کد مقاله | کد نشریه | سال انتشار | مقاله انگلیسی | نسخه تمام متن |
---|---|---|---|---|
402646 | 676973 | 2015 | 9 صفحه PDF | دانلود رایگان |
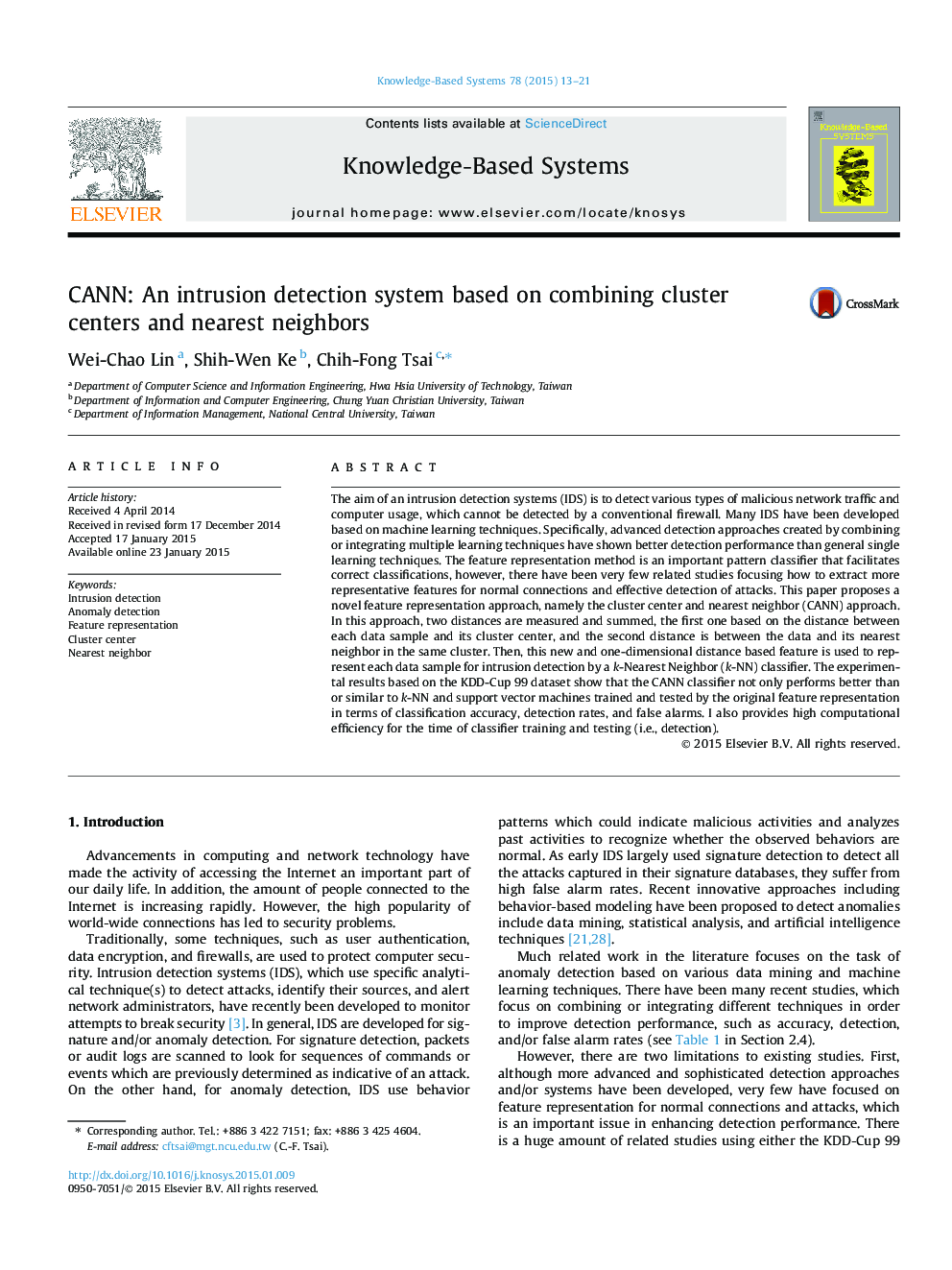
The aim of an intrusion detection systems (IDS) is to detect various types of malicious network traffic and computer usage, which cannot be detected by a conventional firewall. Many IDS have been developed based on machine learning techniques. Specifically, advanced detection approaches created by combining or integrating multiple learning techniques have shown better detection performance than general single learning techniques. The feature representation method is an important pattern classifier that facilitates correct classifications, however, there have been very few related studies focusing how to extract more representative features for normal connections and effective detection of attacks. This paper proposes a novel feature representation approach, namely the cluster center and nearest neighbor (CANN) approach. In this approach, two distances are measured and summed, the first one based on the distance between each data sample and its cluster center, and the second distance is between the data and its nearest neighbor in the same cluster. Then, this new and one-dimensional distance based feature is used to represent each data sample for intrusion detection by a k-Nearest Neighbor (k-NN) classifier. The experimental results based on the KDD-Cup 99 dataset show that the CANN classifier not only performs better than or similar to k-NN and support vector machines trained and tested by the original feature representation in terms of classification accuracy, detection rates, and false alarms. I also provides high computational efficiency for the time of classifier training and testing (i.e., detection).
Journal: Knowledge-Based Systems - Volume 78, April 2015, Pages 13–21