کد مقاله | کد نشریه | سال انتشار | مقاله انگلیسی | نسخه تمام متن |
---|---|---|---|---|
407350 | 678138 | 2013 | 11 صفحه PDF | دانلود رایگان |
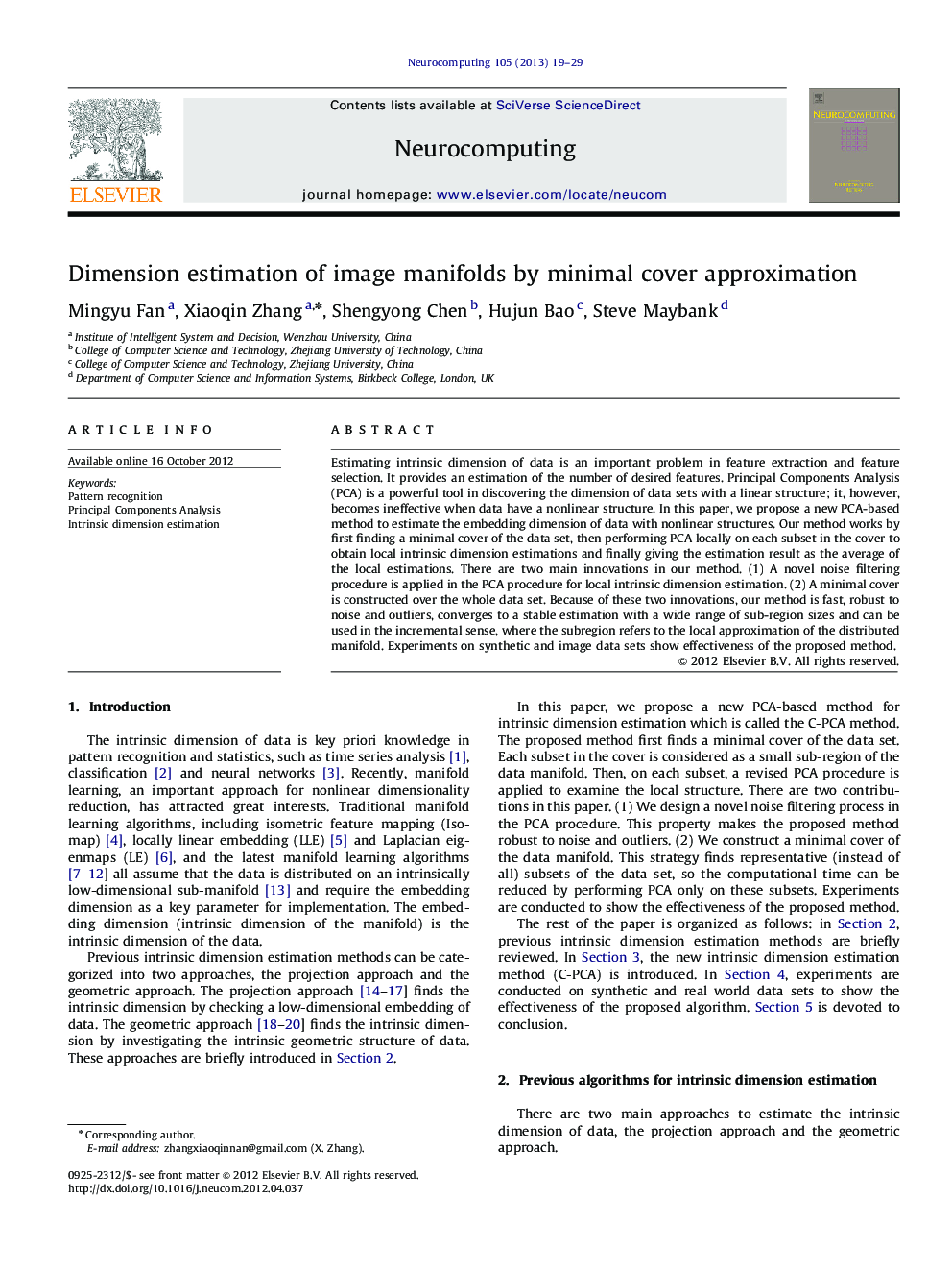
Estimating intrinsic dimension of data is an important problem in feature extraction and feature selection. It provides an estimation of the number of desired features. Principal Components Analysis (PCA) is a powerful tool in discovering the dimension of data sets with a linear structure; it, however, becomes ineffective when data have a nonlinear structure. In this paper, we propose a new PCA-based method to estimate the embedding dimension of data with nonlinear structures. Our method works by first finding a minimal cover of the data set, then performing PCA locally on each subset in the cover to obtain local intrinsic dimension estimations and finally giving the estimation result as the average of the local estimations. There are two main innovations in our method. (1) A novel noise filtering procedure is applied in the PCA procedure for local intrinsic dimension estimation. (2) A minimal cover is constructed over the whole data set. Because of these two innovations, our method is fast, robust to noise and outliers, converges to a stable estimation with a wide range of sub-region sizes and can be used in the incremental sense, where the subregion refers to the local approximation of the distributed manifold. Experiments on synthetic and image data sets show effectiveness of the proposed method.
Journal: Neurocomputing - Volume 105, 1 April 2013, Pages 19–29