کد مقاله | کد نشریه | سال انتشار | مقاله انگلیسی | نسخه تمام متن |
---|---|---|---|---|
405031 | 677474 | 2014 | 15 صفحه PDF | دانلود رایگان |
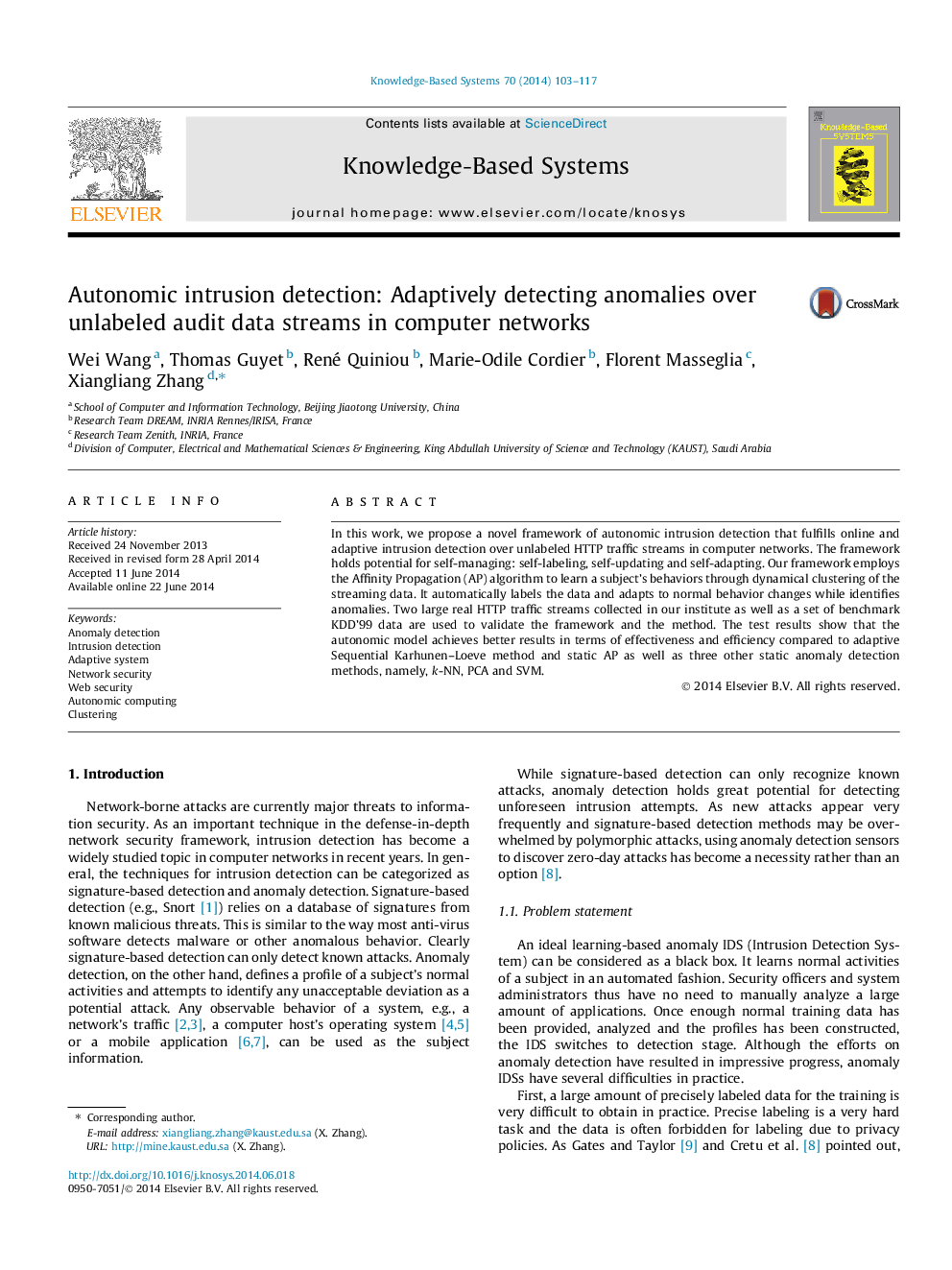
In this work, we propose a novel framework of autonomic intrusion detection that fulfills online and adaptive intrusion detection over unlabeled HTTP traffic streams in computer networks. The framework holds potential for self-managing: self-labeling, self-updating and self-adapting. Our framework employs the Affinity Propagation (AP) algorithm to learn a subject’s behaviors through dynamical clustering of the streaming data. It automatically labels the data and adapts to normal behavior changes while identifies anomalies. Two large real HTTP traffic streams collected in our institute as well as a set of benchmark KDD’99 data are used to validate the framework and the method. The test results show that the autonomic model achieves better results in terms of effectiveness and efficiency compared to adaptive Sequential Karhunen–Loeve method and static AP as well as three other static anomaly detection methods, namely, k-NN, PCA and SVM.
Journal: Knowledge-Based Systems - Volume 70, November 2014, Pages 103–117