کد مقاله | کد نشریه | سال انتشار | مقاله انگلیسی | نسخه تمام متن |
---|---|---|---|---|
409203 | 679058 | 2014 | 17 صفحه PDF | دانلود رایگان |
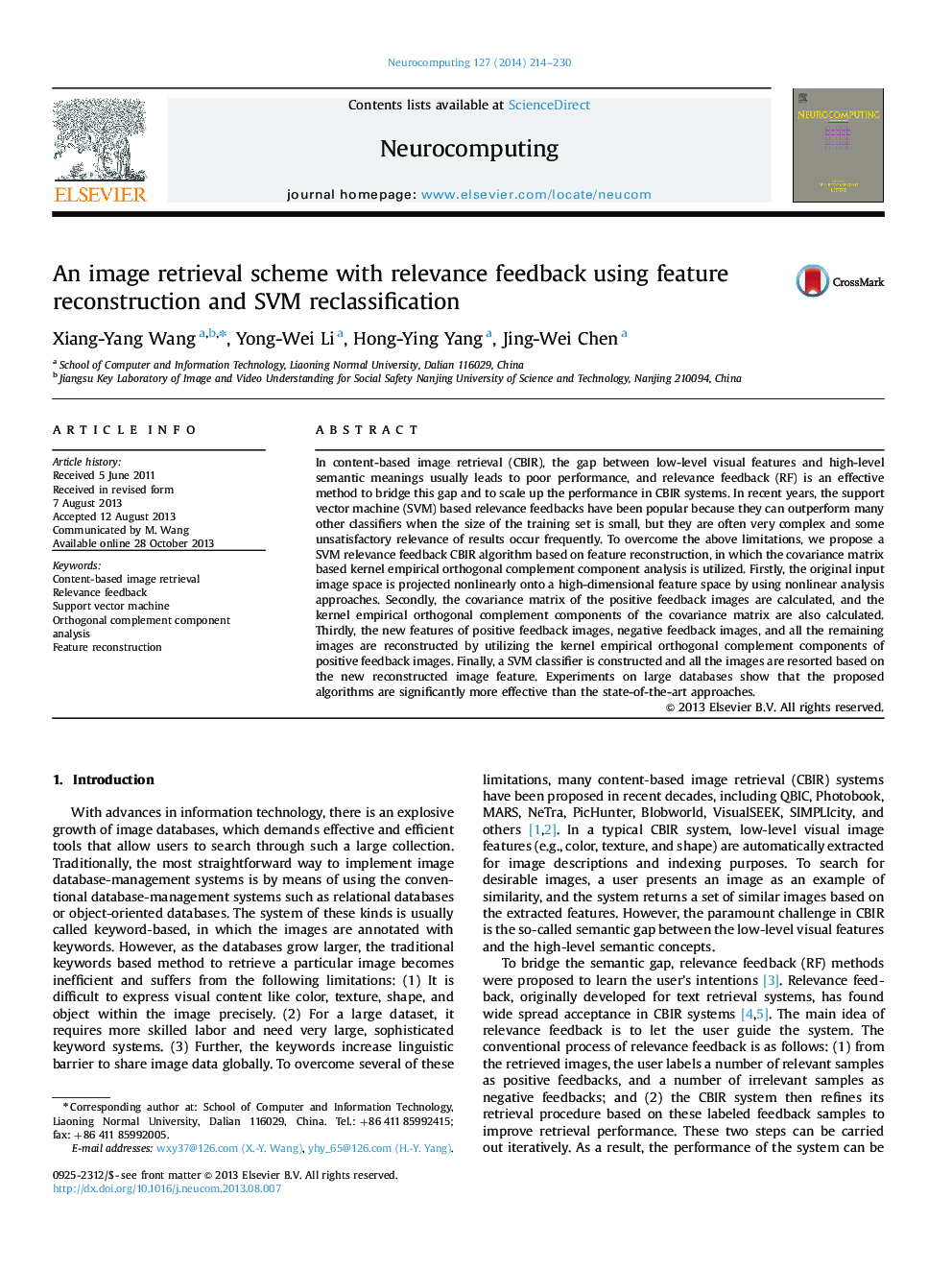
In content-based image retrieval (CBIR), the gap between low-level visual features and high-level semantic meanings usually leads to poor performance, and relevance feedback (RF) is an effective method to bridge this gap and to scale up the performance in CBIR systems. In recent years, the support vector machine (SVM) based relevance feedbacks have been popular because they can outperform many other classifiers when the size of the training set is small, but they are often very complex and some unsatisfactory relevance of results occur frequently. To overcome the above limitations, we propose a SVM relevance feedback CBIR algorithm based on feature reconstruction, in which the covariance matrix based kernel empirical orthogonal complement component analysis is utilized. Firstly, the original input image space is projected nonlinearly onto a high-dimensional feature space by using nonlinear analysis approaches. Secondly, the covariance matrix of the positive feedback images are calculated, and the kernel empirical orthogonal complement components of the covariance matrix are also calculated. Thirdly, the new features of positive feedback images, negative feedback images, and all the remaining images are reconstructed by utilizing the kernel empirical orthogonal complement components of positive feedback images. Finally, a SVM classifier is constructed and all the images are resorted based on the new reconstructed image feature. Experiments on large databases show that the proposed algorithms are significantly more effective than the state-of-the-art approaches.
Journal: Neurocomputing - Volume 127, 15 March 2014, Pages 214–230