کد مقاله | کد نشریه | سال انتشار | مقاله انگلیسی | نسخه تمام متن |
---|---|---|---|---|
407759 | 678168 | 2012 | 15 صفحه PDF | دانلود رایگان |
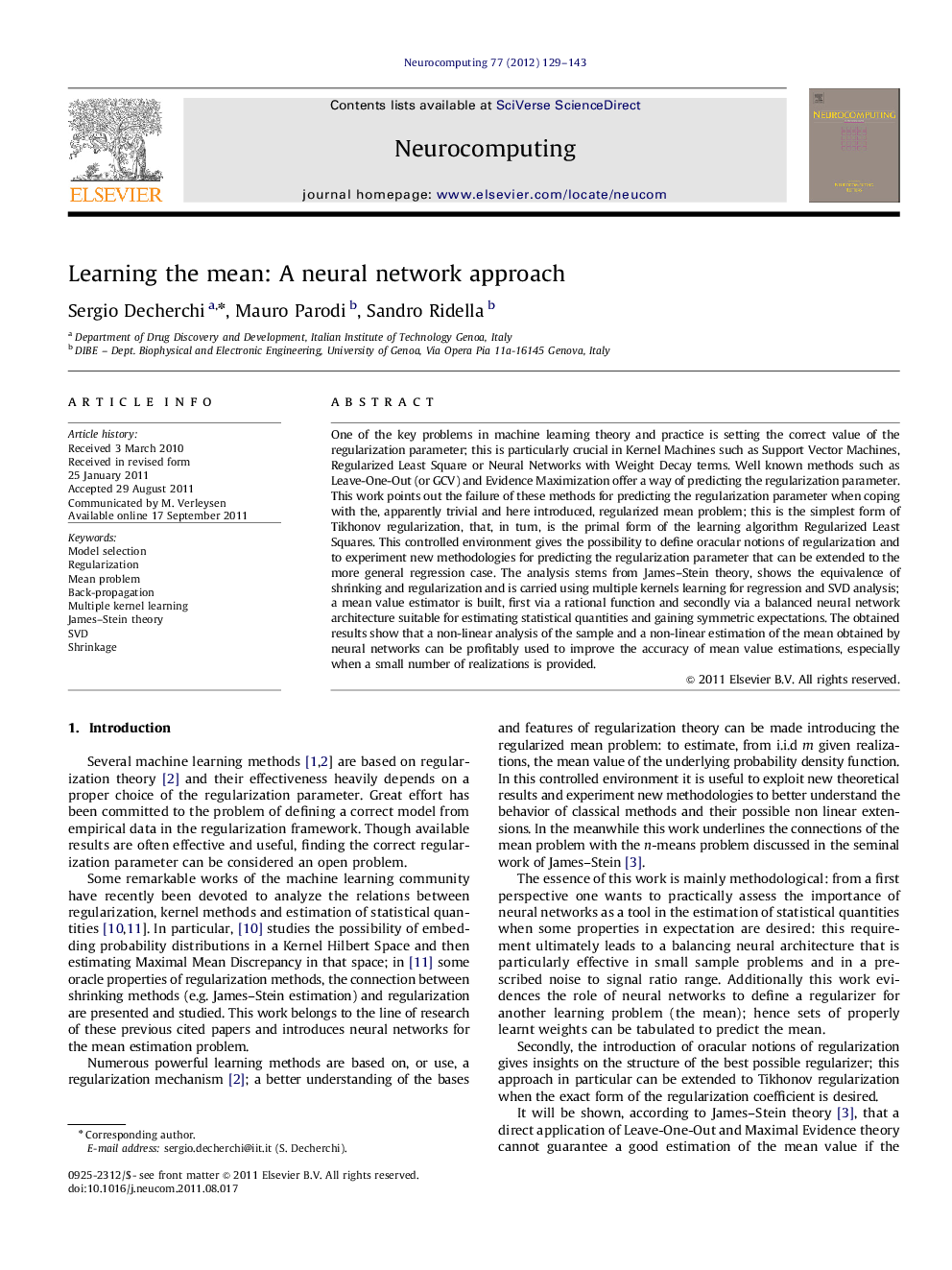
One of the key problems in machine learning theory and practice is setting the correct value of the regularization parameter; this is particularly crucial in Kernel Machines such as Support Vector Machines, Regularized Least Square or Neural Networks with Weight Decay terms. Well known methods such as Leave-One-Out (or GCV) and Evidence Maximization offer a way of predicting the regularization parameter. This work points out the failure of these methods for predicting the regularization parameter when coping with the, apparently trivial and here introduced, regularized mean problem; this is the simplest form of Tikhonov regularization, that, in turn, is the primal form of the learning algorithm Regularized Least Squares. This controlled environment gives the possibility to define oracular notions of regularization and to experiment new methodologies for predicting the regularization parameter that can be extended to the more general regression case. The analysis stems from James–Stein theory, shows the equivalence of shrinking and regularization and is carried using multiple kernels learning for regression and SVD analysis; a mean value estimator is built, first via a rational function and secondly via a balanced neural network architecture suitable for estimating statistical quantities and gaining symmetric expectations. The obtained results show that a non-linear analysis of the sample and a non-linear estimation of the mean obtained by neural networks can be profitably used to improve the accuracy of mean value estimations, especially when a small number of realizations is provided.
Journal: Neurocomputing - Volume 77, Issue 1, 1 February 2012, Pages 129–143