کد مقاله | کد نشریه | سال انتشار | مقاله انگلیسی | نسخه تمام متن |
---|---|---|---|---|
402736 | 676993 | 2013 | 13 صفحه PDF | دانلود رایگان |
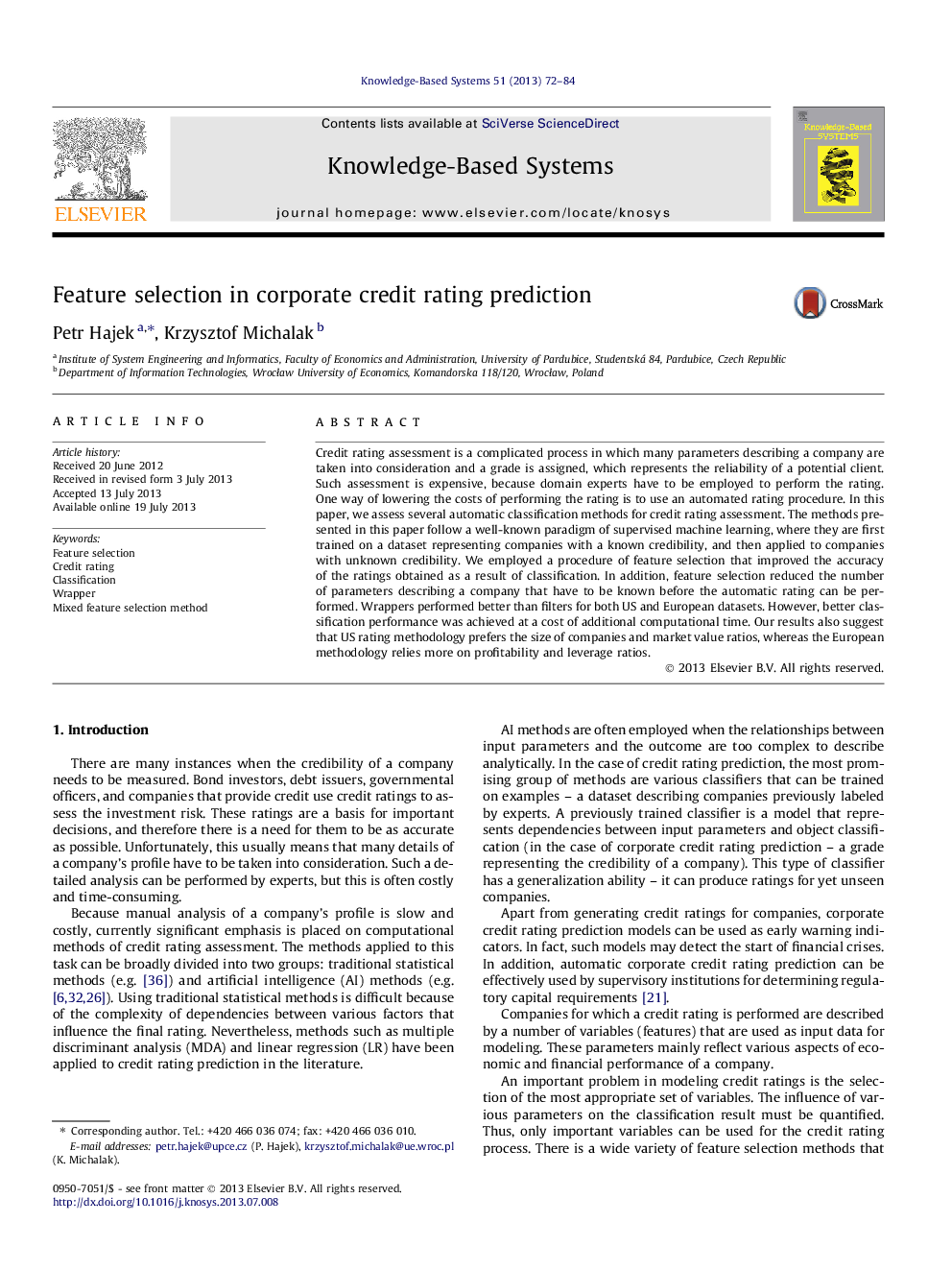
• We employ feature selection for corporate credit rating modeling.
• Feature selection procedure improves the classification accuracy of the credit ratings.
• The number of features required for credit rating classification can be significantly reduced.
Credit rating assessment is a complicated process in which many parameters describing a company are taken into consideration and a grade is assigned, which represents the reliability of a potential client. Such assessment is expensive, because domain experts have to be employed to perform the rating. One way of lowering the costs of performing the rating is to use an automated rating procedure. In this paper, we assess several automatic classification methods for credit rating assessment. The methods presented in this paper follow a well-known paradigm of supervised machine learning, where they are first trained on a dataset representing companies with a known credibility, and then applied to companies with unknown credibility. We employed a procedure of feature selection that improved the accuracy of the ratings obtained as a result of classification. In addition, feature selection reduced the number of parameters describing a company that have to be known before the automatic rating can be performed. Wrappers performed better than filters for both US and European datasets. However, better classification performance was achieved at a cost of additional computational time. Our results also suggest that US rating methodology prefers the size of companies and market value ratios, whereas the European methodology relies more on profitability and leverage ratios.
Journal: Knowledge-Based Systems - Volume 51, October 2013, Pages 72–84