کد مقاله | کد نشریه | سال انتشار | مقاله انگلیسی | نسخه تمام متن |
---|---|---|---|---|
386800 | 660891 | 2014 | 11 صفحه PDF | دانلود رایگان |
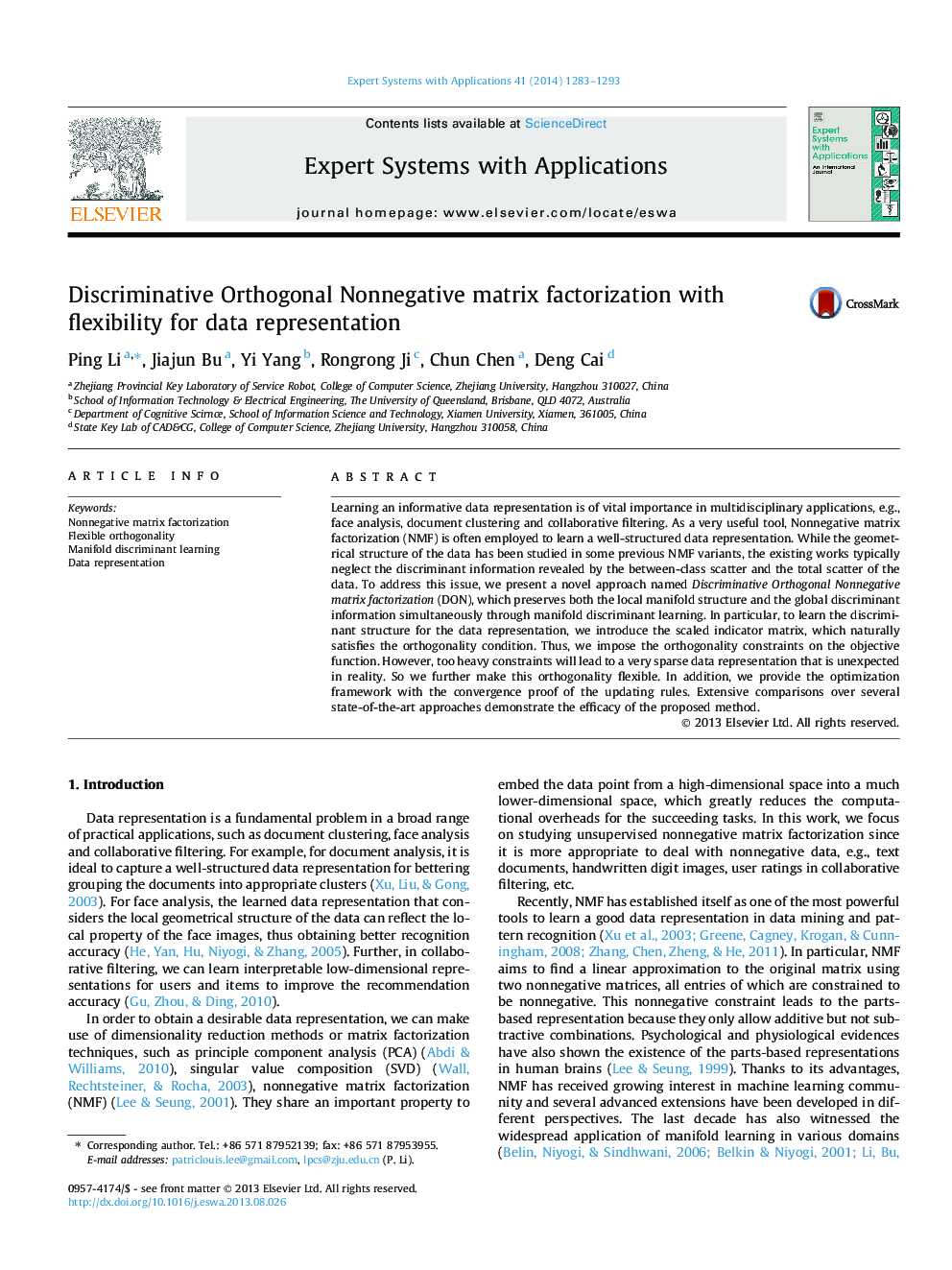
• We propose the Discriminative Orthogonal NMF method for data representation.
• Our method respects the locally geometrical structure of the data.
• Our method employs the global discriminant information of the data.
• We make the method be more adaptive with flexible orthogonality regularization.
• Extensive experiments suggest the superiority of the proposed method.
Learning an informative data representation is of vital importance in multidisciplinary applications, e.g., face analysis, document clustering and collaborative filtering. As a very useful tool, Nonnegative matrix factorization (NMF) is often employed to learn a well-structured data representation. While the geometrical structure of the data has been studied in some previous NMF variants, the existing works typically neglect the discriminant information revealed by the between-class scatter and the total scatter of the data. To address this issue, we present a novel approach named Discriminative Orthogonal Nonnegative matrix factorization (DON), which preserves both the local manifold structure and the global discriminant information simultaneously through manifold discriminant learning. In particular, to learn the discriminant structure for the data representation, we introduce the scaled indicator matrix, which naturally satisfies the orthogonality condition. Thus, we impose the orthogonality constraints on the objective function. However, too heavy constraints will lead to a very sparse data representation that is unexpected in reality. So we further make this orthogonality flexible. In addition, we provide the optimization framework with the convergence proof of the updating rules. Extensive comparisons over several state-of-the-art approaches demonstrate the efficacy of the proposed method.
Journal: Expert Systems with Applications - Volume 41, Issue 4, Part 1, March 2014, Pages 1283–1293