کد مقاله | کد نشریه | سال انتشار | مقاله انگلیسی | نسخه تمام متن |
---|---|---|---|---|
4034307 | 1263446 | 2011 | 6 صفحه PDF | دانلود رایگان |
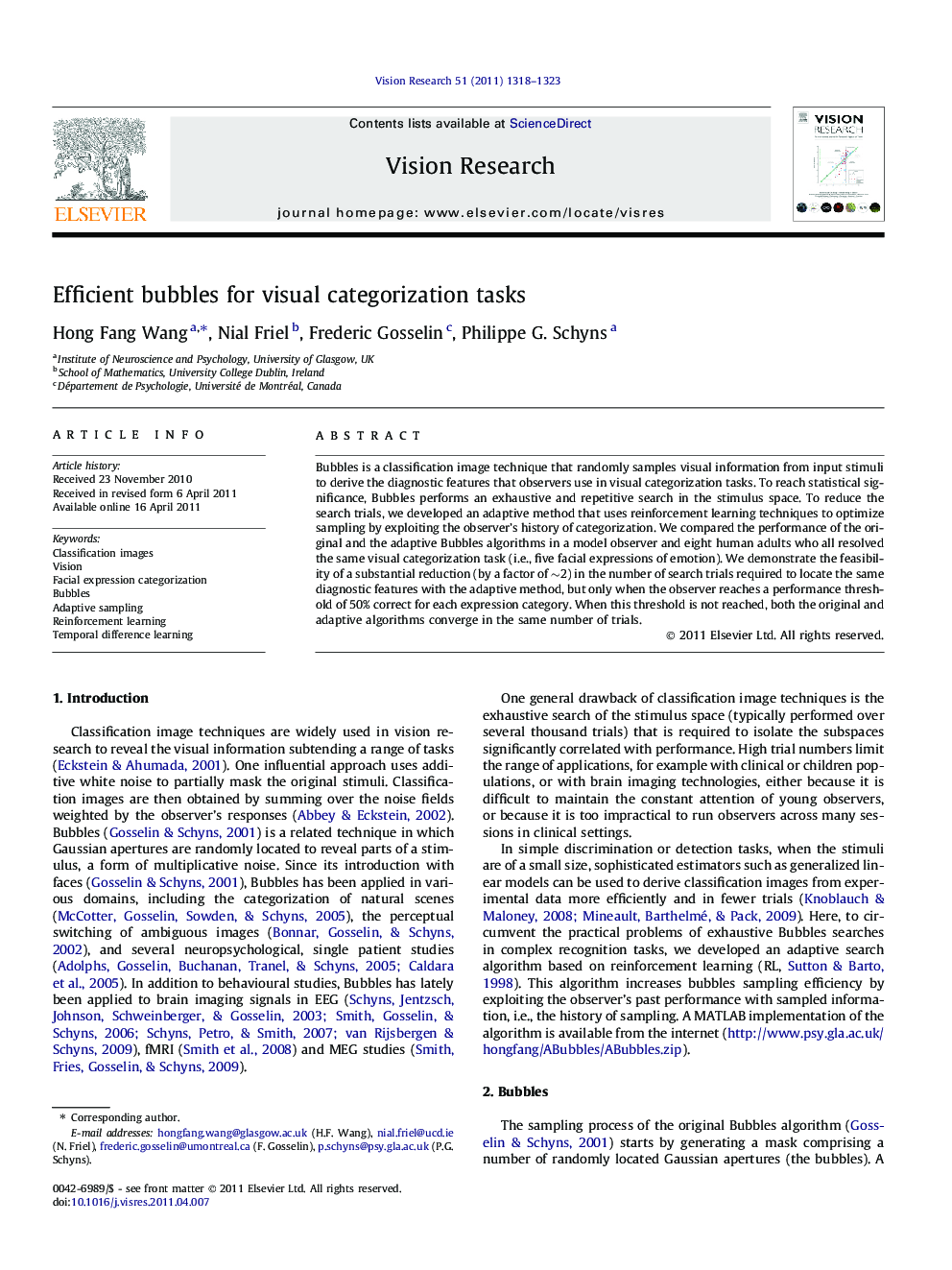
Bubbles is a classification image technique that randomly samples visual information from input stimuli to derive the diagnostic features that observers use in visual categorization tasks. To reach statistical significance, Bubbles performs an exhaustive and repetitive search in the stimulus space. To reduce the search trials, we developed an adaptive method that uses reinforcement learning techniques to optimize sampling by exploiting the observer’s history of categorization. We compared the performance of the original and the adaptive Bubbles algorithms in a model observer and eight human adults who all resolved the same visual categorization task (i.e., five facial expressions of emotion). We demonstrate the feasibility of a substantial reduction (by a factor of ∼2) in the number of search trials required to locate the same diagnostic features with the adaptive method, but only when the observer reaches a performance threshold of 50% correct for each expression category. When this threshold is not reached, both the original and adaptive algorithms converge in the same number of trials.
Research highlights
► We improved the performance of Bubbles by applying reinforcement learning techniques.
► The development has been applied to one model and 8 human observers with a face categorization task.
► Analyses of the new algorithm’s performance are provided.
► Reduction of experimental trials also depends on observer’s response accuracy.
Journal: Vision Research - Volume 51, Issue 12, 21 June 2011, Pages 1318–1323