کد مقاله | کد نشریه | سال انتشار | مقاله انگلیسی | نسخه تمام متن |
---|---|---|---|---|
388491 | 660926 | 2011 | 9 صفحه PDF | دانلود رایگان |
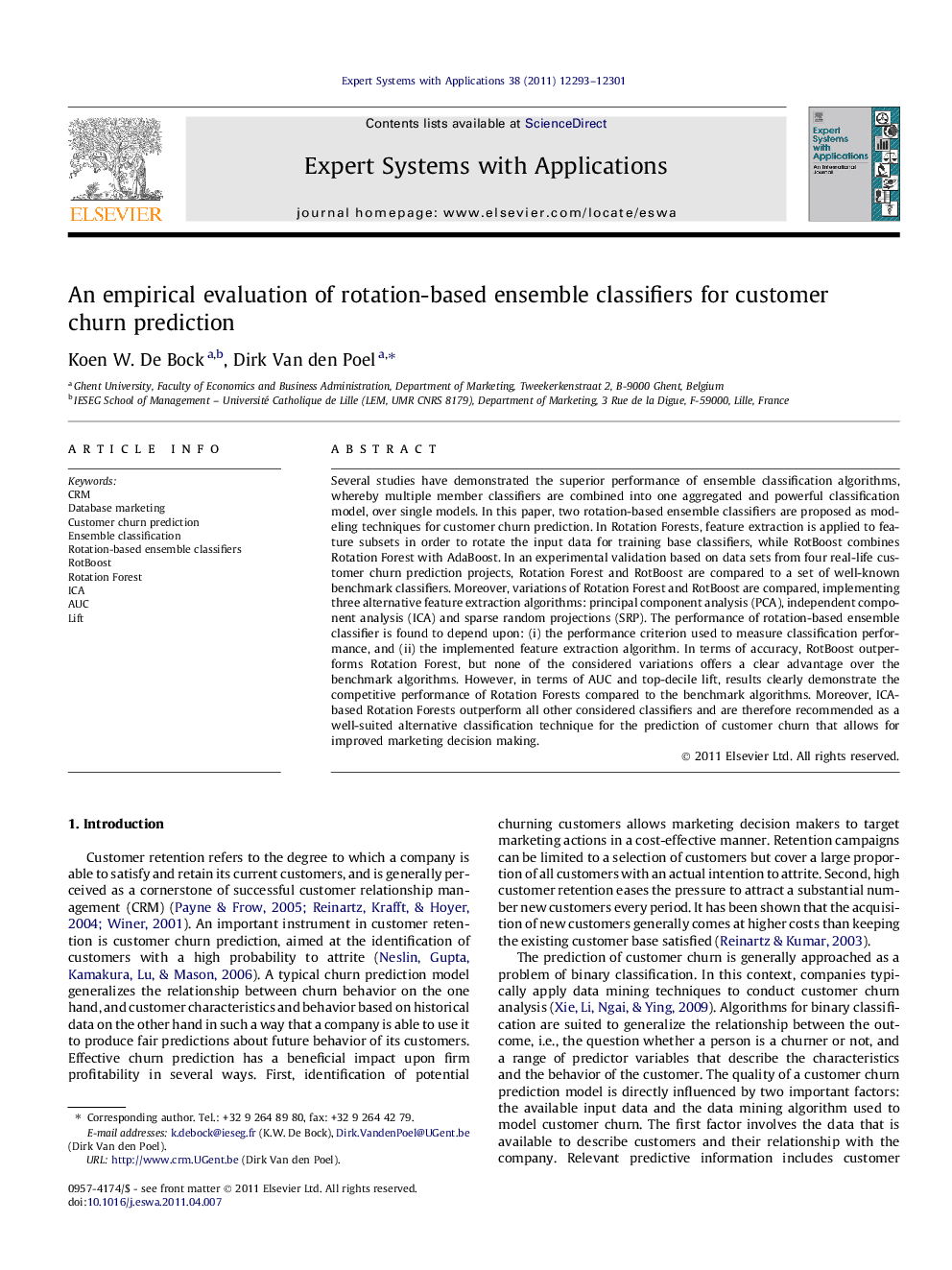
Several studies have demonstrated the superior performance of ensemble classification algorithms, whereby multiple member classifiers are combined into one aggregated and powerful classification model, over single models. In this paper, two rotation-based ensemble classifiers are proposed as modeling techniques for customer churn prediction. In Rotation Forests, feature extraction is applied to feature subsets in order to rotate the input data for training base classifiers, while RotBoost combines Rotation Forest with AdaBoost. In an experimental validation based on data sets from four real-life customer churn prediction projects, Rotation Forest and RotBoost are compared to a set of well-known benchmark classifiers. Moreover, variations of Rotation Forest and RotBoost are compared, implementing three alternative feature extraction algorithms: principal component analysis (PCA), independent component analysis (ICA) and sparse random projections (SRP). The performance of rotation-based ensemble classifier is found to depend upon: (i) the performance criterion used to measure classification performance, and (ii) the implemented feature extraction algorithm. In terms of accuracy, RotBoost outperforms Rotation Forest, but none of the considered variations offers a clear advantage over the benchmark algorithms. However, in terms of AUC and top-decile lift, results clearly demonstrate the competitive performance of Rotation Forests compared to the benchmark algorithms. Moreover, ICA-based Rotation Forests outperform all other considered classifiers and are therefore recommended as a well-suited alternative classification technique for the prediction of customer churn that allows for improved marketing decision making.
► In this article, two rotation-based ensemble classifiers (Rotation Forest and RotBoost) are applied to customer churn prediction.
► Several variations of both algorithms are compared and benchmarked.
► In terms of accuracy, RotBoost outperforms Rotation Forest but does not offer an advantage over the benchmark algorithms.
► In terms of AUC and top-decile lift, Rotation Forest based upon Independent Component Analysis (ICA) outperforms all other classifiers and is recommended as a sensible choice for customer churn prediction modeling.
Journal: Expert Systems with Applications - Volume 38, Issue 10, 15 September 2011, Pages 12293–12301