کد مقاله | کد نشریه | سال انتشار | مقاله انگلیسی | نسخه تمام متن |
---|---|---|---|---|
386979 | 660893 | 2009 | 6 صفحه PDF | دانلود رایگان |
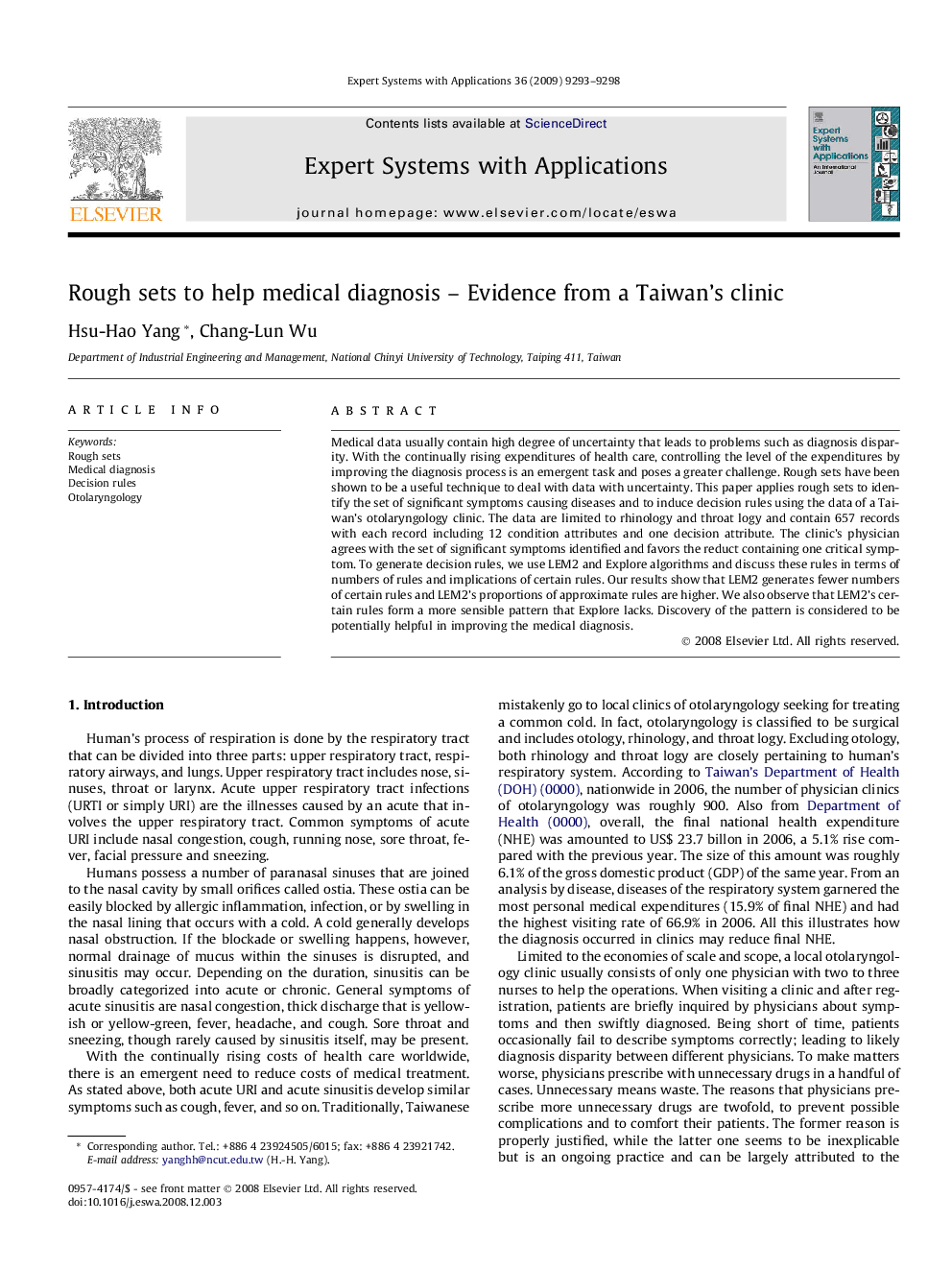
Medical data usually contain high degree of uncertainty that leads to problems such as diagnosis disparity. With the continually rising expenditures of health care, controlling the level of the expenditures by improving the diagnosis process is an emergent task and poses a greater challenge. Rough sets have been shown to be a useful technique to deal with data with uncertainty. This paper applies rough sets to identify the set of significant symptoms causing diseases and to induce decision rules using the data of a Taiwan’s otolaryngology clinic. The data are limited to rhinology and throat logy and contain 657 records with each record including 12 condition attributes and one decision attribute. The clinic’s physician agrees with the set of significant symptoms identified and favors the reduct containing one critical symptom. To generate decision rules, we use LEM2 and Explore algorithms and discuss these rules in terms of numbers of rules and implications of certain rules. Our results show that LEM2 generates fewer numbers of certain rules and LEM2’s proportions of approximate rules are higher. We also observe that LEM2’s certain rules form a more sensible pattern that Explore lacks. Discovery of the pattern is considered to be potentially helpful in improving the medical diagnosis.
Journal: Expert Systems with Applications - Volume 36, Issue 5, July 2009, Pages 9293–9298