کد مقاله | کد نشریه | سال انتشار | مقاله انگلیسی | نسخه تمام متن |
---|---|---|---|---|
385119 | 660860 | 2011 | 8 صفحه PDF | دانلود رایگان |
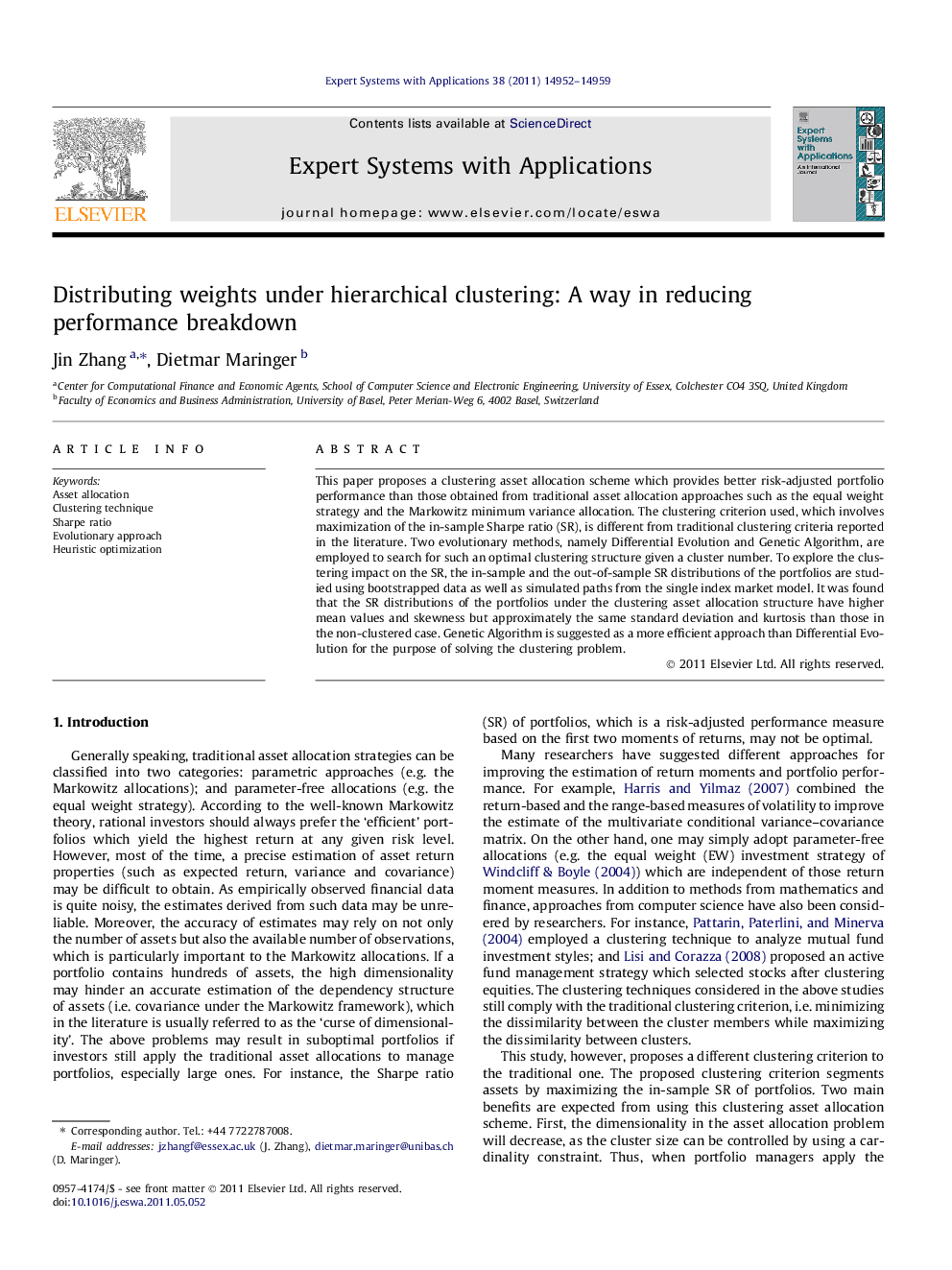
This paper proposes a clustering asset allocation scheme which provides better risk-adjusted portfolio performance than those obtained from traditional asset allocation approaches such as the equal weight strategy and the Markowitz minimum variance allocation. The clustering criterion used, which involves maximization of the in-sample Sharpe ratio (SR), is different from traditional clustering criteria reported in the literature. Two evolutionary methods, namely Differential Evolution and Genetic Algorithm, are employed to search for such an optimal clustering structure given a cluster number. To explore the clustering impact on the SR, the in-sample and the out-of-sample SR distributions of the portfolios are studied using bootstrapped data as well as simulated paths from the single index market model. It was found that the SR distributions of the portfolios under the clustering asset allocation structure have higher mean values and skewness but approximately the same standard deviation and kurtosis than those in the non-clustered case. Genetic Algorithm is suggested as a more efficient approach than Differential Evolution for the purpose of solving the clustering problem.
► We introduce a clustering scheme to improve portfolio Sharpe ratio.
► Mean and Skewness of Sharpe ratio can be improved by using the clustering scheme.
► Genetic Algorithm is apt at finding an optimal clustering structure.
► Clustering asset helps to improve portfolio risk-adjusted performance.
► Sharpe ratio maximization can be considered as a suitable clustering criterion.
Journal: Expert Systems with Applications - Volume 38, Issue 12, November–December 2011, Pages 14952–14959