کد مقاله | کد نشریه | سال انتشار | مقاله انگلیسی | نسخه تمام متن |
---|---|---|---|---|
388468 | 660926 | 2011 | 19 صفحه PDF | دانلود رایگان |
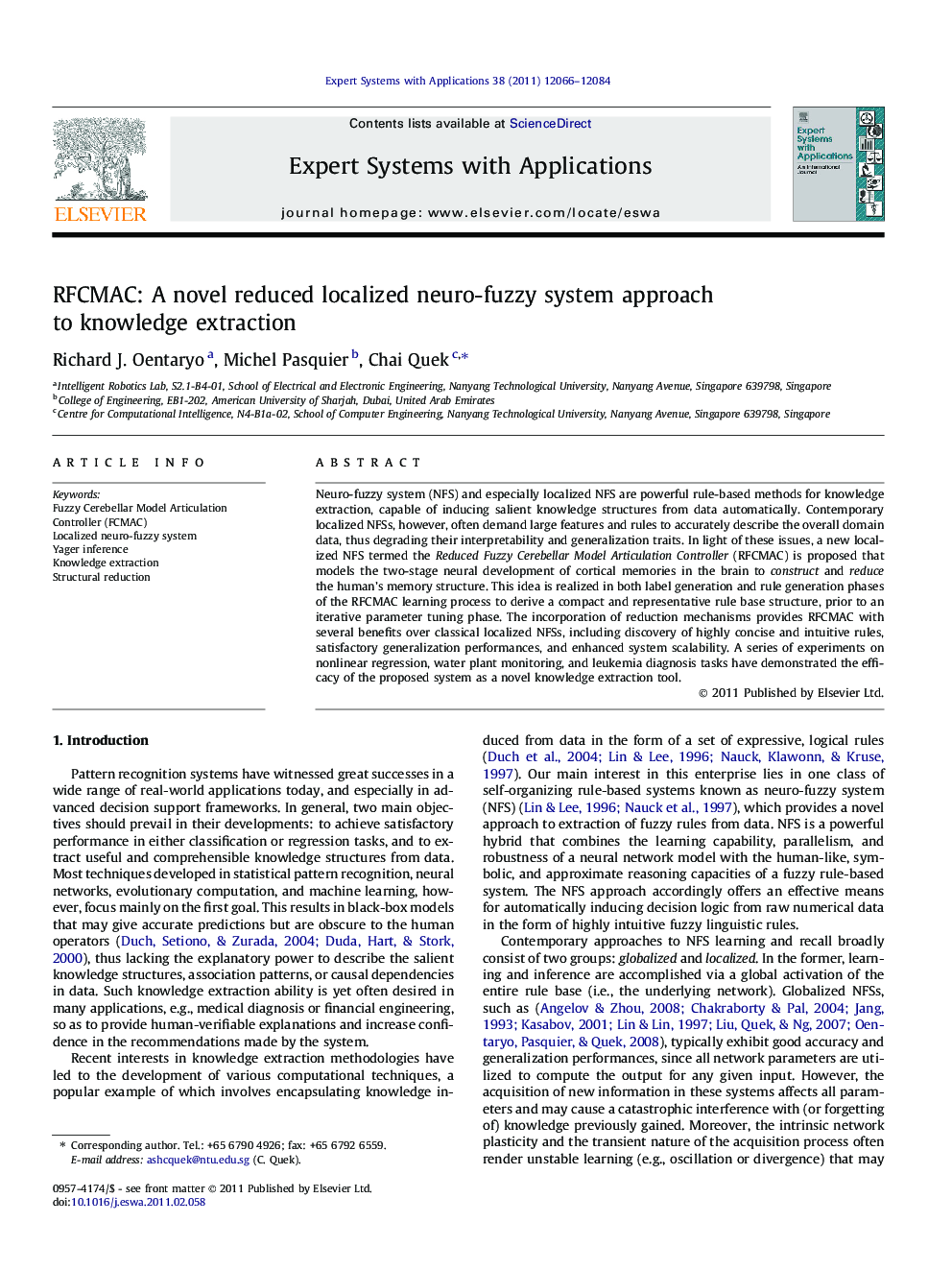
Neuro-fuzzy system (NFS) and especially localized NFS are powerful rule-based methods for knowledge extraction, capable of inducing salient knowledge structures from data automatically. Contemporary localized NFSs, however, often demand large features and rules to accurately describe the overall domain data, thus degrading their interpretability and generalization traits. In light of these issues, a new localized NFS termed the Reduced Fuzzy Cerebellar Model Articulation Controller (RFCMAC) is proposed that models the two-stage neural development of cortical memories in the brain to construct and reduce the human’s memory structure. This idea is realized in both label generation and rule generation phases of the RFCMAC learning process to derive a compact and representative rule base structure, prior to an iterative parameter tuning phase. The incorporation of reduction mechanisms provides RFCMAC with several benefits over classical localized NFSs, including discovery of highly concise and intuitive rules, satisfactory generalization performances, and enhanced system scalability. A series of experiments on nonlinear regression, water plant monitoring, and leukemia diagnosis tasks have demonstrated the efficacy of the proposed system as a novel knowledge extraction tool.
► Localized neuro-fuzzy system as a powerful rule-based tool for knowledge extraction.
► Construct-then-reduce approach enables extraction of concise, interpretable rules.
► Rule reduction mechanism improves system generalization and scalability traits.
► Experiments on complex regression/classification tasks confirm our model’s efficacy.
Journal: Expert Systems with Applications - Volume 38, Issue 10, 15 September 2011, Pages 12066–12084