کد مقاله | کد نشریه | سال انتشار | مقاله انگلیسی | نسخه تمام متن |
---|---|---|---|---|
386038 | 660876 | 2011 | 12 صفحه PDF | دانلود رایگان |
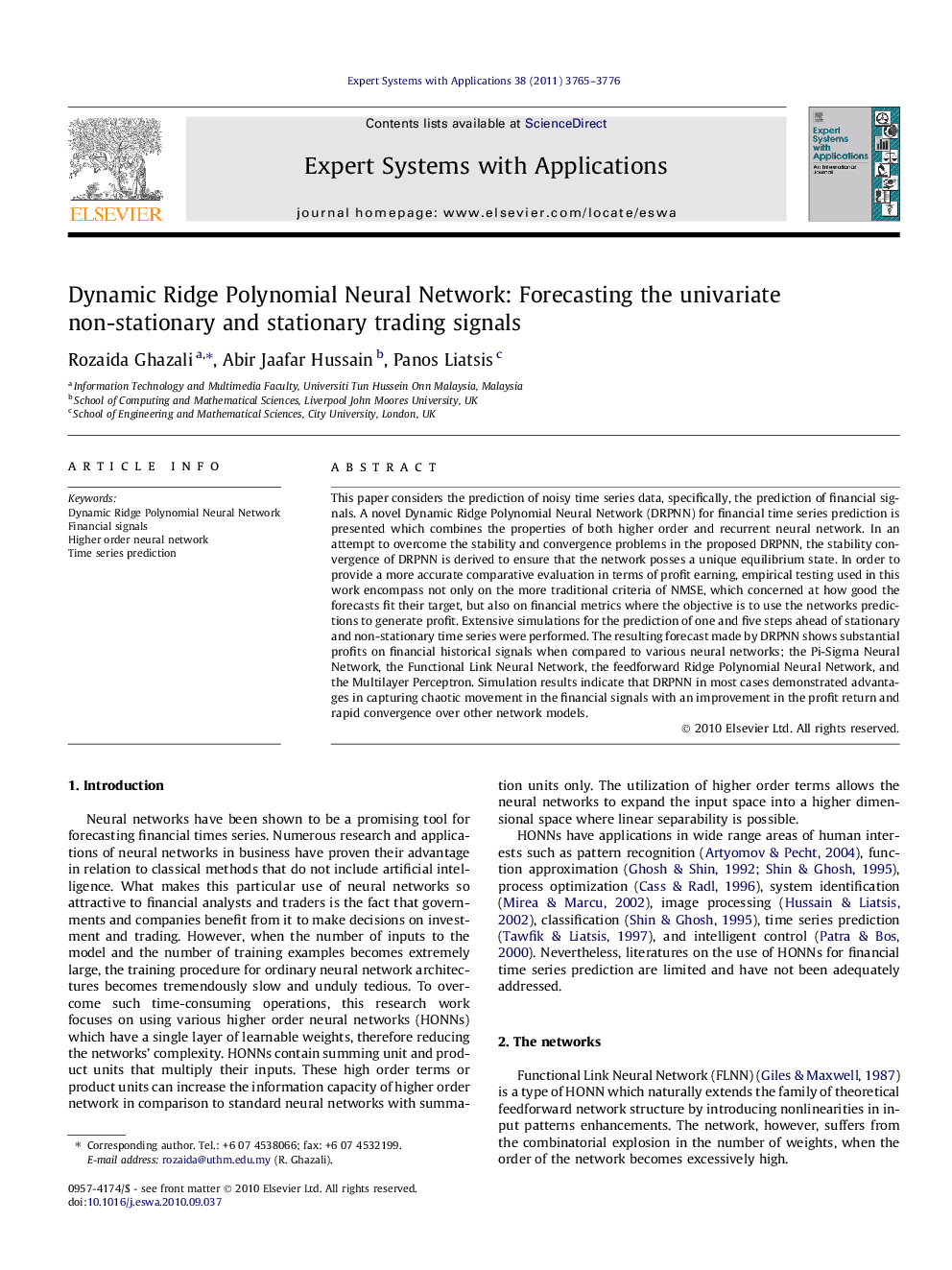
This paper considers the prediction of noisy time series data, specifically, the prediction of financial signals. A novel Dynamic Ridge Polynomial Neural Network (DRPNN) for financial time series prediction is presented which combines the properties of both higher order and recurrent neural network. In an attempt to overcome the stability and convergence problems in the proposed DRPNN, the stability convergence of DRPNN is derived to ensure that the network posses a unique equilibrium state. In order to provide a more accurate comparative evaluation in terms of profit earning, empirical testing used in this work encompass not only on the more traditional criteria of NMSE, which concerned at how good the forecasts fit their target, but also on financial metrics where the objective is to use the networks predictions to generate profit. Extensive simulations for the prediction of one and five steps ahead of stationary and non-stationary time series were performed. The resulting forecast made by DRPNN shows substantial profits on financial historical signals when compared to various neural networks; the Pi-Sigma Neural Network, the Functional Link Neural Network, the feedforward Ridge Polynomial Neural Network, and the Multilayer Perceptron. Simulation results indicate that DRPNN in most cases demonstrated advantages in capturing chaotic movement in the financial signals with an improvement in the profit return and rapid convergence over other network models.
Research highlights
► DRPNN manifests highly nonlinear dynamical behavior induced by the recurrent feedback.
► DRPNNs show a rapid learning, considering the MSE curves end up at less than 90 epochs.
► DRPNNs outperformed other networks in most cases with higher profit return and lower NMSE.
Journal: Expert Systems with Applications - Volume 38, Issue 4, April 2011, Pages 3765–3776