کد مقاله | کد نشریه | سال انتشار | مقاله انگلیسی | نسخه تمام متن |
---|---|---|---|---|
405940 | 678050 | 2016 | 12 صفحه PDF | دانلود رایگان |
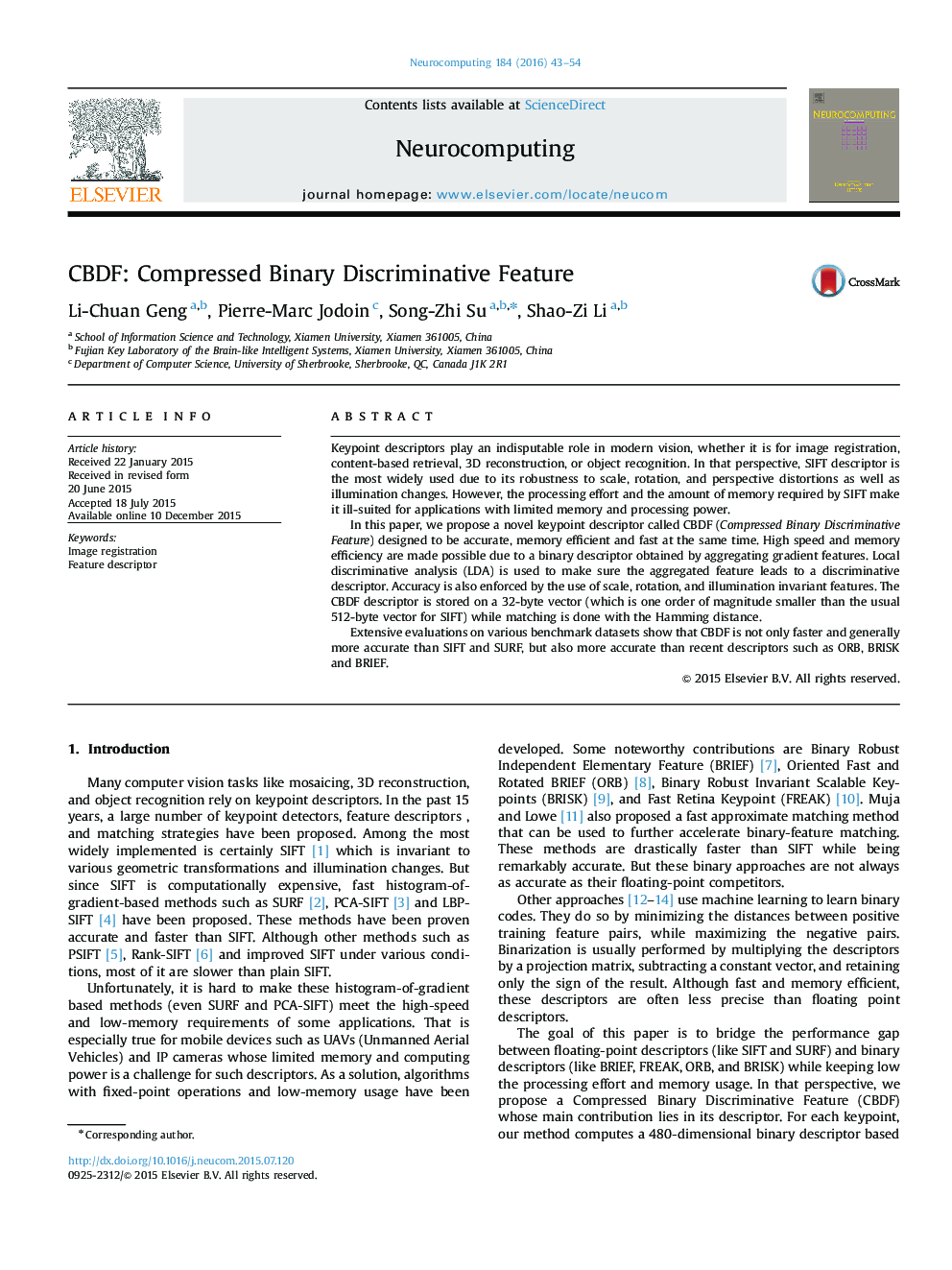
Keypoint descriptors play an indisputable role in modern vision, whether it is for image registration, content-based retrieval, 3D reconstruction, or object recognition. In that perspective, SIFT descriptor is the most widely used due to its robustness to scale, rotation, and perspective distortions as well as illumination changes. However, the processing effort and the amount of memory required by SIFT make it ill-suited for applications with limited memory and processing power.In this paper, we propose a novel keypoint descriptor called CBDF (Compressed Binary Discriminative Feature) designed to be accurate, memory efficient and fast at the same time. High speed and memory efficiency are made possible due to a binary descriptor obtained by aggregating gradient features. Local discriminative analysis (LDA) is used to make sure the aggregated feature leads to a discriminative descriptor. Accuracy is also enforced by the use of scale, rotation, and illumination invariant features. The CBDF descriptor is stored on a 32-byte vector (which is one order of magnitude smaller than the usual 512-byte vector for SIFT) while matching is done with the Hamming distance.Extensive evaluations on various benchmark datasets show that CBDF is not only faster and generally more accurate than SIFT and SURF, but also more accurate than recent descriptors such as ORB, BRISK and BRIEF.
Journal: Neurocomputing - Volume 184, 5 April 2016, Pages 43–54