کد مقاله | کد نشریه | سال انتشار | مقاله انگلیسی | نسخه تمام متن |
---|---|---|---|---|
396995 | 670652 | 2012 | 9 صفحه PDF | دانلود رایگان |
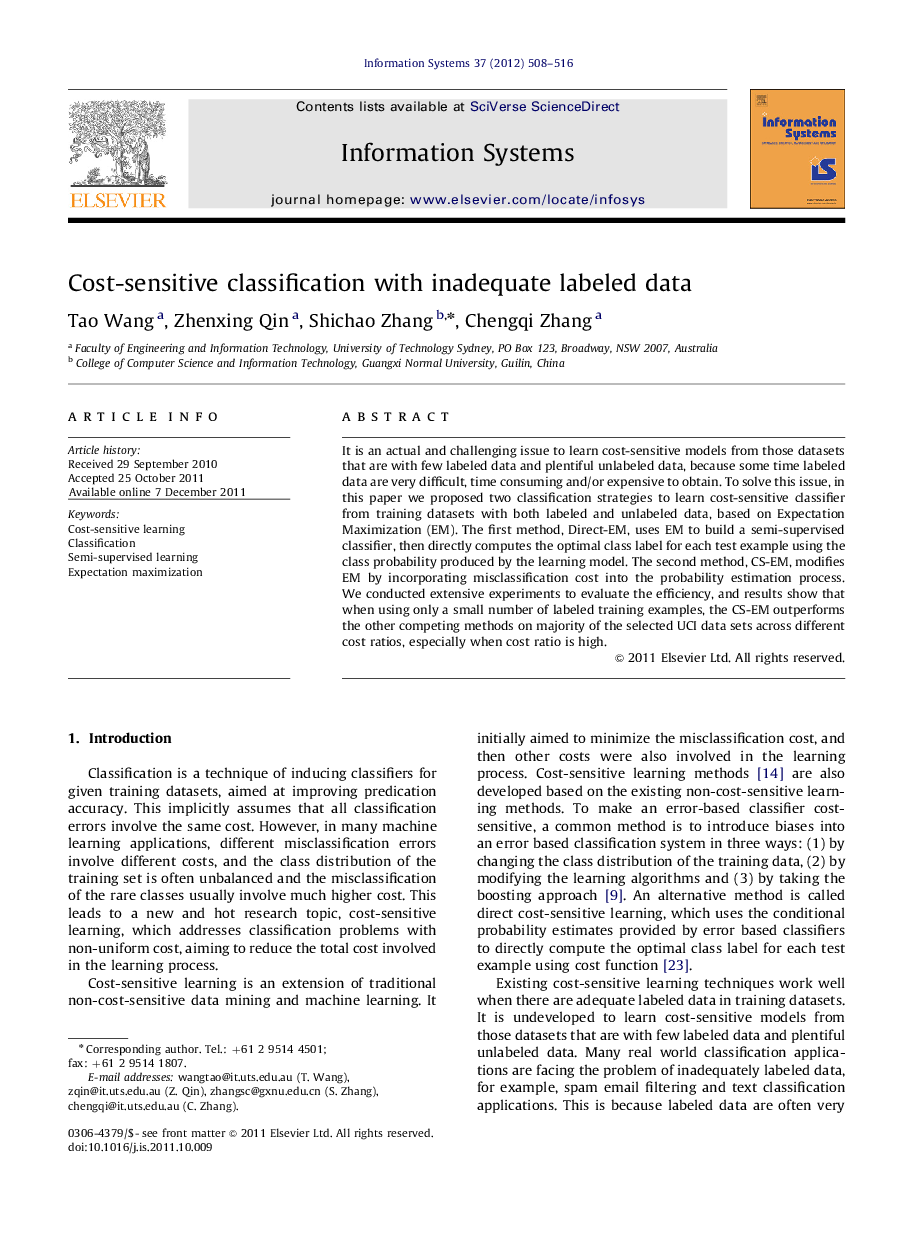
It is an actual and challenging issue to learn cost-sensitive models from those datasets that are with few labeled data and plentiful unlabeled data, because some time labeled data are very difficult, time consuming and/or expensive to obtain. To solve this issue, in this paper we proposed two classification strategies to learn cost-sensitive classifier from training datasets with both labeled and unlabeled data, based on Expectation Maximization (EM). The first method, Direct-EM, uses EM to build a semi-supervised classifier, then directly computes the optimal class label for each test example using the class probability produced by the learning model. The second method, CS-EM, modifies EM by incorporating misclassification cost into the probability estimation process. We conducted extensive experiments to evaluate the efficiency, and results show that when using only a small number of labeled training examples, the CS-EM outperforms the other competing methods on majority of the selected UCI data sets across different cost ratios, especially when cost ratio is high.
► Studying semi-supervised cost-sensitive learning from datasets with both labeled and unlabeled data.
► Designing a semi-supervised cost-sensitive classifier, Direct-EM.
► Proposing CS-EM method that modifies EM by incorporating misclassification cost into the probability estimation process.
Journal: Information Systems - Volume 37, Issue 5, July 2012, Pages 508–516