کد مقاله | کد نشریه | سال انتشار | مقاله انگلیسی | نسخه تمام متن |
---|---|---|---|---|
387454 | 660902 | 2009 | 6 صفحه PDF | دانلود رایگان |
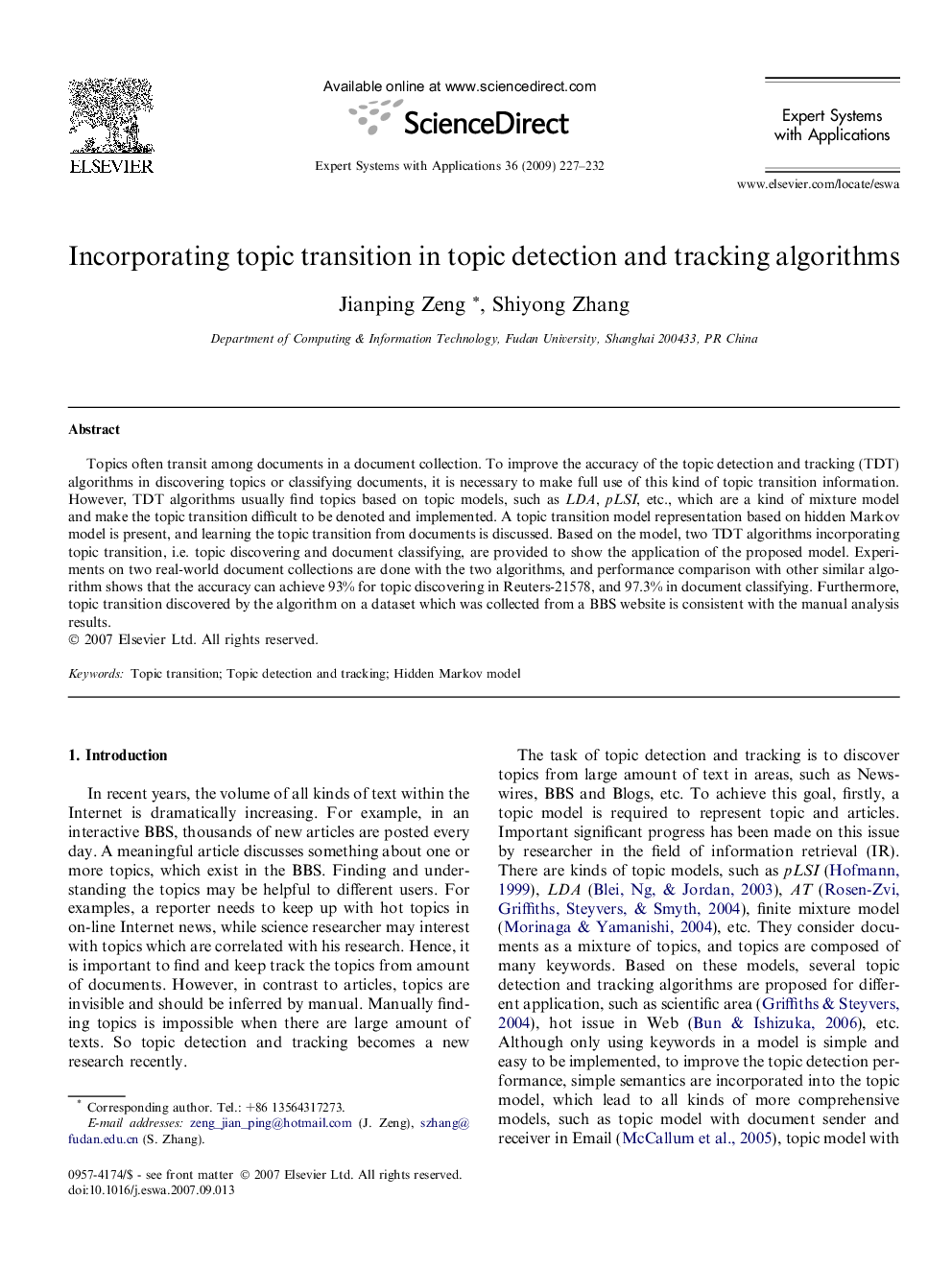
Topics often transit among documents in a document collection. To improve the accuracy of the topic detection and tracking (TDT) algorithms in discovering topics or classifying documents, it is necessary to make full use of this kind of topic transition information. However, TDT algorithms usually find topics based on topic models, such as LDA, pLSI, etc., which are a kind of mixture model and make the topic transition difficult to be denoted and implemented. A topic transition model representation based on hidden Markov model is present, and learning the topic transition from documents is discussed. Based on the model, two TDT algorithms incorporating topic transition, i.e. topic discovering and document classifying, are provided to show the application of the proposed model. Experiments on two real-world document collections are done with the two algorithms, and performance comparison with other similar algorithm shows that the accuracy can achieve 93% for topic discovering in Reuters-21578, and 97.3% in document classifying. Furthermore, topic transition discovered by the algorithm on a dataset which was collected from a BBS website is consistent with the manual analysis results.
Journal: Expert Systems with Applications - Volume 36, Issue 1, January 2009, Pages 227–232