کد مقاله | کد نشریه | سال انتشار | مقاله انگلیسی | نسخه تمام متن |
---|---|---|---|---|
385597 | 660868 | 2011 | 14 صفحه PDF | دانلود رایگان |
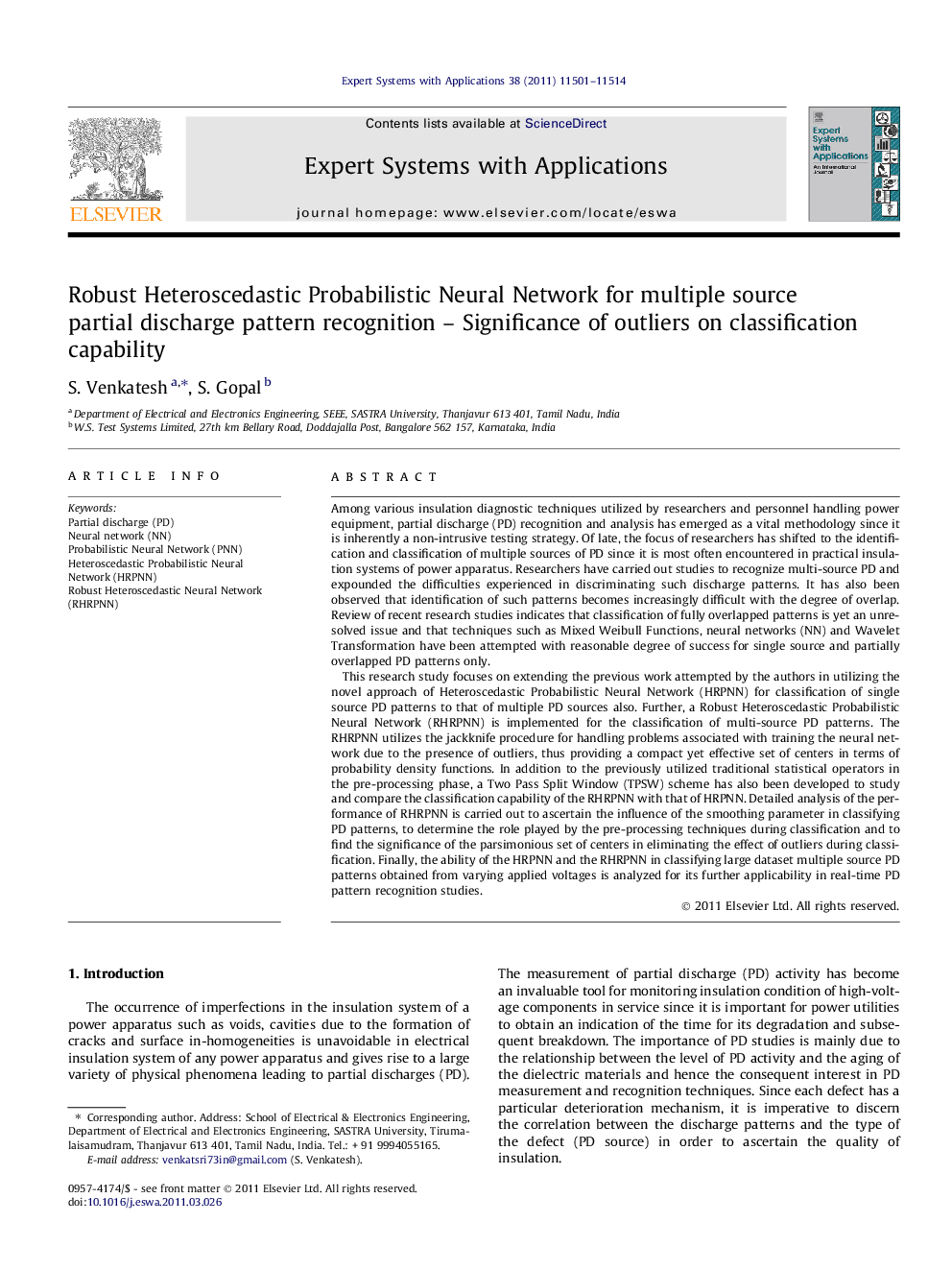
Among various insulation diagnostic techniques utilized by researchers and personnel handling power equipment, partial discharge (PD) recognition and analysis has emerged as a vital methodology since it is inherently a non-intrusive testing strategy. Of late, the focus of researchers has shifted to the identification and classification of multiple sources of PD since it is most often encountered in practical insulation systems of power apparatus. Researchers have carried out studies to recognize multi-source PD and expounded the difficulties experienced in discriminating such discharge patterns. It has also been observed that identification of such patterns becomes increasingly difficult with the degree of overlap. Review of recent research studies indicates that classification of fully overlapped patterns is yet an unresolved issue and that techniques such as Mixed Weibull Functions, neural networks (NN) and Wavelet Transformation have been attempted with reasonable degree of success for single source and partially overlapped PD patterns only.This research study focuses on extending the previous work attempted by the authors in utilizing the novel approach of Heteroscedastic Probabilistic Neural Network (HRPNN) for classification of single source PD patterns to that of multiple PD sources also. Further, a Robust Heteroscedastic Probabilistic Neural Network (RHRPNN) is implemented for the classification of multi-source PD patterns. The RHRPNN utilizes the jackknife procedure for handling problems associated with training the neural network due to the presence of outliers, thus providing a compact yet effective set of centers in terms of probability density functions. In addition to the previously utilized traditional statistical operators in the pre-processing phase, a Two Pass Split Window (TPSW) scheme has also been developed to study and compare the classification capability of the RHRPNN with that of HRPNN. Detailed analysis of the performance of RHRPNN is carried out to ascertain the influence of the smoothing parameter in classifying PD patterns, to determine the role played by the pre-processing techniques during classification and to find the significance of the parsimonious set of centers in eliminating the effect of outliers during classification. Finally, the ability of the HRPNN and the RHRPNN in classifying large dataset multiple source PD patterns obtained from varying applied voltages is analyzed for its further applicability in real-time PD pattern recognition studies.
► Robust Heteroscedastic Probabilistic Neural Network (RHRPNN) for multi-source PD recognition.
► Novel approach of utilizing Two Pass Split Window pre-processing with Robust HRPNN.
► Substantial classification rate using TPSW preprocessing scheme with HRPNN and RHRPNN.
► Noteworthy classification of large data multi-source PD for varying applied voltages.
► More frugal yet optimum centers pertaining to PD classes in RHRPNN compared to HRPNN.
Journal: Expert Systems with Applications - Volume 38, Issue 9, September 2011, Pages 11501–11514