexperienced sensor troubles suddenly occur. The simulation results show that GNP-RL works well in the testing even if wrong sensor information is given because GNP-RL has a function to automatically change programs using alternative actions. In addition, the analysis on the effects of the parameters of GNP-RL is carried out in both training and testing simulations.
► We proposed an adaptive learning mechanism using reinforcement learning.
► The adaptability is examined when some sensors are suddenly broken.
► Faulty sensors are indirectly detected through the changes of Q values.
► The size of Q table is compact, so the quick recovery from troubles is possible.
Journal: Expert Systems with Applications - Volume 39, Issue 16, 15 November 2012, Pages 12349–12357