کد مقاله | کد نشریه | سال انتشار | مقاله انگلیسی | نسخه تمام متن |
---|---|---|---|---|
404885 | 677461 | 2007 | 11 صفحه PDF | دانلود رایگان |
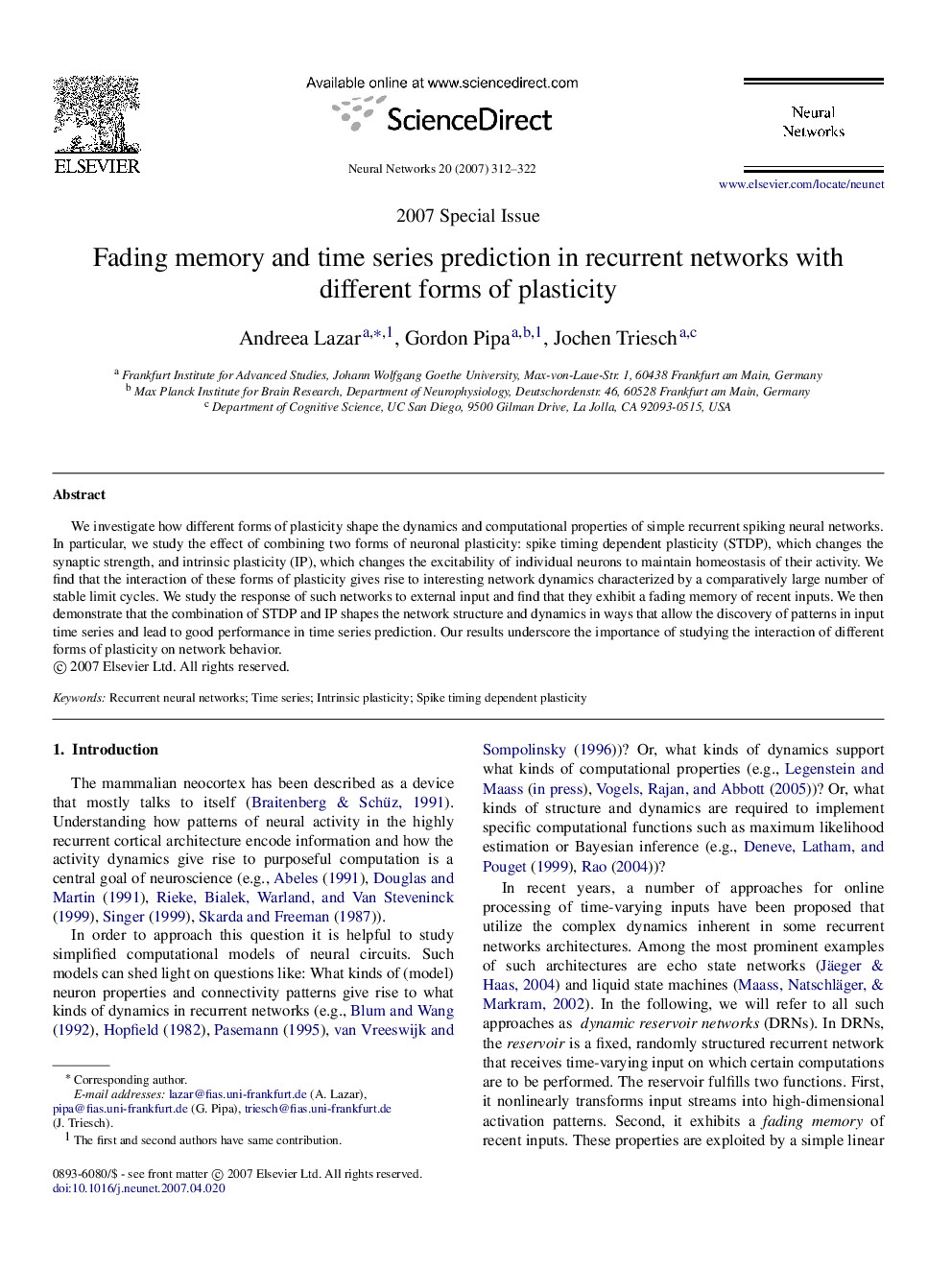
We investigate how different forms of plasticity shape the dynamics and computational properties of simple recurrent spiking neural networks. In particular, we study the effect of combining two forms of neuronal plasticity: spike timing dependent plasticity (STDP), which changes the synaptic strength, and intrinsic plasticity (IP), which changes the excitability of individual neurons to maintain homeostasis of their activity. We find that the interaction of these forms of plasticity gives rise to interesting network dynamics characterized by a comparatively large number of stable limit cycles. We study the response of such networks to external input and find that they exhibit a fading memory of recent inputs. We then demonstrate that the combination of STDP and IP shapes the network structure and dynamics in ways that allow the discovery of patterns in input time series and lead to good performance in time series prediction. Our results underscore the importance of studying the interaction of different forms of plasticity on network behavior.
Journal: Neural Networks - Volume 20, Issue 3, April 2007, Pages 312–322