کد مقاله | کد نشریه | سال انتشار | مقاله انگلیسی | نسخه تمام متن |
---|---|---|---|---|
405257 | 677516 | 2012 | 8 صفحه PDF | دانلود رایگان |
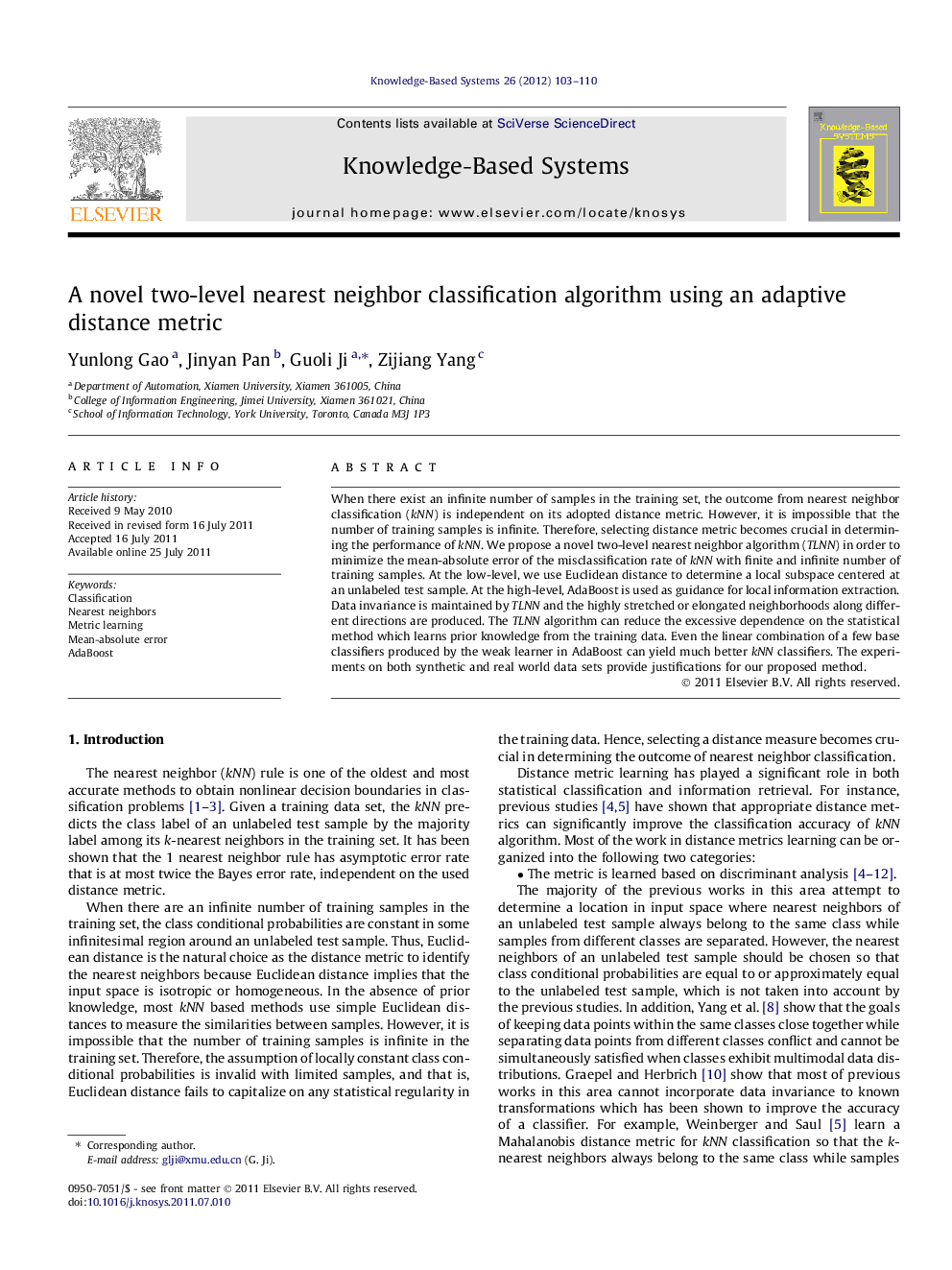
When there exist an infinite number of samples in the training set, the outcome from nearest neighbor classification (kNN) is independent on its adopted distance metric. However, it is impossible that the number of training samples is infinite. Therefore, selecting distance metric becomes crucial in determining the performance of kNN. We propose a novel two-level nearest neighbor algorithm (TLNN) in order to minimize the mean-absolute error of the misclassification rate of kNN with finite and infinite number of training samples. At the low-level, we use Euclidean distance to determine a local subspace centered at an unlabeled test sample. At the high-level, AdaBoost is used as guidance for local information extraction. Data invariance is maintained by TLNN and the highly stretched or elongated neighborhoods along different directions are produced. The TLNN algorithm can reduce the excessive dependence on the statistical method which learns prior knowledge from the training data. Even the linear combination of a few base classifiers produced by the weak learner in AdaBoost can yield much better kNN classifiers. The experiments on both synthetic and real world data sets provide justifications for our proposed method.
Journal: Knowledge-Based Systems - Volume 26, February 2012, Pages 103–110