کد مقاله | کد نشریه | سال انتشار | مقاله انگلیسی | نسخه تمام متن |
---|---|---|---|---|
409802 | 679090 | 2015 | 11 صفحه PDF | دانلود رایگان |
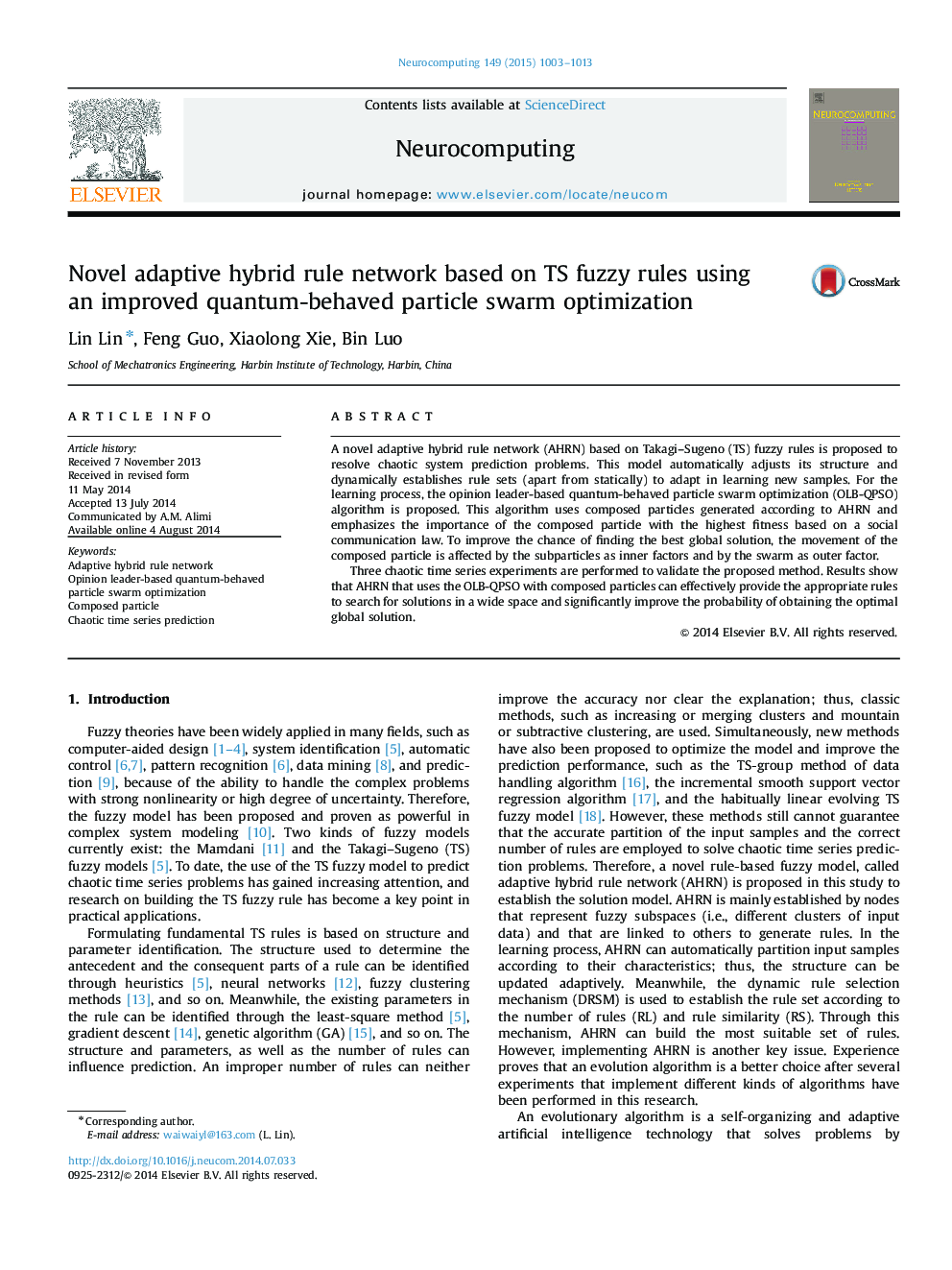
• A novel adaptive model is proposed to deal with the chaotic time series prediction.
• Dynamic rule selection mechanism is proposed for the model to establish the rule set.
• Opinion leader-based QPSO (OLB-QPSO) algorithm is proposed to learn this model.
• The composed particle with a multiple structure is used in OLB-QPSO.
A novel adaptive hybrid rule network (AHRN) based on Takagi–Sugeno (TS) fuzzy rules is proposed to resolve chaotic system prediction problems. This model automatically adjusts its structure and dynamically establishes rule sets (apart from statically) to adapt in learning new samples. For the learning process, the opinion leader-based quantum-behaved particle swarm optimization (OLB-QPSO) algorithm is proposed. This algorithm uses composed particles generated according to AHRN and emphasizes the importance of the composed particle with the highest fitness based on a social communication law. To improve the chance of finding the best global solution, the movement of the composed particle is affected by the subparticles as inner factors and by the swarm as outer factor.Three chaotic time series experiments are performed to validate the proposed method. Results show that AHRN that uses the OLB-QPSO with composed particles can effectively provide the appropriate rules to search for solutions in a wide space and significantly improve the probability of obtaining the optimal global solution.
Journal: Neurocomputing - Volume 149, Part B, 3 February 2015, Pages 1003–1013