کد مقاله | کد نشریه | سال انتشار | مقاله انگلیسی | نسخه تمام متن |
---|---|---|---|---|
405172 | 677499 | 2013 | 10 صفحه PDF | دانلود رایگان |
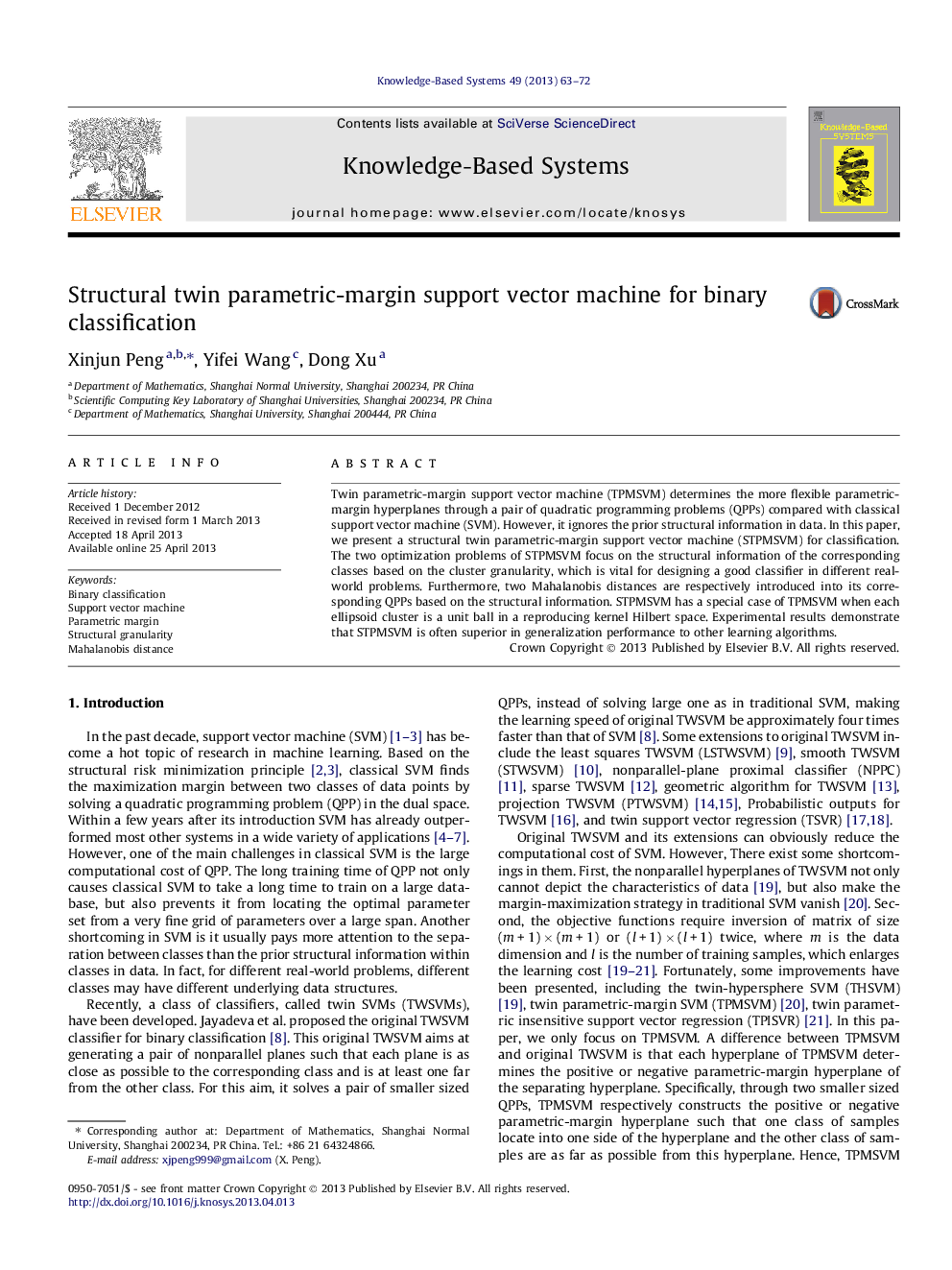
• A structural twin parametric-margin support vector machine (STPMSVM) classifier is presented.
• The structural information of corresponding classes based on cluster granularity is embedded into the optimization problems of STPMSVM.
• Two related Mahalanobis distances are respectively introduced into its corresponding QPPs based on structural information.
• STPMSVM degenerates into TPMSVM when each ellipsoid cluster is a unit ball in a reproducing kernel Hilbert space.
• STPMSVM is often superior in generalization performance to other learning algorithms.
Twin parametric-margin support vector machine (TPMSVM) determines the more flexible parametric-margin hyperplanes through a pair of quadratic programming problems (QPPs) compared with classical support vector machine (SVM). However, it ignores the prior structural information in data. In this paper, we present a structural twin parametric-margin support vector machine (STPMSVM) for classification. The two optimization problems of STPMSVM focus on the structural information of the corresponding classes based on the cluster granularity, which is vital for designing a good classifier in different real-world problems. Furthermore, two Mahalanobis distances are respectively introduced into its corresponding QPPs based on the structural information. STPMSVM has a special case of TPMSVM when each ellipsoid cluster is a unit ball in a reproducing kernel Hilbert space. Experimental results demonstrate that STPMSVM is often superior in generalization performance to other learning algorithms.
Journal: Knowledge-Based Systems - Volume 49, September 2013, Pages 63–72