کد مقاله | کد نشریه | سال انتشار | مقاله انگلیسی | نسخه تمام متن |
---|---|---|---|---|
408010 | 678242 | 2011 | 12 صفحه PDF | دانلود رایگان |
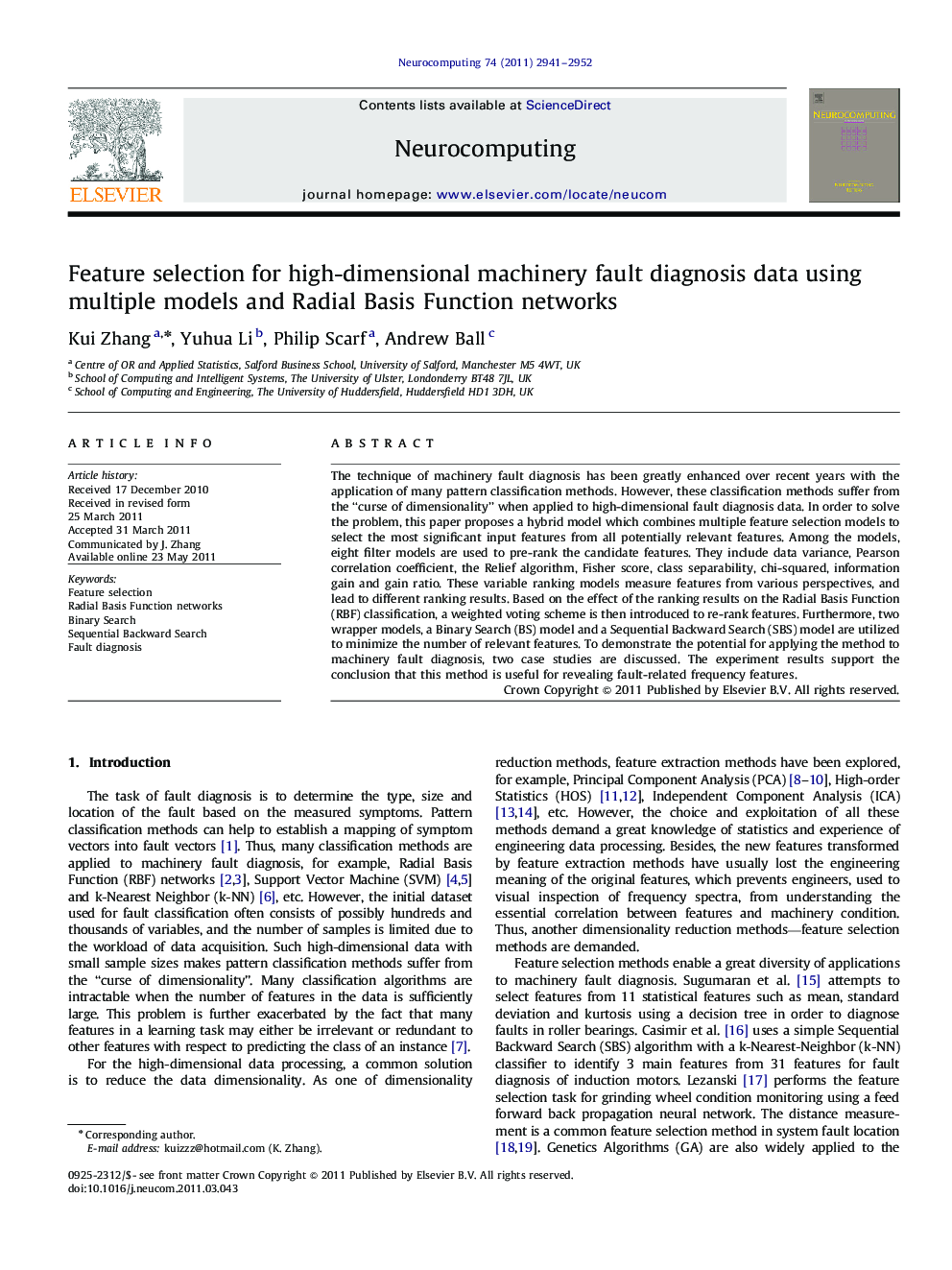
The technique of machinery fault diagnosis has been greatly enhanced over recent years with the application of many pattern classification methods. However, these classification methods suffer from the “curse of dimensionality” when applied to high-dimensional fault diagnosis data. In order to solve the problem, this paper proposes a hybrid model which combines multiple feature selection models to select the most significant input features from all potentially relevant features. Among the models, eight filter models are used to pre-rank the candidate features. They include data variance, Pearson correlation coefficient, the Relief algorithm, Fisher score, class separability, chi-squared, information gain and gain ratio. These variable ranking models measure features from various perspectives, and lead to different ranking results. Based on the effect of the ranking results on the Radial Basis Function (RBF) classification, a weighted voting scheme is then introduced to re-rank features. Furthermore, two wrapper models, a Binary Search (BS) model and a Sequential Backward Search (SBS) model are utilized to minimize the number of relevant features. To demonstrate the potential for applying the method to machinery fault diagnosis, two case studies are discussed. The experiment results support the conclusion that this method is useful for revealing fault-related frequency features.
► The classification methods suffer from the ‘curse of dimensionality’.
► The fault-related features can be identified by applying feature selection algorithms.
► Feature select can be implemented by combining multiple feature selection models.
► The selected frequency features have important engineering significance.
Journal: Neurocomputing - Volume 74, Issue 17, October 2011, Pages 2941–2952