کد مقاله | کد نشریه | سال انتشار | مقاله انگلیسی | نسخه تمام متن |
---|---|---|---|---|
399952 | 1438763 | 2012 | 7 صفحه PDF | دانلود رایگان |
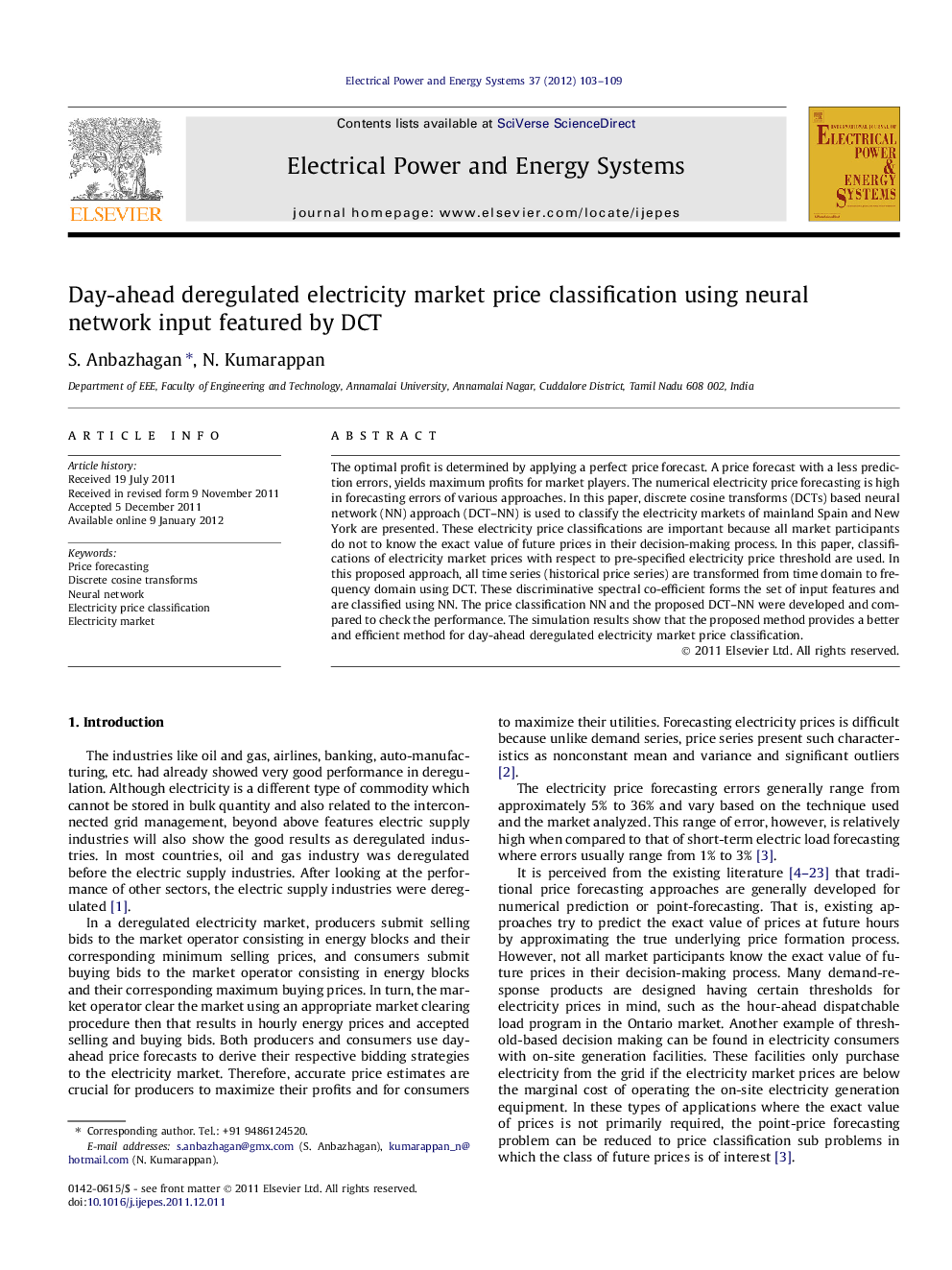
The optimal profit is determined by applying a perfect price forecast. A price forecast with a less prediction errors, yields maximum profits for market players. The numerical electricity price forecasting is high in forecasting errors of various approaches. In this paper, discrete cosine transforms (DCTs) based neural network (NN) approach (DCT–NN) is used to classify the electricity markets of mainland Spain and New York are presented. These electricity price classifications are important because all market participants do not to know the exact value of future prices in their decision-making process. In this paper, classifications of electricity market prices with respect to pre-specified electricity price threshold are used. In this proposed approach, all time series (historical price series) are transformed from time domain to frequency domain using DCT. These discriminative spectral co-efficient forms the set of input features and are classified using NN. The price classification NN and the proposed DCT–NN were developed and compared to check the performance. The simulation results show that the proposed method provides a better and efficient method for day-ahead deregulated electricity market price classification.
► We presented DCT–NN model for price classification in Spain and New York markets.
► The key factors impacting electricity price classification are historical prices.
► Past 42 days were trained and the next 7 days were classified.
► The proposed approach has a simple and better neural network (NN) structure.
► DCT–NN is a good tool for price classification in terms of efficiency compared to NN.
Journal: International Journal of Electrical Power & Energy Systems - Volume 37, Issue 1, May 2012, Pages 103–109