کد مقاله | کد نشریه | سال انتشار | مقاله انگلیسی | نسخه تمام متن |
---|---|---|---|---|
409497 | 679074 | 2015 | 15 صفحه PDF | دانلود رایگان |
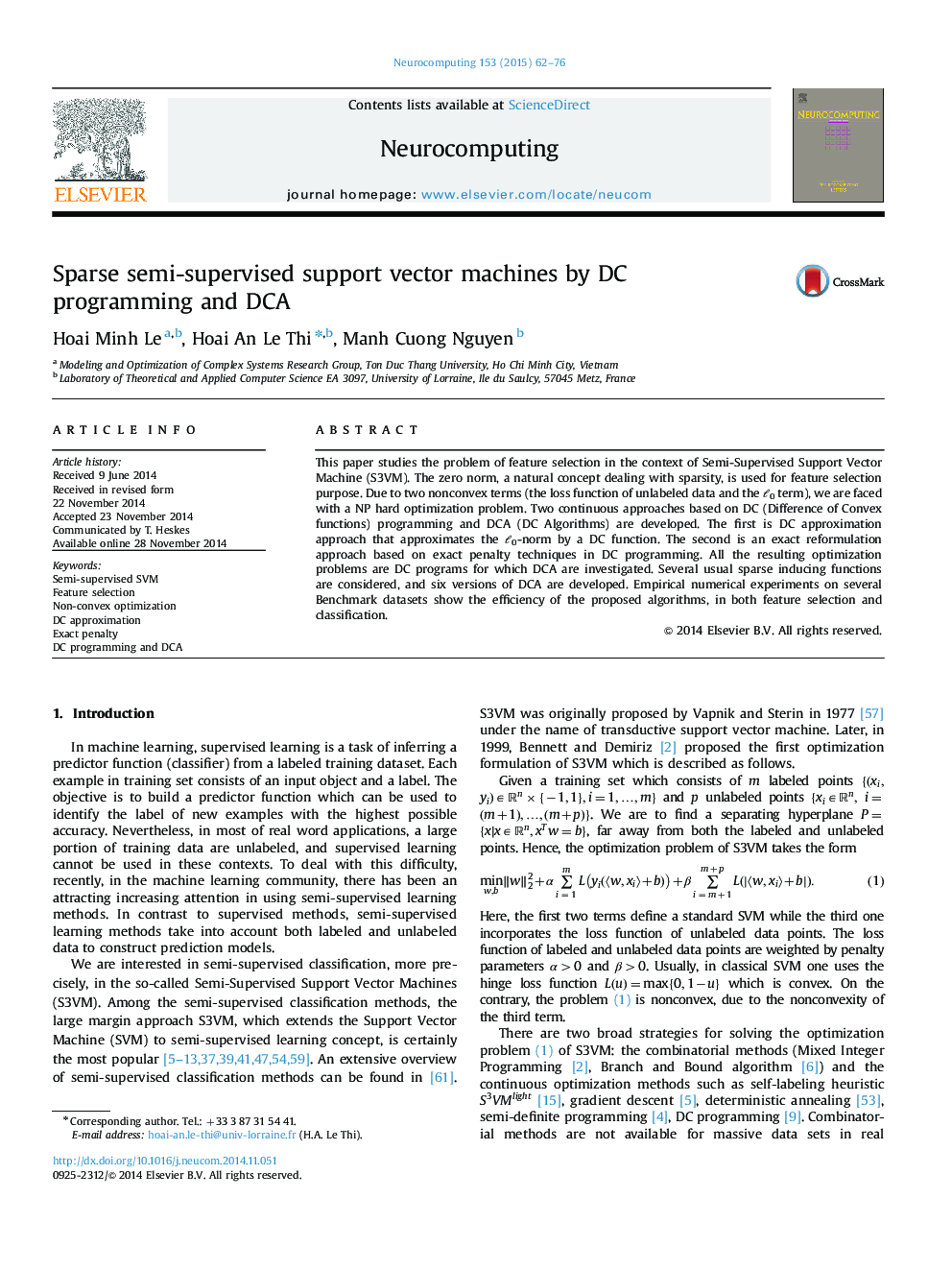
• Two DC (Difference of Convex) Algorithms based approaches are investigated for feature selection in S3VM using the zero norm.
• The first is DC approximation approach that approximates the zero norm by a DC function. Five approximations are considered.
• The second is an exact reformulation approach based on exact penalty techniques in DC programming.
• All the resulting problems are DC programs for which six versions of DCA are developed.
• A careful empirical experiment on several benchmark datasets are performed.
This paper studies the problem of feature selection in the context of Semi-Supervised Support Vector Machine (S3VM). The zero norm, a natural concept dealing with sparsity, is used for feature selection purpose. Due to two nonconvex terms (the loss function of unlabeled data and the ℓ0 term), we are faced with a NP hard optimization problem. Two continuous approaches based on DC (Difference of Convex functions) programming and DCA (DC Algorithms) are developed. The first is DC approximation approach that approximates the ℓ0-norm by a DC function. The second is an exact reformulation approach based on exact penalty techniques in DC programming. All the resulting optimization problems are DC programs for which DCA are investigated. Several usual sparse inducing functions are considered, and six versions of DCA are developed. Empirical numerical experiments on several Benchmark datasets show the efficiency of the proposed algorithms, in both feature selection and classification.
Journal: Neurocomputing - Volume 153, 4 April 2015, Pages 62–76