کد مقاله | کد نشریه | سال انتشار | مقاله انگلیسی | نسخه تمام متن |
---|---|---|---|---|
405810 | 678034 | 2016 | 16 صفحه PDF | دانلود رایگان |
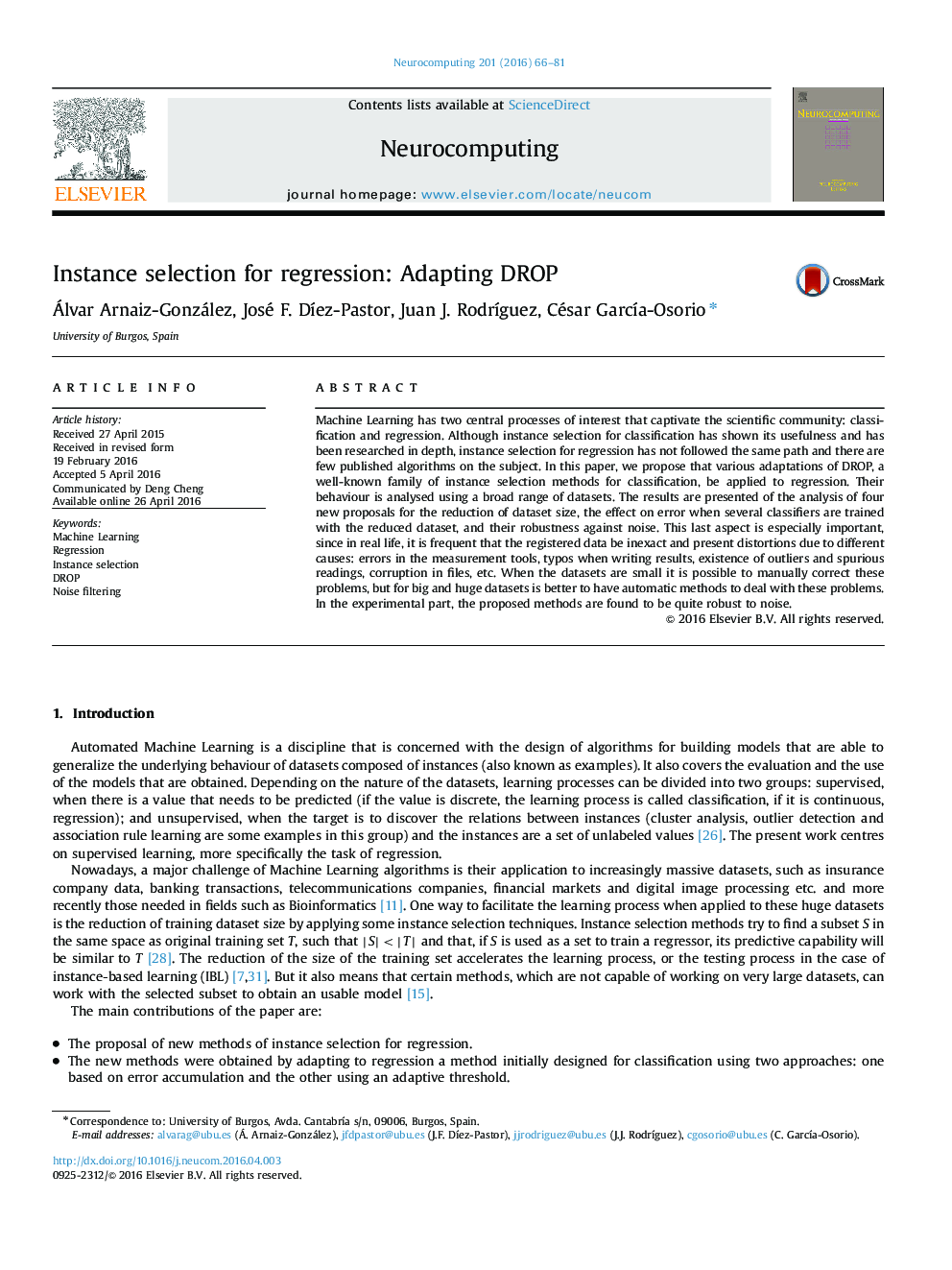
Machine Learning has two central processes of interest that captivate the scientific community: classification and regression. Although instance selection for classification has shown its usefulness and has been researched in depth, instance selection for regression has not followed the same path and there are few published algorithms on the subject. In this paper, we propose that various adaptations of DROP, a well-known family of instance selection methods for classification, be applied to regression. Their behaviour is analysed using a broad range of datasets. The results are presented of the analysis of four new proposals for the reduction of dataset size, the effect on error when several classifiers are trained with the reduced dataset, and their robustness against noise. This last aspect is especially important, since in real life, it is frequent that the registered data be inexact and present distortions due to different causes: errors in the measurement tools, typos when writing results, existence of outliers and spurious readings, corruption in files, etc. When the datasets are small it is possible to manually correct these problems, but for big and huge datasets is better to have automatic methods to deal with these problems. In the experimental part, the proposed methods are found to be quite robust to noise.
Journal: Neurocomputing - Volume 201, 12 August 2016, Pages 66–81