کد مقاله | کد نشریه | سال انتشار | مقاله انگلیسی | نسخه تمام متن |
---|---|---|---|---|
403755 | 677327 | 2012 | 14 صفحه PDF | دانلود رایگان |
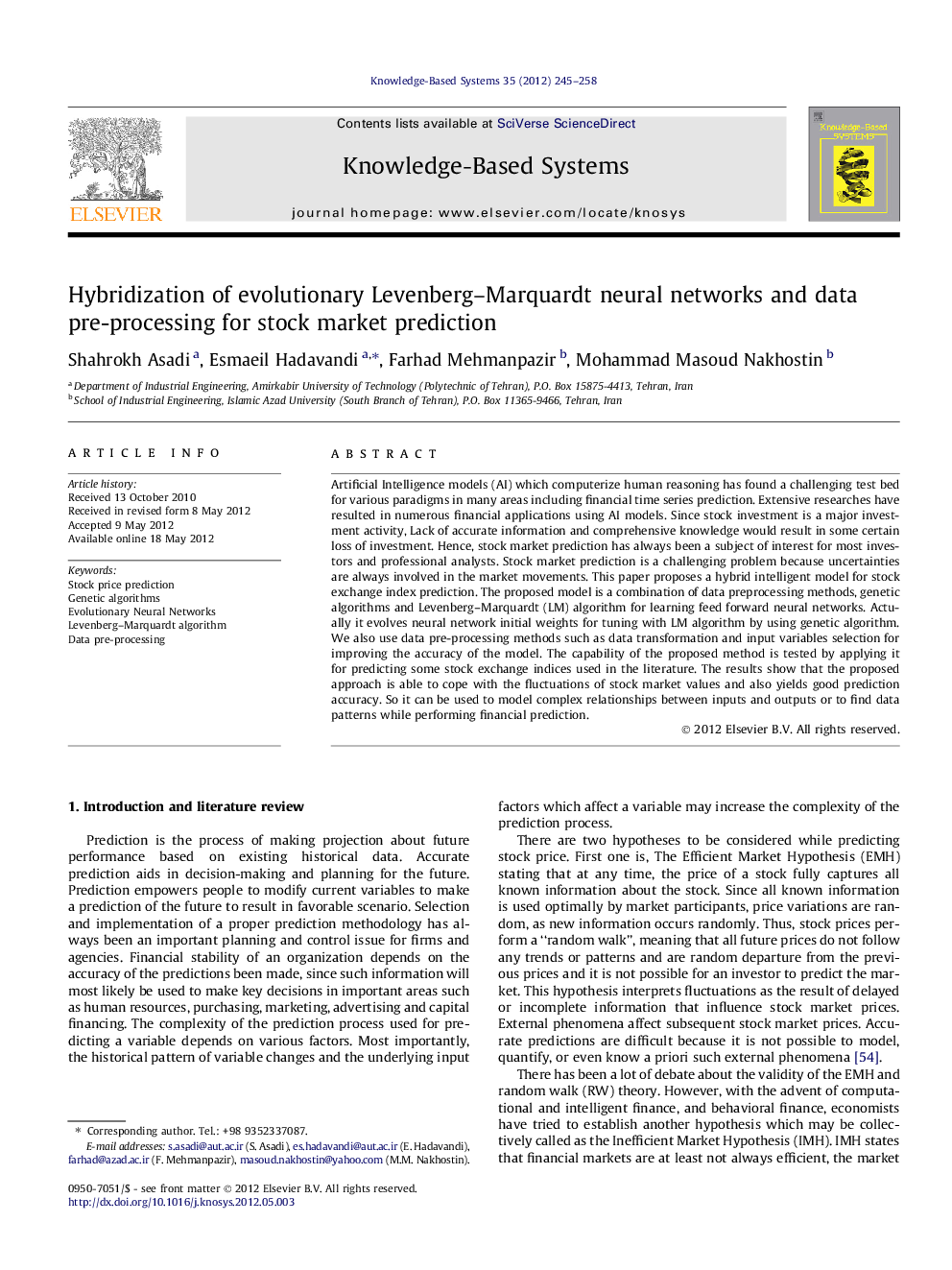
Artificial Intelligence models (AI) which computerize human reasoning has found a challenging test bed for various paradigms in many areas including financial time series prediction. Extensive researches have resulted in numerous financial applications using AI models. Since stock investment is a major investment activity, Lack of accurate information and comprehensive knowledge would result in some certain loss of investment. Hence, stock market prediction has always been a subject of interest for most investors and professional analysts. Stock market prediction is a challenging problem because uncertainties are always involved in the market movements. This paper proposes a hybrid intelligent model for stock exchange index prediction. The proposed model is a combination of data preprocessing methods, genetic algorithms and Levenberg–Marquardt (LM) algorithm for learning feed forward neural networks. Actually it evolves neural network initial weights for tuning with LM algorithm by using genetic algorithm. We also use data pre-processing methods such as data transformation and input variables selection for improving the accuracy of the model. The capability of the proposed method is tested by applying it for predicting some stock exchange indices used in the literature. The results show that the proposed approach is able to cope with the fluctuations of stock market values and also yields good prediction accuracy. So it can be used to model complex relationships between inputs and outputs or to find data patterns while performing financial prediction.
Journal: Knowledge-Based Systems - Volume 35, November 2012, Pages 245–258