کد مقاله | کد نشریه | سال انتشار | مقاله انگلیسی | نسخه تمام متن |
---|---|---|---|---|
385184 | 660863 | 2012 | 14 صفحه PDF | دانلود رایگان |
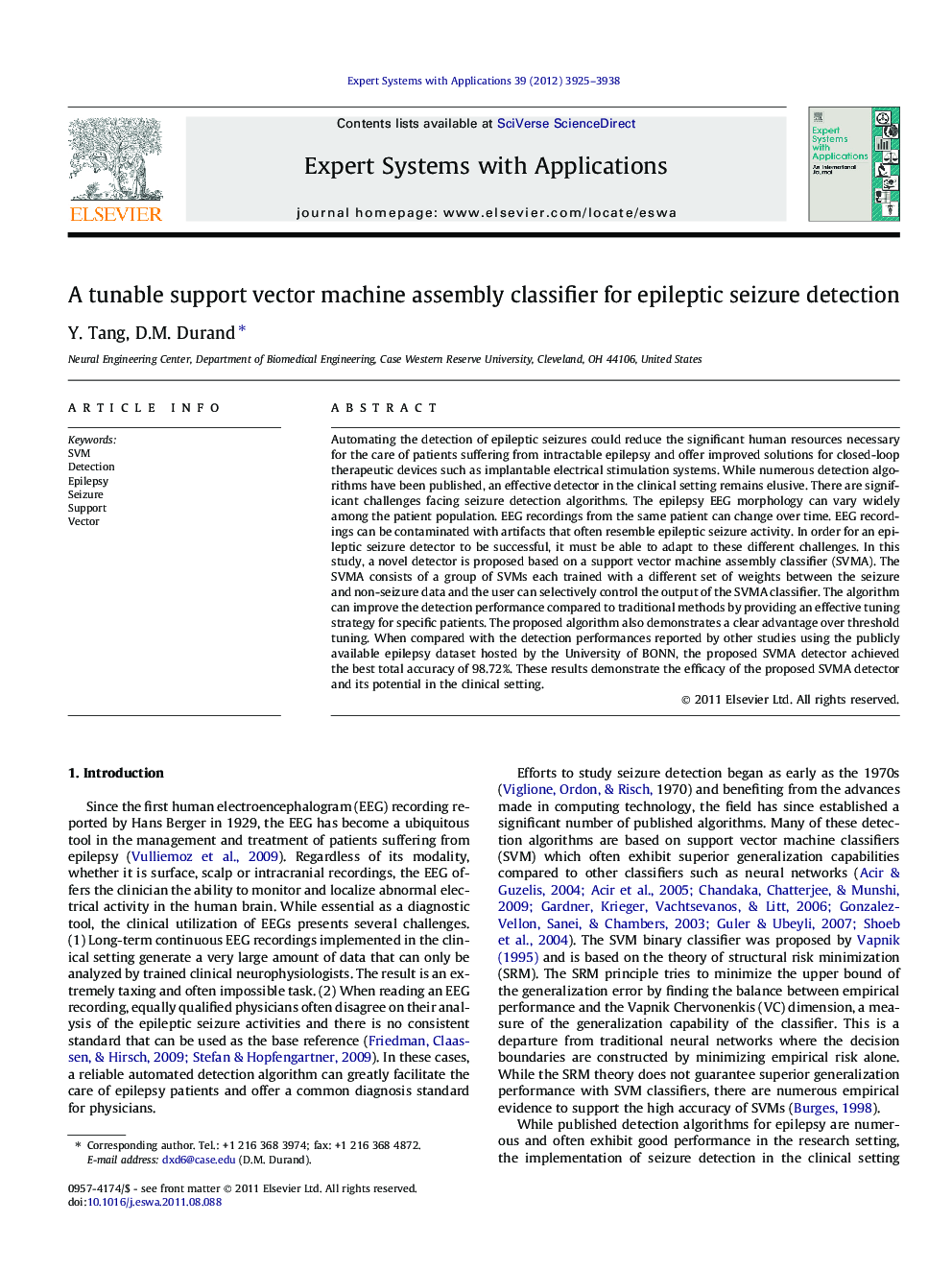
Automating the detection of epileptic seizures could reduce the significant human resources necessary for the care of patients suffering from intractable epilepsy and offer improved solutions for closed-loop therapeutic devices such as implantable electrical stimulation systems. While numerous detection algorithms have been published, an effective detector in the clinical setting remains elusive. There are significant challenges facing seizure detection algorithms. The epilepsy EEG morphology can vary widely among the patient population. EEG recordings from the same patient can change over time. EEG recordings can be contaminated with artifacts that often resemble epileptic seizure activity. In order for an epileptic seizure detector to be successful, it must be able to adapt to these different challenges. In this study, a novel detector is proposed based on a support vector machine assembly classifier (SVMA). The SVMA consists of a group of SVMs each trained with a different set of weights between the seizure and non-seizure data and the user can selectively control the output of the SVMA classifier. The algorithm can improve the detection performance compared to traditional methods by providing an effective tuning strategy for specific patients. The proposed algorithm also demonstrates a clear advantage over threshold tuning. When compared with the detection performances reported by other studies using the publicly available epilepsy dataset hosted by the University of BONN, the proposed SVMA detector achieved the best total accuracy of 98.72%. These results demonstrate the efficacy of the proposed SVMA detector and its potential in the clinical setting.
► Implemented a novel support vector machine assembly epileptic seizure detector.
► Demonstrated easy patient specific tuning with the detector.
► Demonstrated superior tuning performance versus threshold tuning.
► Achieved the highest total accuracy within published results on the same dataset.
Journal: Expert Systems with Applications - Volume 39, Issue 4, March 2012, Pages 3925–3938