کد مقاله | کد نشریه | سال انتشار | مقاله انگلیسی | نسخه تمام متن |
---|---|---|---|---|
403448 | 677231 | 2016 | 11 صفحه PDF | دانلود رایگان |
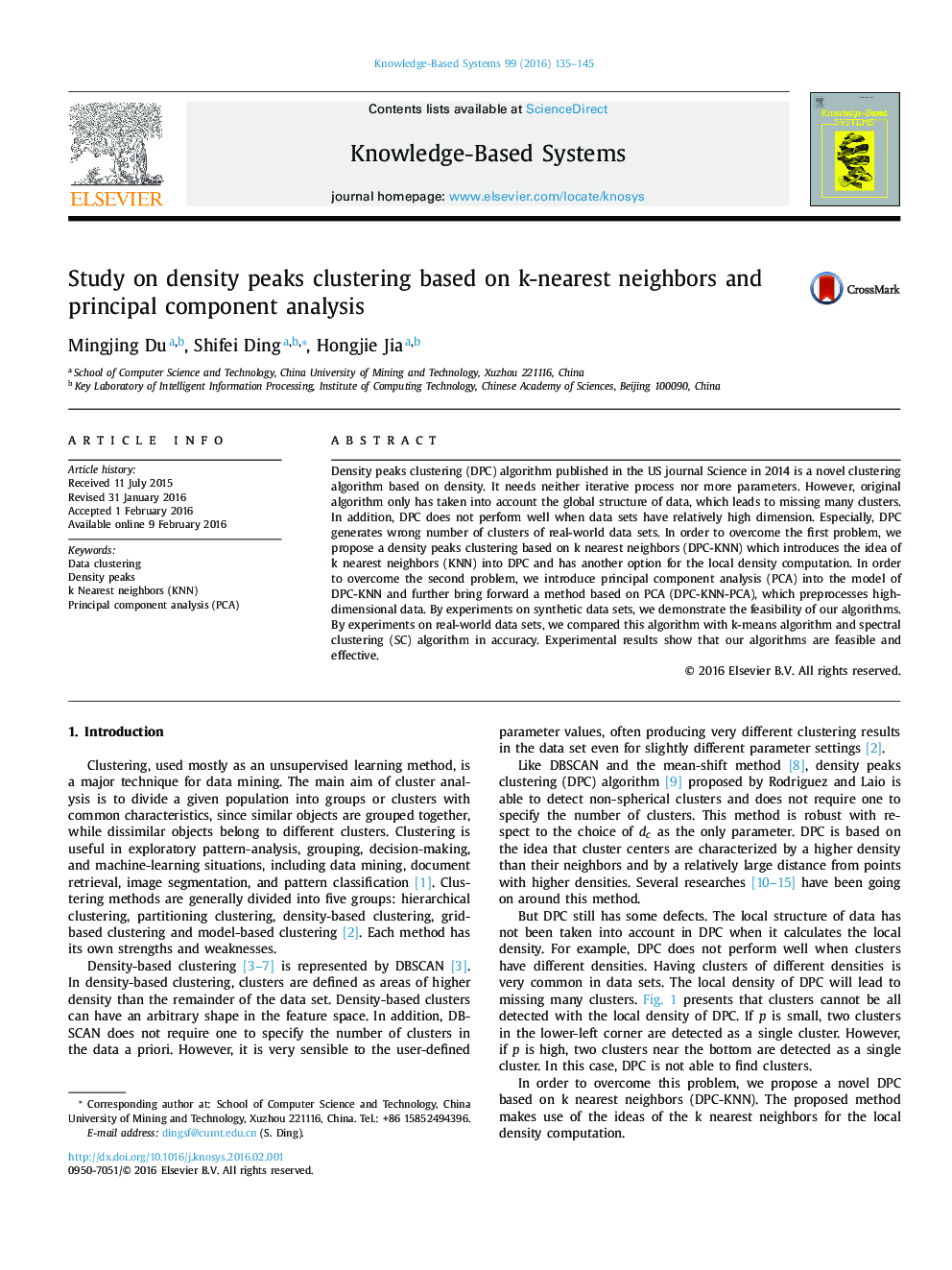
Density peaks clustering (DPC) algorithm published in the US journal Science in 2014 is a novel clustering algorithm based on density. It needs neither iterative process nor more parameters. However, original algorithm only has taken into account the global structure of data, which leads to missing many clusters. In addition, DPC does not perform well when data sets have relatively high dimension. Especially, DPC generates wrong number of clusters of real-world data sets. In order to overcome the first problem, we propose a density peaks clustering based on k nearest neighbors (DPC-KNN) which introduces the idea of k nearest neighbors (KNN) into DPC and has another option for the local density computation. In order to overcome the second problem, we introduce principal component analysis (PCA) into the model of DPC-KNN and further bring forward a method based on PCA (DPC-KNN-PCA), which preprocesses high-dimensional data. By experiments on synthetic data sets, we demonstrate the feasibility of our algorithms. By experiments on real-world data sets, we compared this algorithm with k-means algorithm and spectral clustering (SC) algorithm in accuracy. Experimental results show that our algorithms are feasible and effective.
Journal: Knowledge-Based Systems - Volume 99, 1 May 2016, Pages 135–145