کد مقاله | کد نشریه | سال انتشار | مقاله انگلیسی | نسخه تمام متن |
---|---|---|---|---|
409182 | 679058 | 2014 | 8 صفحه PDF | دانلود رایگان |
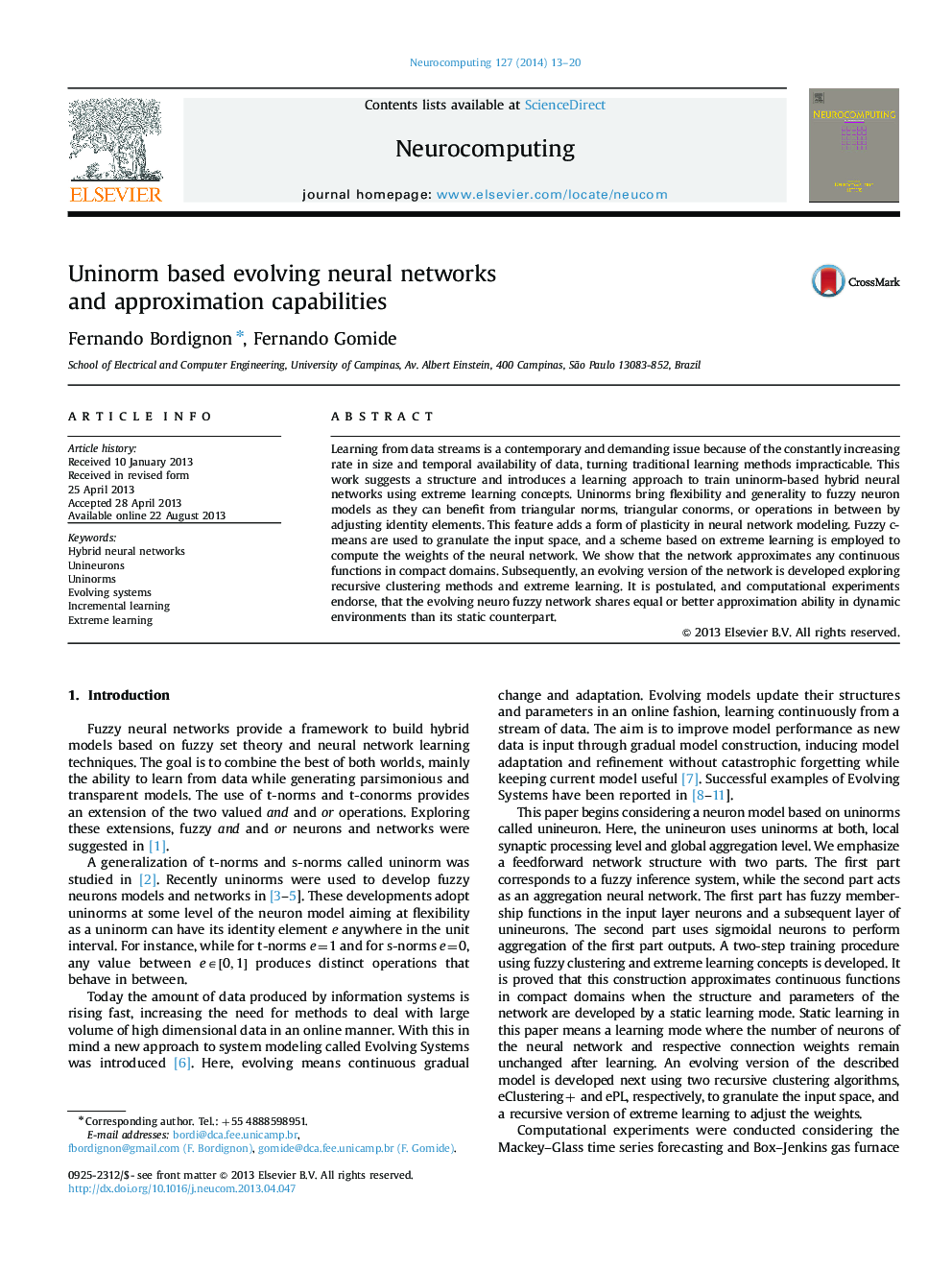
Learning from data streams is a contemporary and demanding issue because of the constantly increasing rate in size and temporal availability of data, turning traditional learning methods impracticable. This work suggests a structure and introduces a learning approach to train uninorm-based hybrid neural networks using extreme learning concepts. Uninorms bring flexibility and generality to fuzzy neuron models as they can benefit from triangular norms, triangular conorms, or operations in between by adjusting identity elements. This feature adds a form of plasticity in neural network modeling. Fuzzy c-means are used to granulate the input space, and a scheme based on extreme learning is employed to compute the weights of the neural network. We show that the network approximates any continuous functions in compact domains. Subsequently, an evolving version of the network is developed exploring recursive clustering methods and extreme learning. It is postulated, and computational experiments endorse, that the evolving neuro fuzzy network shares equal or better approximation ability in dynamic environments than its static counterpart.
Journal: Neurocomputing - Volume 127, 15 March 2014, Pages 13–20