کد مقاله | کد نشریه | سال انتشار | مقاله انگلیسی | نسخه تمام متن |
---|---|---|---|---|
405558 | 677671 | 2011 | 11 صفحه PDF | دانلود رایگان |
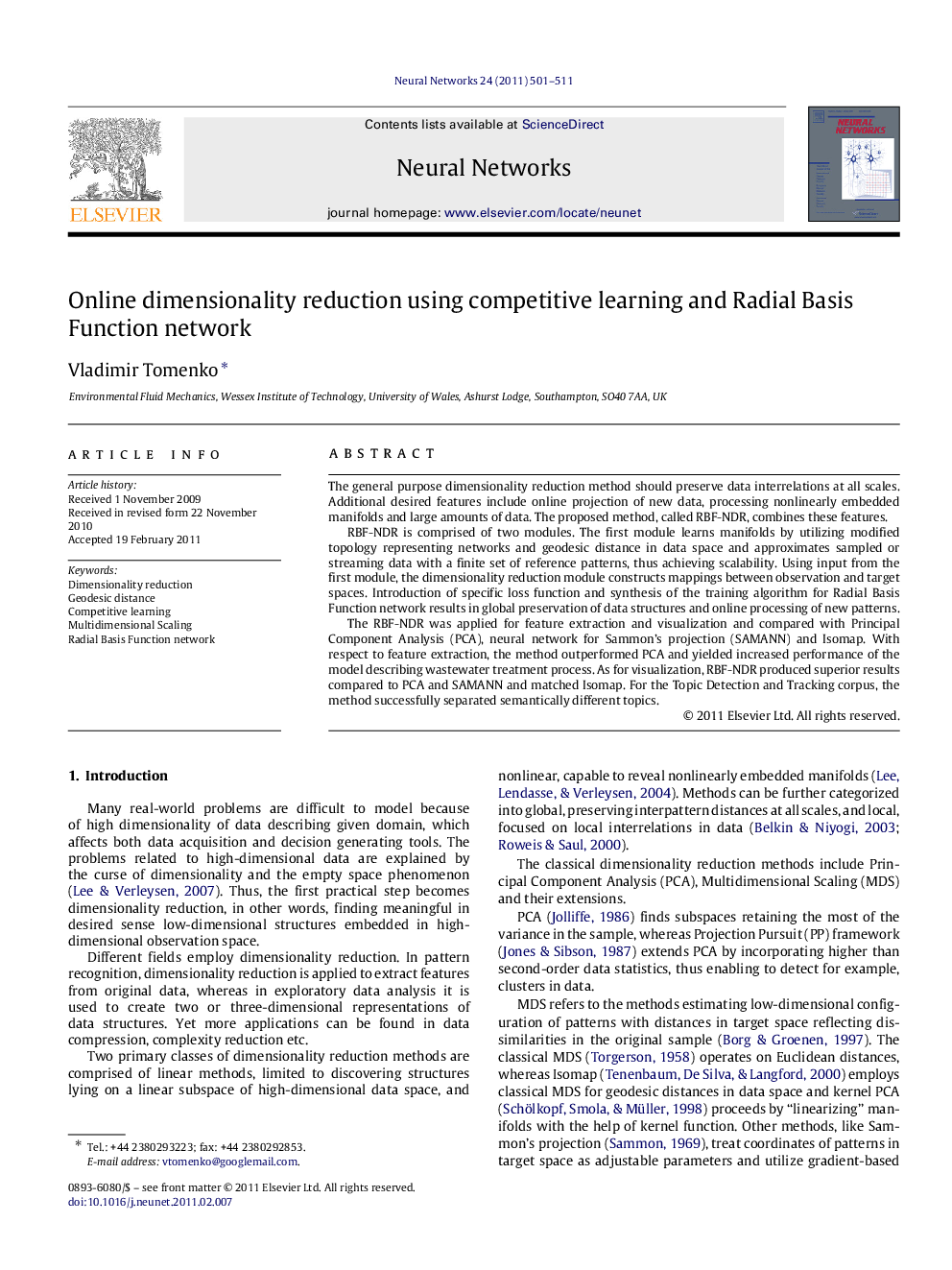
The general purpose dimensionality reduction method should preserve data interrelations at all scales. Additional desired features include online projection of new data, processing nonlinearly embedded manifolds and large amounts of data. The proposed method, called RBF-NDR, combines these features.RBF-NDR is comprised of two modules. The first module learns manifolds by utilizing modified topology representing networks and geodesic distance in data space and approximates sampled or streaming data with a finite set of reference patterns, thus achieving scalability. Using input from the first module, the dimensionality reduction module constructs mappings between observation and target spaces. Introduction of specific loss function and synthesis of the training algorithm for Radial Basis Function network results in global preservation of data structures and online processing of new patterns.The RBF-NDR was applied for feature extraction and visualization and compared with Principal Component Analysis (PCA), neural network for Sammon’s projection (SAMANN) and Isomap. With respect to feature extraction, the method outperformed PCA and yielded increased performance of the model describing wastewater treatment process. As for visualization, RBF-NDR produced superior results compared to PCA and SAMANN and matched Isomap. For the Topic Detection and Tracking corpus, the method successfully separated semantically different topics.
Journal: Neural Networks - Volume 24, Issue 5, June 2011, Pages 501–511