کد مقاله | کد نشریه | سال انتشار | مقاله انگلیسی | نسخه تمام متن |
---|---|---|---|---|
388648 | 660935 | 2010 | 10 صفحه PDF | دانلود رایگان |
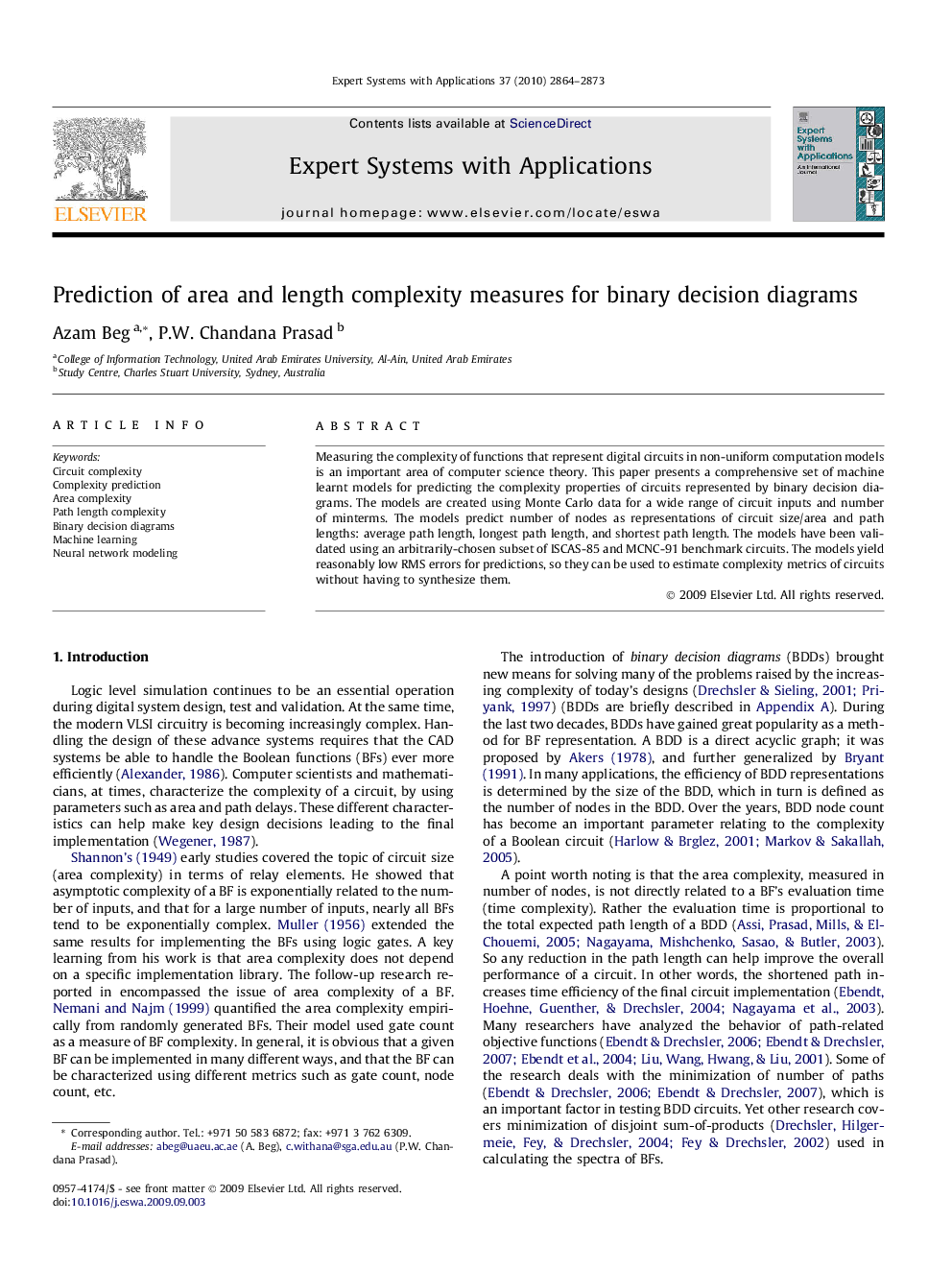
Measuring the complexity of functions that represent digital circuits in non-uniform computation models is an important area of computer science theory. This paper presents a comprehensive set of machine learnt models for predicting the complexity properties of circuits represented by binary decision diagrams. The models are created using Monte Carlo data for a wide range of circuit inputs and number of minterms. The models predict number of nodes as representations of circuit size/area and path lengths: average path length, longest path length, and shortest path length. The models have been validated using an arbitrarily-chosen subset of ISCAS-85 and MCNC-91 benchmark circuits. The models yield reasonably low RMS errors for predictions, so they can be used to estimate complexity metrics of circuits without having to synthesize them.
Journal: Expert Systems with Applications - Volume 37, Issue 4, April 2010, Pages 2864–2873