کد مقاله | کد نشریه | سال انتشار | مقاله انگلیسی | نسخه تمام متن |
---|---|---|---|---|
398404 | 1438722 | 2016 | 12 صفحه PDF | دانلود رایگان |
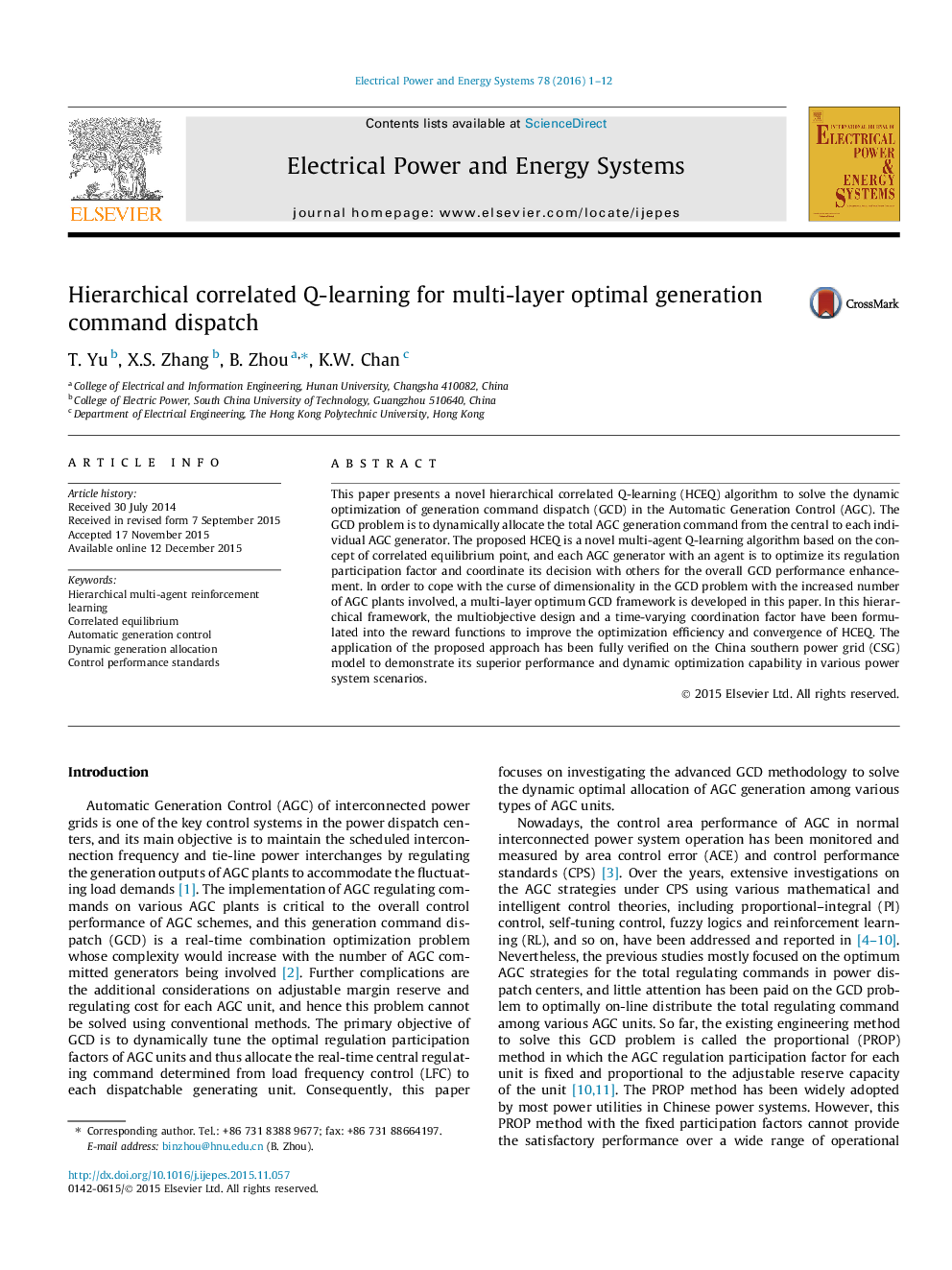
• A multi-layer framework is formulated for dynamic optimal generation allocation.
• A hierarchical correlated Q-learning is proposed for solving this problem.
• Proposed scheme can enhance the generation performance with less regulating costs.
This paper presents a novel hierarchical correlated Q-learning (HCEQ) algorithm to solve the dynamic optimization of generation command dispatch (GCD) in the Automatic Generation Control (AGC). The GCD problem is to dynamically allocate the total AGC generation command from the central to each individual AGC generator. The proposed HCEQ is a novel multi-agent Q-learning algorithm based on the concept of correlated equilibrium point, and each AGC generator with an agent is to optimize its regulation participation factor and coordinate its decision with others for the overall GCD performance enhancement. In order to cope with the curse of dimensionality in the GCD problem with the increased number of AGC plants involved, a multi-layer optimum GCD framework is developed in this paper. In this hierarchical framework, the multiobjective design and a time-varying coordination factor have been formulated into the reward functions to improve the optimization efficiency and convergence of HCEQ. The application of the proposed approach has been fully verified on the China southern power grid (CSG) model to demonstrate its superior performance and dynamic optimization capability in various power system scenarios.
Journal: International Journal of Electrical Power & Energy Systems - Volume 78, June 2016, Pages 1–12