کد مقاله | کد نشریه | سال انتشار | مقاله انگلیسی | نسخه تمام متن |
---|---|---|---|---|
405028 | 677474 | 2014 | 10 صفحه PDF | دانلود رایگان |
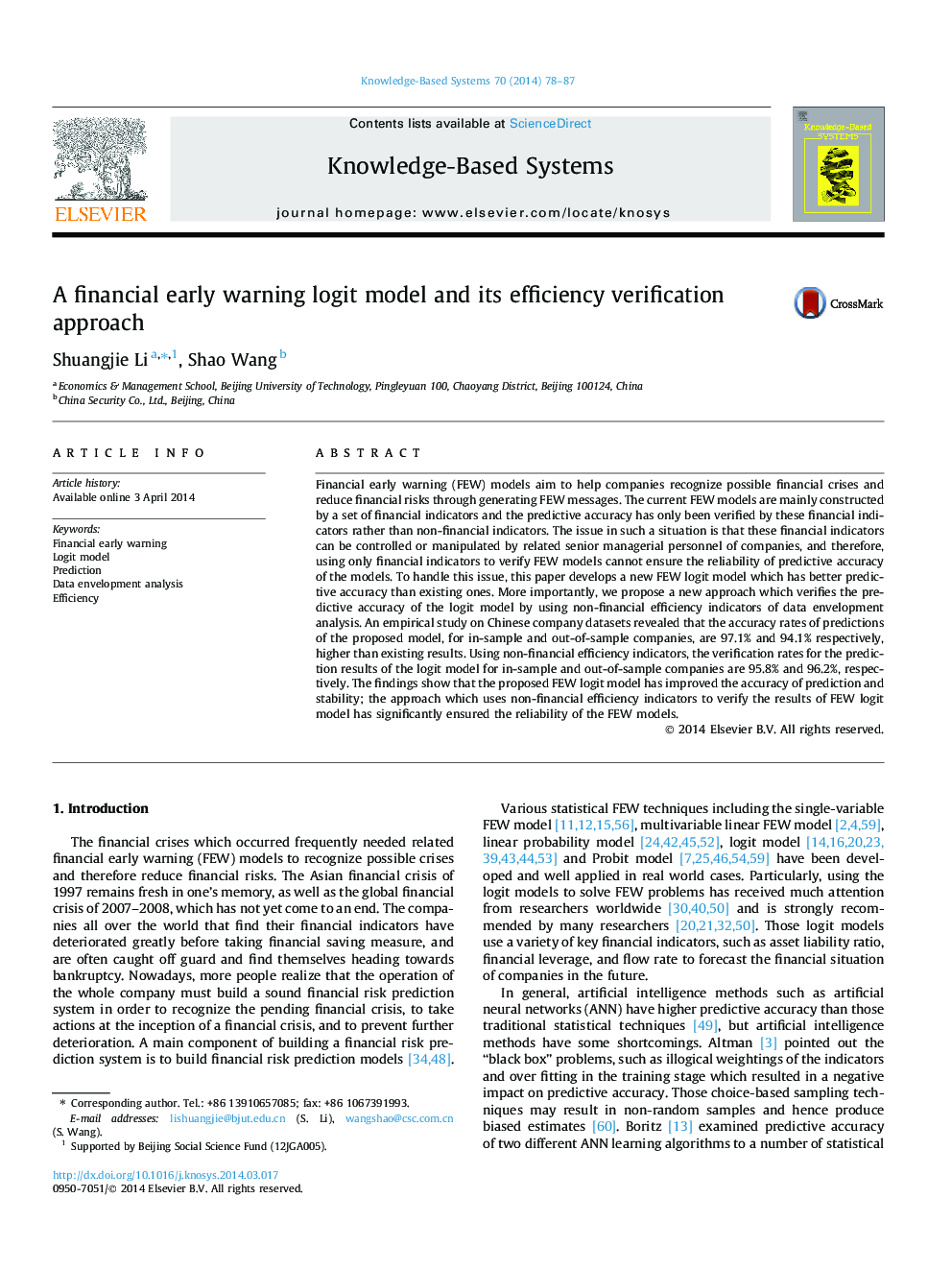
Financial early warning (FEW) models aim to help companies recognize possible financial crises and reduce financial risks through generating FEW messages. The current FEW models are mainly constructed by a set of financial indicators and the predictive accuracy has only been verified by these financial indicators rather than non-financial indicators. The issue in such a situation is that these financial indicators can be controlled or manipulated by related senior managerial personnel of companies, and therefore, using only financial indicators to verify FEW models cannot ensure the reliability of predictive accuracy of the models. To handle this issue, this paper develops a new FEW logit model which has better predictive accuracy than existing ones. More importantly, we propose a new approach which verifies the predictive accuracy of the logit model by using non-financial efficiency indicators of data envelopment analysis. An empirical study on Chinese company datasets revealed that the accuracy rates of predictions of the proposed model, for in-sample and out-of-sample companies, are 97.1% and 94.1% respectively, higher than existing results. Using non-financial efficiency indicators, the verification rates for the prediction results of the logit model for in-sample and out-of-sample companies are 95.8% and 96.2%, respectively. The findings show that the proposed FEW logit model has improved the accuracy of prediction and stability; the approach which uses non-financial efficiency indicators to verify the results of FEW logit model has significantly ensured the reliability of the FEW models.
Journal: Knowledge-Based Systems - Volume 70, November 2014, Pages 78–87